Leo M. Lamber: Good afternoon, and welcome to the Areté Medallion ceremony. Today we have the honor of bestowing the inaugural Imagining the Internet Areté Medallion to Dr. Vint Cerf, Vice President and Chief Internet Evangelist for Google, and a modern pioneer who changed the way we live our lives. As an architect and codesigner of the Internet, Dr. Cerf imagined a world that didn’t exist and developed technologies that undergird our daily lives, the global knowledge economy, and endless possibilities for creating a healthier, more educated, and more connected world.
The Areté Medallion was established to honor innovators, change agents, thought leaders who have dedicated their lives as public servants and have initiated and sustained work that benefits the greater good of humanity through contributions that enhance the global future.
“Areté” is Greek for moral virtue and striving for excellence in service to humanity. In ancient Greek, the term areté was used to describe people stretching to reach their fullest potential in life, embodying goodness and excellence. Innovation and entrepreneurship are essential 21st century skills and critical goals of Elon’s student-centered learning environment.
It is fitting that Dr. Cerf is the very first recipient of the are Areté Medallion, based on his contributions to global policy development and the continued spread of the Internet. He’s been recognized with the US Medal of Technology, the Alan Turing Award, known as the Nobel Prize of computer science, and the presidential Medal of Freedom, and has served as founding President of the Internet Society, member of the National Science Board, and on the faculty of Stanford University. His exemplary service to the development of our digital lives stands as a tremendous example for Elon’s students, who are developing themselves as change-makers, building new initiatives and partnerships, and making their communities, from Burlington to Beijing, better through leadership and service.
I’d like to call on Professor of Communications and Director of the Imagining the Internet Center Janna Anderson to introduce Dr. Cerf. A widely-recognized authority on our digital future, since the Fall of 2000 Professor Anderson has led Elon’s internationally-recognized efforts to document the development of digital technology. Nearly four hundred Elon students, faculty, staff, and alumni have participated in research through the Center, and Janna’s students have provided multimedia coverage at conferences in Athens, Hong Kong, Rio de Janeiro, among many other places. Thank you, Professor Anderson. Your prolific scholarship, outstanding teaching, and passionate mentoring of hundreds of Elon students have provided all of us with a clear vision for the future. Please welcome professor Janna Anderson.
Janna Anderson: Thank you, and welcome to everyone here for family weekend. Who’s here for family weekend? Alright! Beat Villanova. Go! Who’s going shopping today? Anybody going shopping, yeah? Taking the kids out to dinner? Yeah?
We’re really excited for the privilege of having Vint Cerf here today. He was telling us he was in San Francisco just yesterday. He’s going to be in Brasilia tomorrow. And his travel schedule would kill a twenty year-old. So, he needs an award just for all the travel he does, really. He’s an amazing guy. I could talk forever about Vint, so I’m going to read my script so that I don’t stay here forever and give him a chance to talk.
It’s an honor for us to award Dr. Vinton Gray Cerf the inaugural Areté Medallion, recognizing all of the work he’s done over the past few decades, mostly being excellent in public service. The most important thing is working for global good. In the 1970s and 80s, Vint Cerf and a brilliant group of engineers, brought to life a communications network that has become many orders of magnitude more powerful in the years since than they had ever imagined it would become.
Bob Kahn and others developed the TCP/IP protocol suite and the early network, and in the decades that followed, millions of applications emerged. Billions of people, more than half the world, are carrying a connection to global intelligence in their pockets today. It wasn’t just they that did it, but it was the information invention, the communications network that they created, that inspired everyone to get together and make these things happen. Everyone whose life has been enriched by being connected to the Internet please wave your phone, your tablet, your your PC, in welcome to one of the great engineers of all time, right here.
In a few minutes, we’re going to be treated to a wonderful talk by this amazing engineer and public intellectual who was one of the first to imagine IP on everything. For nearly five decades, he has lived a life of nonstop public service. He is now working to make it possible for every item on earth to be connected in what is called the Internet of Things. And he is a classic t‑shirt to prove it. It reads “IP on everything.” He had that on his Facebook site— Is it still your Facebook homepage profile photo? Engineers have a great sense of humor. You’ve got to hang out with them more often if you don’t already, right?
He knew from the start that a network is only as good as the people who build it and use it. And so it wasn’t just the engineering feat that is amazing in what this man has done in his lifetime. He has involved himself in the leadership position in every organization that has been founded to try to figure out how we’re going to make this thing work, not only technically but socially, to serve humanity as well as it can.
Many who know him believe that Vint may also be the first human to ever have been cloned. As I mentioned before he’s everywhere all the time. He has been awarded dozens of honorary degrees. He’s been named a fellow of or elected president of just about every organization he’s ever been a part of. And he’s working now to solve global problems such as gender inequality, the digital divide, and the changing nature of jobs in an era of artificial intelligence. And as you can see—well, you will see soon, he’s going to be talking not only about the Internet of Things but about artificial intelligence as we move into the future today.
So he’s been working to solve these problems, but every time anybody asks him to do almost anything, he’ll just…he’ll do that thing. He’s one of those people who gets things done, and he gets them done right away. If you ask him a favor, he’ll do it. I’ve asked him several times over the years, I’ve been so, “Oh, I don’t know if I should ask him this…” And he just moves forward and he does it. He checks it off the list. He participates. He’s out there. If you ask Vint, he’s going to be there for you, and that’s one of the reasons why he’s so popular not only in the engineering community but among all the people who are online and doing things.
So it’s not possible to list every good deed he’s done. It hasn’t probably been documented. Maybe if you went through his email account you could find all of his yeses and and see all the things he’s done. But it’s why he’s on the road 80% of the time. It’s amazing, really.
So, he and Tim Berners-Lee, I think, are the Paul McCartney and Mick Jagger of communications for us. The rock, okay! They rock, man. So you have to be excited about this because this is a once-in-a-lifetime opportunity to spend time with him. Where we are today is greatly due to their commitment to do good and tirelessly work for a better future. They didn’t just build that thing and then step back and say, “Dude. Yeah. I really did a great job there.” And they didn’t monetize that thing. They didn’t try to take advantage of it as a business opportunity. They thought it up, invented it to connect people, to information, to each other, to everything. It’s amazing. So learn your lesson. Follow their lead. Do good for everyone.
The ancient Greeks used the term areté to describe a person who has reached the greatest effectiveness with dignity, character, and distinction. Vint Cerf embodies areté. The people of Elon University and the Imagining the Internet Center are pleased to honor him with Elon’s inaugural Areté Medallion.
Vint Cerf: Thank you very much. You know, I have received a number of awards, but what’s important about this one is this is the first time it’s been given, and that’s a big honor, to be sort of the person who receives the first instance of it. Of course maybe we’ll find a typo in here, too. You never know. That has happened at least once before.
I’m very very pleased to be on this campus. It’s a beautiful campus. I got a chance to walk around this morning, see the new construction, which is a little bit behind schedule but nonetheless very exciting. Especially the new facilities experimenting with teaching tools. For example the gigantic touchscreen and things like that.
One of the things that really caught my attention is that in some of the classrooms that have been designed with this large 96″ high-res touchscreen display is that the students who are in there will also have the ability to push things onto the screen from their laptops or from their mobiles. And it dawned on me that in most cases, like this one, I have material to show you but I don’t get this feedback that we might get if we were collaborating on whatever is up there on the screen. And this idea of education becoming a collaborative experience I think may be very important. And so you’ll be exploring that in this new school. And it will be very interesting to see what comes out of that. I think Lee Rainie will certainly want to assess what happens as a result, if possible. Because we may learn a lot from that. So I’m really excited to await the opening of the new school and the facilities that it has there.
But what I’d like to do this afternoon is talk a little bit about artificial intelligence and the Internet. I want to warn you of a couple of things. First one is I don’t consider myself an expert in artificial intelligence. We have some pretty remarkable people at Google who are, and I don’t want you to blame them for my kind of lightweight understanding of what’s going on.
But I do have a few examples of things that are starting to happen. And you can see that AI normally stands for artificial intelligence, but I’ve often concluded it stands for “artificial idiot.” And the reason is very simple. It turns out that these systems are good, but they’re good in kind of narrow ways. And we have to remember that so we don’t mistakenly imbue some of these artificial intelligences and chat bots and the like with a breadth of knowledge that they don’t actually have, and also with social intelligence that they don’t have.
If any of you have read Sherry Turkle’s book called Alone Together, the first part of the book is about people’s interaction with humaniform robots, that is robots that have a somewhat human appearance. And often, people will imbue the robot with a kind of social intelligence that it doesn’t have. If the robot appears to ignore that person, they get insulted or depressed. And of course the robot doesn’t have a clue about what’s going on. And so we tend to project onto these humaniform things more depth than is really going on. So we should be very careful about that.
There is one other phenomenon. The closer you get to lifelike, the more creepy it gets. And seriously, if you’ve seen some of the robots that are being made in Japan, that are being used in hotel lobbies and things like that and department stores, they look like they’re kinda dead bodies that are animated, you know. Sort of zombies. And so it’s a very funny phenomenon that if it’s too lifelike it gets really disturbing. And so it could very well be that we need something which is not terribly precise and not high-fidelity. Those of you who’ve ever seen a movie with Robin Williams called The Bicentennial Man will appreciate the transformation as he goes from a very stylized-looking robot to one which is very nearly human. And of course eventually he ends up being essentially human. And that was his objective. It’s sort of a Pinocchio story.
Okay, so let me give you an example of how artificial intelligence doesn’t always work. This is an example of language translation. I was in Germany at the time, and I was pulling up a weather report. And it was being translated by the the Google translation system. And you will see in the lower left-hand side, probability of rain was 61%. Probably of snow was zero. Probability of ice cream was 0%.
And you know, I thought well what was that? And it turns out that they meant hail. And the German word for hail is “eis.” But that’s also the word for ice cream. And absent the context that was necessary, the translation system translated this as “ice cream.” And so I showed this slide to my German friends, thinking you know, “Can you tell me more about this ice cream storm that you have here that I’ve never experienced?” So that’s just a tiny little example. But it shows how easy it is for something which is trying hard to understand natural language to get it wrong.
On the whole I will say that the Google’s translation systems are actually pretty good. And there have been occasions where I have gone to a page in Germany or France or Spain where the web pages are automatically translated, and they happened so fast that a couple of times the translation has been so good and so quick that I didn’t notice that it wasn’t originally in English, that it was in some other language. So I’m very proud of what Google has been able to do. But as I say, every once awhile goes clunk.
So here’s another thing which we are very proud of at Google and that’s speech recognition. And I want to distinguish speech understanding from speech recognition. The ability to figure out that words were spoken and what those words were, and how to spell them and turn them into text is what speech recognition is about.
We have had extraordinarily good results with speech synthesis, where we’ve taken text and turned it back into speech. And of course the display of text. That can be quite helpful for example for a person who is hearing impaired and needs captioning, for example, to see a video or to carry on a conversation.
Google Maps is now commonly accessed by voice request. In fact when Gene Gabbard, my good friend who’s here—we go back many years at MCI—was coming here, we use them my little little Google Maps program, and I spoke the address that we were going to. And it’s quite shocking how well the system works. One thing that helps it is that the vocabulary that it’s expecting is the names of streets and towns and cities and so on; countries. And that narrows to some extent the recognition problem because you’re drawing from a vocabulary of a certain type. And that helps improve the quality of the recognition.
We also are able to tie things together. You might want to experiment with this. If you were to say something like, “Where is the Museum of Modern Art in New York City?” and it answers with an address or something like that. And if you then said, “How far away is it?” You haven’t specifically mentioned anything; the reference to “it” is the museum. It remembers things like that. So we’re starting to tie conversation together. And one of the big objectives at Google has been to achieve a kind of interactive interface, for example with Google Search, where you’re not simply asking for search terms or just a certain sentence or a statement or question, but rather that there is an interactive engagement. And the interaction is intended to help you figure out whether the search engine understands what you’re looking for.
I noticed something very interesting a few months ago. Maybe you have as well. I used to just type search terms into the Google search engine. Now I type a complete question, if that’s what I’m looking for an answer for. And I found that it was doing better in responding when I asked a grammatically well-formed question. And I asked the natural language guys you know, “What’s going on?” And they said, “Well, we had been doing a lot of work with just the search terms, but now we’re paying a lot more attention to sentence structure, to semantics.”
There’s something we have called the Knowledge Graph. It has about a billion nodes in it. And it associates concepts and nouns with each other. So if two concepts are related, the branch between the two nodes says how they’re related. We use that a lot, especially in our search algorithms. So when we read the question that you’ve written, we also ask our own knowledge graph how do the turns in that question relate to things we know about in this knowledge graph, and it expands the search to a broader space and therefore we hope gives you more complete coverage. If you said, “Where is Lindau,” for example, and then you said, “Are there any restaurants there?” that’s the ellipsis kind of thing, and we’re hoping to refine that even further.
Here’s another example. This is the the German translation of what the Semantic Web is all about. And the translation of that looks like this. And at the time that I was putting this example together, the translation happened automatically, and it happened so fast that I actually didn’t notice that it started out in German. And here you see the translation in English. So we’re getting better and better not only at doing the translation but producing sentence structures that look natural.
So now there’s this question about the Deep Web. And here, in a sense this is kind of like dark matter in the universe. We know it exists but we can’t see it. If it isn’t in HTML, the standard web search engine can’t see it. These are for example databases that are full of information. And in the case where there isn’t any metadata describing what’s in the database, then we might not encounter it during a search. We certainly can’t index it because usually the way these systems are accessible is you keep asking questions of the database and that derives its content to you. But we can’t send the knowledge robot, or the little spider, around the Internet asking bazillions of questions of all the databases in order to infer what’s in it.
So one of the things that we would need is a way of getting these databases to describe themselves in some sense, or the creators to describe them in a way that gives us semantic knowledge of what’s in the database. And I want to distinguish Deep Web from dark web. Dark Web is a whole other thing. That’s where all kinds of illegal content and malware is found. But Deep Web is a perfectly legitimate notion. It’s just that we have trouble finding things in it. So I have this general feeling that we have a long way to go to make our artificial intelligence mechanisms capable of seeing what’s in the World Wide Web or what’s in other things that are not necessarily web-based so as to help us find and use that content.
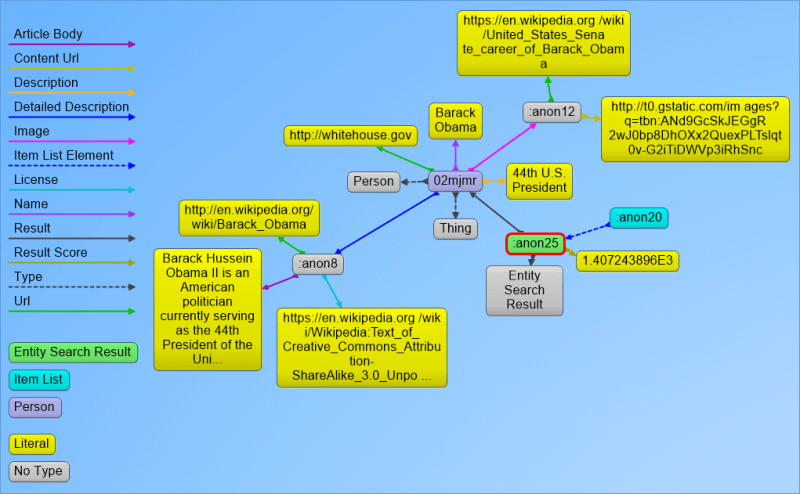
Image: Search Engine Land, “A Layman’s Visual Guide To Google’s Knowledge Graph Search API”
Here’s our Knowledge Graph, just as an example. I’m not sure whether that’s terribly readable to you. I apologize for that, and I think trying to blow it up probably won’t work. But what what you’re seeing basically is descriptors of concepts that’re being associated with each other. And those descriptors are what we use in order to analyze what we’re finding in the net. Now, this is not just a question of using it for translation, for example, or using it for search. We use it to infer the content of conversation. For example if you ask what is the status of a particular flight on an airline, our knowledge graph knows that airlines use airplanes; they know that you go from one place to another; there’s a whole lot of knowledge that’s associated with that. So if you start asking questions like, “What kind of airplane am I flying?” on that flight, the system understands that question and can try to respond if it has the data that’s available.
Ray Kurzweil is now working at Google and has been there for several years. I don’t know if you’ve read any of his books. One of them is called The Singularity is Near. And the Singularity from his point of view is the point at which computers are smarter than people. And he’s hoping that by 2029 that computers will be so smart that he’ll be able to upload himself into a computer and then go explore the galaxy. That’s not why we hired him. We hired him to work on the Knowledge Graph that would help us with our AI applications. But he’s quite excited about the exponential ability to gain knowledge and to infer things from it.
Some people are worried, for example, that once a computer knows how to do something, it will learn how to do it better than any human can. And so for any particular thing humans can do, if the computer ever figures out how to do it, then we’re doomed. Now I think that’s too pessimistic. I have a much more optimistic view of a lot of this stuff, which is that these machines are there to help augment our thinking ability.
There’s a man you should know if you don’t. His name is Douglas Engelbart. Doug used to run the Augmentation of Human Intellect Project at SRI international in Menlo Park, California. This was many years ago in the mid to late 60s. And his view was that computers were there to help us think better. And he liked the idea of collaborating among people and facilitating the collaboration through the use of the computer. He invented a system which for all practical purposes was a World Wide Web in a box. It was called the oN-Line System (NLS). It had, in the system, hyperlinking. So he invented the notion of connecting documents to each other by clicking on— Clicking? He invented the mouse so you could point to something on the screen and then click to say “pay attention to that place that I’m pointing to.”
He had a very elaborate editor which allowed people to create documents, and the whole idea there was that knowledge workers would create content by creating documents and could reference each other. People could collaborate. We were using a projector, a much much older one than what you have here, that was called a GE Light Valve that cost fifty thousand dollars. It was half the size of a refrigerator. But we were using it to project somebody typing on a keyboard, building a document with this oN-Line System. And I remember John Postel, who used to be the Internet Assigned Numbers Authority, was taking notes. And while I was talking, he was typing away, and his words were showing up on the screen. And I stopped to see what I was going to say next. And then I realized that nothing would happen if I didn’t say anything. So, it was an odd feedback loop.
This whole point, though, is that that Ray is very very interested in trying to codify semantic information. One of the questions I asked him, which I don’t have an answer to yet, is whether this knowledge graph and the mechanisms that go with it, including inference, are sufficiently rich that we could get the computers running these text ingestion systems to just read lots and lots and lots of books and then essentially form knowledge and knowledge structures by reading. And you know, considering the vast range of material that’s in written form, you could imagine a computer essentially learning from that.
And I don’t know how far we can get with this, but we have discovered that machine learning is effective for certain classes of activity. Classification, for example. You know, the ability to distinguish cats and dogs and other things in images, or distinguishing a cancerous cell from a non-cancerous one. A lot of those kinds of machine learning tasks are actually quite easily done, or readily done these days. More complex kinds of recognition might turn out to be a lot harder.
But at some point I kept thinking well maybe the computer can start learning itself. There is a guy whose name is Doug Lenat. He’s at University of Texas Austin. He has been working probably for the last twenty years, maybe twenty-five years, on something he calls “Cyc,” which stood for encyclopedia. What he was trying to do there is to get the computer to learn by reading. And his idea was that if you took a paragraph of text and you then manually incorporated the information that the computer would need to recognize and understand the text, that eventually he’d build up a sufficient corpus that the machine wouldn’t need help from you anymore, it would just learn from reading.
So an example of a sentence was that, “Wellington learned that Napoleon had died, and he was saddened.” Well, in order to understand that sentence in its entirety you have to understand that Wellington and Napoleon were human beings; humans are born, they live for a while and they die. You have to know that Wellington and Napoleon were great adversaries. You might have to know that Wellington defeated Napoleon. And you also have to understand that great adversaries sometimes form a certain degree of admiration for each other despite their opposition. And all of that would go into understanding the depth of that one sentence, so you can imagine having to encode all that other information so that the computer would have that to draw on to fully understand what’s going on. So in some sense, that’s a little bit about what the Knowledge Graph is trying to do.
Well, another thing that AI has been regularly associated with is games. I guess I should describe for you how AI was treated at Stanford University in the 1960s when I was an undergraduate there. Basically if it didn’t work, it was artificial intelligence. And if it did work it was engineering. So every time we figured out how to get some AI thing to work, when it finally worked it wasn’t AI anymore. Mostly because AI at the time was kind of filled with heuristics that sometimes worked and sometimes didn’t, whereas engineering is supposed to get it right.
Well, I’m sure many of you are very familiar with these games that we’ve gotten computers to play. IBM Deep Blue played Garry Kasparov. And the interesting thing, he was beaten several times by by Deep Blue, and it was considered at the time—this is like 1997—a huge accomplishment, a milestone in artificial intelligence.
One very amusing incident, though, happened where the computer made a move that Kasparov could not understand. I mean, it made no sense whatsoever. And he he was clearly concerned about it because he thought for quite a long time and he had to play the endgame much faster than otherwise, and in the end it turned out it was a bug. It was just a mistake. The computer didn’t know what it was doing. But Kasparov assumed that it did, and lost the game as a result.
AlPhaGo, on the other hand, was a system that was built by our DeepMind company in London. They were training our special computing systems for this sort of neural network to play Go. And they played literally tens of millions of games. Sometimes they had multiple computers playing against each other. Sometimes they played against Lee Sedol, who was the expert that it defeated four times out of five.
I’m not a Go player and so I can’t express very well what happened in the first game, except I am told that at move 37, there was consternation among the knowledgeable Go players because the machine did something that didn’t make any sense to them at all. And in this case, it was not a bug. It turned out the machine had found a particular tactic which much later in the game turned out to allow the machine to capture a significant portion of the board.
So that was four games out of five that Lee Sedol lost. I have to give a lot of credit to him for being willing to take this risk. We basically told him that we’d give him a million dollars if he won three out of the five games, and he lost four out of the five. I don’t know whether they gave him the million dollars anyway, frankly. We should have, I think, if we didn’t.
But I want to emphasize how limited these kinds of capabilities are. We should not overstate the importance of this in the broader sense of intelligence as you and I might think of it. Checkers, for example, and tic-tac-toe were fairly easy targets way back in the 1960s, as very simple, straightforward games to play. The training of these things involves playing the same games over and over and over again and feeding back to the machine learning algorithm whether or not they won or lost. And so this is not the same kind of mentation that you do when you’re playing chess or Go. It’s adjustment of a bunch of parameters in a neural network.
And what is interesting about this is that we have the DeepMind systems play a whole bunch of different kinds of board games, and it learned to play the games without being told what all the rules were. They only had simple feedback like “you lost the game;” that’s one possibility. Or “this movie is illegal.” And after a while, the learning algorithm adjusted a lot of the parameters in the neural network until it could learn to play the game successfully. So there are lots of other games like the Atari video games and Pong and Breakout; these are all very simple kinds of games. But the computer learned how to play them simply by playing them and being given very very simple feedback.
Now, some of you will remember IBM Watson’s play on Jeopardy. This is as you all know a question and answer system. There is a programming system called Hadoop (and maybe some of the computer science folks here are familiar with that). It’s an implementation of the MapReduce algorithm. That’s what we use at Google to do a fast search of the index that Google generates of the World Wide Web. In principle, what happens is that you may replicate data across a large number of processors, and when you ask a question like, “Which web pages have these words on them?” that question goes to tens of thousands of machines, each of which has a portion of the index at its disposal. All the machines that find web pages with those words on it raise their hands. That’s the mapping part. Then you sweep all that stuff together in order to see what the response is to the query.
Then you have the other problem. You got ten million responses, you have to figure out what’s the right order in which to present them to the users. And that uses another algorithm, the first one of which was called PageRank. This is Larry Page’s idea where he just took all the web page responses to a query and said, “Which pages had more pointers going to it?” (references), and rank ordered them by reference. That’s the PageRank. And of course that’s turned out to be too crude now, and many people know about that, so they try to game the system, so now we have about two hundred and fifty signals that go into the ordering of the response that comes back. But again, the basic principle is to spread the information out in parallel, look for “hits,” and then bring it back together and then do this sorting.
Another thing which Watson did was of course to analyze the query that came to it and then generate hypotheses for what question was it that this descriptor represented an answer to. It was looking for all kinds of supporting evidence and scoring of its various possible hypotheses and answers, and then it had to synthesize an answer. Not only was it applicable to Jeopardy, which is a particular game, but it also is now being used for health diagnostics and analytics. And you can seen the sort of tool here, and the possibilities across quite a wide range of different applications where we’re accumulating substantial amounts of data about which we can reason.
At Google, we believe that special hardware is in fact worth investing in. And so in the case of our DeepMind company, we’ve developed a TensorFlow chipset. That system is physically not available to the public, except we put these gadgets into our data centers. And we made an API available to the public. So if people are curious and interested in writing AI programs, TensorFlow is open to you to try things out. And we encouraged that. We’ve taught classes in it. You’ll find online support for this.
The physical Tensor Processing Unit is not available. I asked why not. Why not share those with other people? And they said the API is there to create a stable layer of interaction with this device. But the reason they don’t want the hardware to get out is not that that’s a big secret but that they plan to evolve it. And so the whole idea was to take advantage of what people do with the TensorFlow programming system and then feed that back into the tensor chip design until we can make it perform more effectively.
IBM has a similar kind of neural network that is called True North. And these are all emulating the way our brains sort of work, right. So we have neurons with lots and lots of dendrites. The neurons touch many many other neurons. The dendritic connections are either excitatory or inhibitory. And the feedback loop basically is based on experience. So if you do something and the feedback is “that didn’t work,” then the excitatory and inhibitory connections’ parameters get adjusted in the chipset. And they get adjusted in our brains by some electrochemical process and modifications of the synapses.
So what’s very peculiar about these kinds of chipsets is that after all the training gets done and a set of parameters have been set in consequence of all the interaction, we’re not sure why it works. Just like we’re not quite sure why our brains work. And so for example, if you could open up one of these neural chips and look at all the parametric settings on all the little simulated neurons and ask the programmer, “If I change this value from .01 to .05, what will happen?” And the answer is, “Beats the hell out of me.” And so we actually don’t quite understand in a deep sense what what’s going on other than this interesting balancing of parameters as a consequence of feedback of learning.
It’s a little unnerving to think that we’re building machines that we don’t understand. On the other hand, it’s fair to say that the network, the Internet, is at a scale now where we don’t fully understand it, either. Not only in the technical sense like what’s it going to do or how is it going to behave, but also in the social sense, how is it going to impact our society? And that question of course goes double, I think, for artificial intelligence.
So if we look at the neural networks that are available now, most of the experiences with them are trying things out to see for which applications they actually work well. So for image recognition and classification they actually are quite good. We can tell the difference between dogs and cats. We can sometimes look at a scene and separate objects in it. That’s really important for robotics because you want the robot to look at a scene and notice that there are different things like there’s a glass here, and there’s a screen here and, the two are not the same thing; it’s not a two-dimensional thing. Sometimes you can figure that out because if you have multiple views of something and you can see that one thing moves, and I can see around the screen, and see that there’s another object there. But this image recognition is very important to a lot of applications, including robotics.
And here’s an interesting experiment that I’m now free to tell you about. It was not publicized for a while. We took our TensorFlow Processing Unit and we started training it against the cooling system of one of our data centers. Now, if you think about it, I don’t know if you seen pictures of data centers. They’re big, big things. I mean, I’m a programmer type and for me a computer is my laptop. It’s a little hard to believe when you walk into a data center you need this much iron in order to operate at the scale that Google does. So for me it’s a little weird to see these eight-inch pipes of cold water flowing, fans blowing, and you know, megawatts, tens, hundreds of megawatts of power going in and out.
So, you walk into this thing and it’s clear that you’re generating a lot heat. I had even proposed at one point that because there was so much heat being generated and heat rises, that we should put pizza ovens at the top of the racks and then go into the pizza business. But they said no, the cheese drops down and messes up all the circuit boards. That was a dumb idea so we didn’t do that.
Anyway, we were using manual controls roughly on the order of once a week. So we’d gathered a lot of data about how well we had managed to cool the system and then we would adjust the parameters manually. Well, we decided well maybe we can get the neural chips to learn how to make more efficient the cooling system. You know, minimize the amount of power, how much water flow, and so on. And so we tried that out at one of the data centers, and after a time, we found that we could reduce the cost of the cooling system by 40%. Not four percent, forty percent. So of course the next reaction is, “Well let’s do that with all the data centers.” So this is a kind of dramatic example of how the machine’s ability to respond very quickly to a large amount of data and to learn to adjust parameters quickly makes a huge difference.
There’s another situation in a company that is experimenting with fusion, as in fusing hydrogen together to make helium, or fusing protons together with boron in order to make carbon. Which is a really interesting process because it’s not radioactive. Instead of taking hydrogen and tritium, for example, and trying to fuse that (you generate a bunch of neutrons and it becomes very radioactive), this particular design creates a plasma of boron and then you feed protons into it and the fusion creates carbon which then splits into three different alpha particles which are not radioactive. So it’s pretty cool.
Anyway, the problem is that the plasmas in fusion systems are very unstable. And human beings simply can’t react fast enough. This is the one thing about Star Trek that I always found completely bogus. You know, they’re flying around a half light speeds and everything, and the captain is saying, “On my mark, fire.” Come on. That’s five hundred milliseconds of round-trip time delay and everything. It wouldn’t work. But it’s no worse than the science fiction movies you see with the astronauts that’re orbiting Saturn and they’re having real-time communication with the guys in Houston. You know, “Houston we have a problem.” That’s baloney, but it would make for a boring movie if you had to wait for several hours for the interactions. So this is sort of artistic license.
But what was interesting about the plasma fusion case is that the plasma could be stabilized by using this kind of a neural network to detect and respond to instability. And so we’re starting to see all kinds of interesting possibilities here with the neural chips. Robotic controls similarly. The ability to use these things to stabilize motion, for example. And to do so quickly so that instead of inching your way forward to pick up the glass, you have a much more smooth ability to see things, figure out how to approach them, and pick them up. And obviously I’ve mentioned game learning already, and machine learning generally speaking, are all areas in which these neural networks are starting to be applied.
So I want to leave you with a sense on this topic that there is a wide-open space now for exploring ways of using neural networks or using hybrid systems, neural networks as part of it, taking advantage of the machine learning training and the conventional computing and melding those together. I can’t overemphasize enough that this is essentially all software. And there is no limit, there’s no boundary on software. It is a limitless opportunity. Whatever you can imagine, you may in fact be able to program. And so this leaves you with an enormous amount of space in which to explore the ability to create these systems to interact with the real world, to interact with the virtual world.
One of the most important recent developments over the last decade, I would say, is applying computers to scientific enterprise, whether it’s gathering large amounts of data and analyzing it, or simulating what might happen. So for example, a couple of years back, maybe three years? the Nobel Prize in chemistry was shared by three chemists who had not done wetland experiments. They had used computer simulations to predict what was going to happen with molecular interaction. And the fact that the computer was capable of doing enough computation to do that, and the results were in fact demonstrable by measurement, suggests that we’ve entered a very new space of scientific enterprise where computing has become part of the heartland of a lot of it. So the astrophysicists and the chemists and the biologists are all having to become computer programmers or finding some to help them do their work.
I thought I would finish up with some examples of artificial intelligence at work. And so let’s try our self-driving cars to start with.
[Cerf’s comments during playback appear below, with timestamps approximate to the source videos.]
https://www.youtube.com/watch?v=cdgQpa1pUUE
[0:34] This is an older model of our self-driving cars. The new ones are cuter. And they don’t have steering wheels, brakes, and accelerators. So that’s right, you don’t need any hands because there’s nothing to grab.
[1:07] This is one of our blind employees.
So we’re hopeful that these self-driving cars— We’ve now driven a couple million miles in the San Francisco area with these things. There have been a few accidents, none of them serious, although one of them was kind of amusing. The car came up to an obstruction in the lane it was in, and there was this big bus to the left of it. And so apparently the car decided that if it just kind of inched its way around the obstruction that the bus would get out of the way, except it didn’t. And so we had this enormous collision at 3mph with the bus.
There was another situation where we were monitoring the car as it was driving, remotely, and it stopped at an intersection and didn’t go anywhere. And so we tapped into the video to find out what was happening there. And there was a woman in a wheelchair in the intersection chasing a duck with a broom. And I confess to you I wouldn’t know what to do and the car just sat there saying “there’s stuff moving; I don’t want to run into it.” So I would have done the same thing.
So I thought that you might find it amusing to see what we’ve done at Boston Dynamics. Boston Dynamics was acquired by Google a couple of years ago. We are actually going to sell it again to another company. But some of its robotic work has been simply astonishing, and so I’m going to show you videos of several of the Boston Dynamics robots. These were largely developed under DARPA support, the Defense Advanced Research Projects Agency, which funded the Internet effort and the ARPANET and many other things as well. So let’s see what their Atlas robot looks like.
https://www.youtube.com/watch?v=9kawY1SMfY0
[0:18] Now this is really impressive. Uneven ground covered with snow and ice.
[0:45] That must be the 90 proof oil that it’s been consuming. The recovery is incredible, though. I mean really when you think about it.
[1:22] Sort of waiting for it to go, “Ta da!” right.
[1:27] Okay, now this is…you’re about to see robot abuse.
[2:17] I’m waiting for the, “Alright, you little twit.” That’s impressive. It got back up again.
[2:30] And now he’s getting the hell out of there.
Do you notice a certain kind of affinity that you formed because of this largely humaniform thing?
https://www.youtube.com/watch?v=M8YjvHYbZ9w
[0:20] What the hell was that thing?
[0:21] Here we go again. That’s why we’re selling Boston Dynamics, you know. They’re just a bunch of mean people.
[0:40] You can almost believe this is a dog.
[0:48] Now, interesting design here. Uneven ground. And these are really low-level routines that allow it to move. This is not like it’s thinking through every single step.
[1:03] And you can see they miss and they recover. So, we learned a lot about how to make these things work without having think through every single motion.
[1:22] Best buddies.
[1:48] The idea here was to invent robots that could carry things, so from a military point of view these need to be pack-bots, in a sense.
[1:56] Now that’s the big horse. And what you’re hearing is the noisy motor that runs it. Eventually the military decided it probably wasn’t the best thing to use out in the battlefield because you’d signal to everybody that you had this big damn thing out there. So so much for stealth.
Oh, this is worth watching:
https://www.youtube.com/watch?v=S7nhygaGOmo
[0:15] You wonder what this dog is thinking.
[0:26] The little guys always take on the big guys.
There are about fifty-two or something of these videos available on YouTube if you haven’t already encountered them. I think I have one last one. Yes, this one:
https://www.youtube.com/watch?v=q2gehrAhflQ
[0:05] Okay, this is not what I thought I was going to show you, and I haven’t seen this one so I have no idea what’s about to happen. This is what happens when you go to a URL where the destination turns out not to have what you thought it was.
[1:25] I’m waiting for it to back up against the tree and squirt some oil on it.
[1:30] I’m going to stop this one and see whether I can find the— Well, I don’t know. This this might be it. If it isn’t then we’ll sort of finish up.
Okay, I don’t see the one that I wanted to show you, which I can’t find is the one where a tiny little dog got built that was smaller than Spot. But I’m afraid… Okay.
Well I think you get the idea first of all that we have a lot of fun with this stuff. But most important, these devices actually have the potential to do useful work. And people who are nervous about robots and say they’re going to take over and so on I think are overly pessimistic about this. It’s my belief, from the optimistic point of view, that these devices, the programmable devices of the Internet of Things, and the artificial agents that we build will actually be our friends and be useful.
However I think we also need to remember that they are made out of software. And we don’t know how to write perfect software. We don’t know how to write bug-free software. And so the consequence is that however much we might benefit from these devices and programmable things in general, we also have to be aware that they may not work exactly the way they were intended to work or the way we expect them to. And the more we rely on them the more surprised we maybe when they don’t work the way we expect.
And so this sort of says how we’re going to have to adjust to this 21st century where we are surrounded by software, and that the software may not always work the way we expect it to. Which means that we have to build in a certain amount of anticipation for when things go wrong. And we hope that the people who write the software have thought their way through enough of this so that the systems are at least safe, even if they don’t always perform as advertised.
And so that’s my biggest worry right now generally with the Internet of Things, with robotic devices, and everything else. That we preserve safety and reliability and top of our list of requirements, after which we need to worry about privacy and the ability to interwork among all the other devices.
So this is early days for all of these technologies. And we won’t see the end of this, presumably. Our great-grandchildren will. And in the long run I am convinced that they will do us a lot of good. I sure hope I’m right, because as I get older I might rely on these little buggers. Thank you all very much for your time.
Further Reference
Lee Rainie also hosted a discussion and Q&A session with Dr. Cerf earlier that day.
Overview post about the award ceremony.