Gideon Lichfield: Hello everybody. Welcome to the session on Compassion Through Computation: Fighting Algorithmic Bias. I’m Gideon Lichfield, I’m the editor of MIT Technology Review. And I’m going to be moderating the discussion with two very interesting speakers. Joy Buolamwini, who is currently doing she says her fourth degree at MIT and is founder of the Algorithmic Justice League, and uses a variety of different forms of expression to examine the way in which algorithmic bias affects the AI technology that we use, and describes herself also as a poet of code. And then, Justine Cassell is the Associate Dean of Technology, Strategy, and Impact at the School of Computer Science at Carnegie Mellon.
I’m gonna ask Joy to speak first and then Justine, then we will have a Q&A. And you can ask questions by raising your hand, but if you feel like using technology, there is this app we are using called Slido. You can access it by this website, wef.ch/betazone. You can go in there, you can put in questions that you want to ask the speakers. Those will come up and then we will be able to select from them and lead the discussion. So, I’ll ask Joy to step up first. Thank you Joy.
Joy Buolamwini: Hello, my name is Joy Buolamwini, founder of the Algorithmic Justice League, where we focus on creating a world with more inclusive and ethical systems. And the way we do this is by running algorithmic audits to hold companies accountable.
I’m also a poet of code, telling stories that make daughters of diasporas dream, and sons of privilege pause. So today it’s my pleasure to share with you a spoken word poem that’s also an algorithmic audit called “AI, Ain’t I A Woman?” And it’s a play on Sojourner Truth’s 19th-century speech where she was advocating for women’s rights, asking for her humanity to be recognized. So we’re gonna ask AI if it recognizes the humanity of some of the most iconic women of color. You’re ready? [The Davos recording omits most of the corresponding visuals for Joy’s piece; the following is her published version.]
https://www.youtube.com/watch?v=QxuyfWoVV98
So there you are. [applause] And so what you see in the poem I just shared, which is also an algorithmic audit, is a reflection of something I call the coded gaze. Now, you might have heard of the white gaze, the male gaze, the post-colonial gaze. Well, to this lexicon we add the coded gaze, and it is a reflection of the priorities, the preferences, and also sometimes the prejudices of those who have the power to shape technology. So this is my term for algorithmic bias that can lead to exclusionary experiences or discriminatory practices.
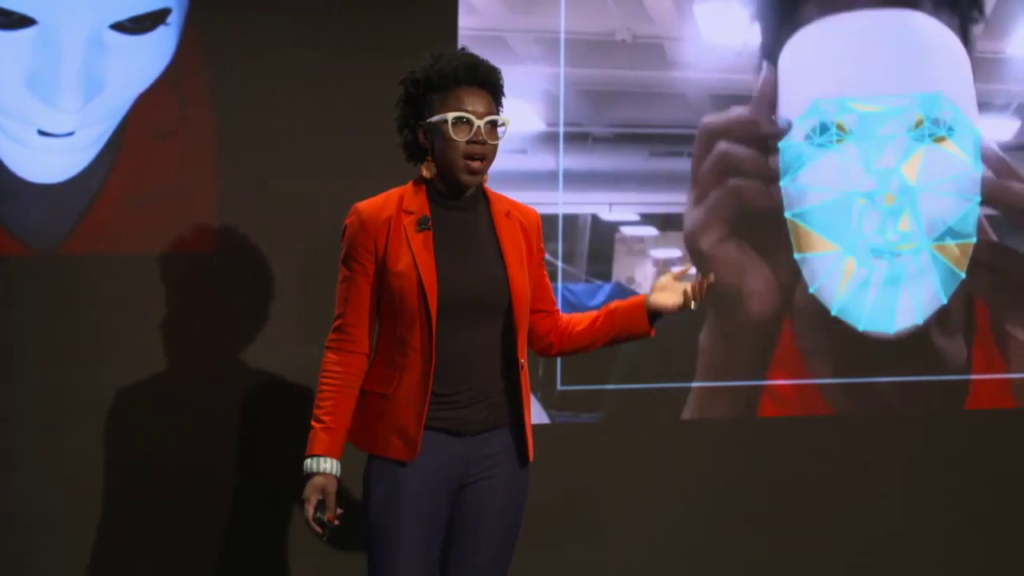
So let me show you how I first encountered the coded gaze. I was working on a project that used computer vision. Didn’t work on my face until I did something: I pulled out a white mask. And then I was detected. So I wanted to know what was going on, and I shared this story with a large audience using the TED platform; over a million views. And I thought somebody might check my claims, so let me check myself.
And I took my TED profile image and ran it on the computer vision systems from many leading companies. And I found some companies didn’t detect my face at all. But the companies that did detect my face? labeled me male. I’m not male; I’m a woman, phenomenally. And so I wanted to know what was going on.
Then I read a report coming from Georgetown Law showing that one in two adults, over 130 million people, have their face in the face recognition network that can be searched unwarranted, using algorithms that haven’t even been audited for accuracy. And across the pond in the UK where they actually have been checking how these systems work, the numbers don’t look so good. You have false match rates over 90%; more than 2,400 innocent people being mismatched. And you even had a case where two innocent women were falsely matched with men. So some of the examples that I show in “AI, Ain’t I a Woman,” or the TED profile image, they have real-world consequences.
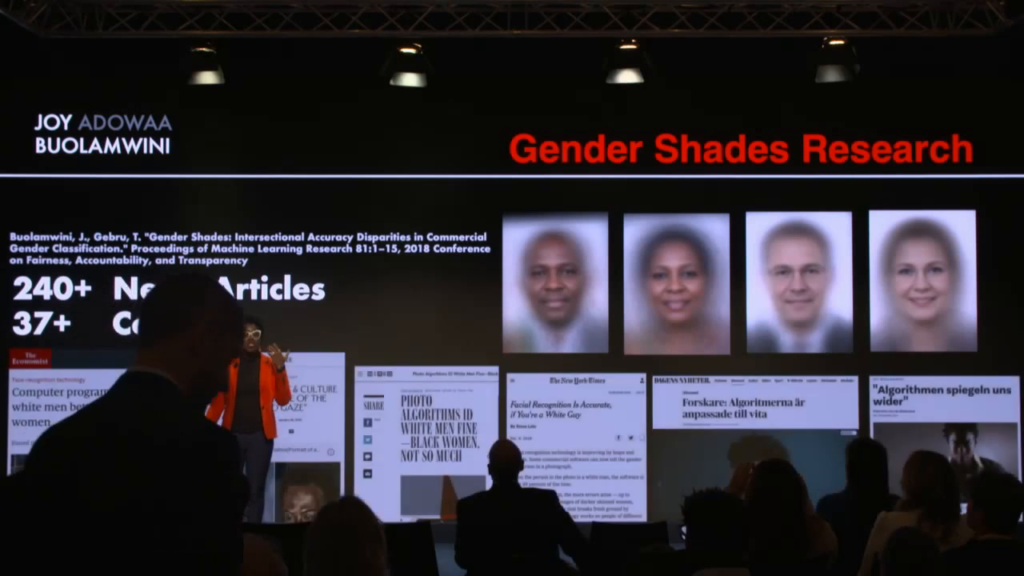
And because of the real-world consequences, this is why I focused my MIT research on analyzing how accurate systems work when it came to detecting the gender of a particular face. And so with the research we’re doing, it’s been actually covered in more than thirty countries, more than 240 articles, talking about some of the issues with facial analysis technology.
So in order to assess how well these systems actually work, I ran into a problem. A problem that I call the “pale, male data issue.” And in machine learning, which are the techniques being used for computer vision (hence finding the pattern of a face), data is destiny. And right now if we look at many of the training sets or even the benchmarks by which we judge progress, we find that there’s an overrepresentation of men—75% male for this national benchmark from the US government. 80% lighter-skinned individuals. So pale, male data sets are destined to fail the rest of the world, which is why we have to be intentional about being inclusive.
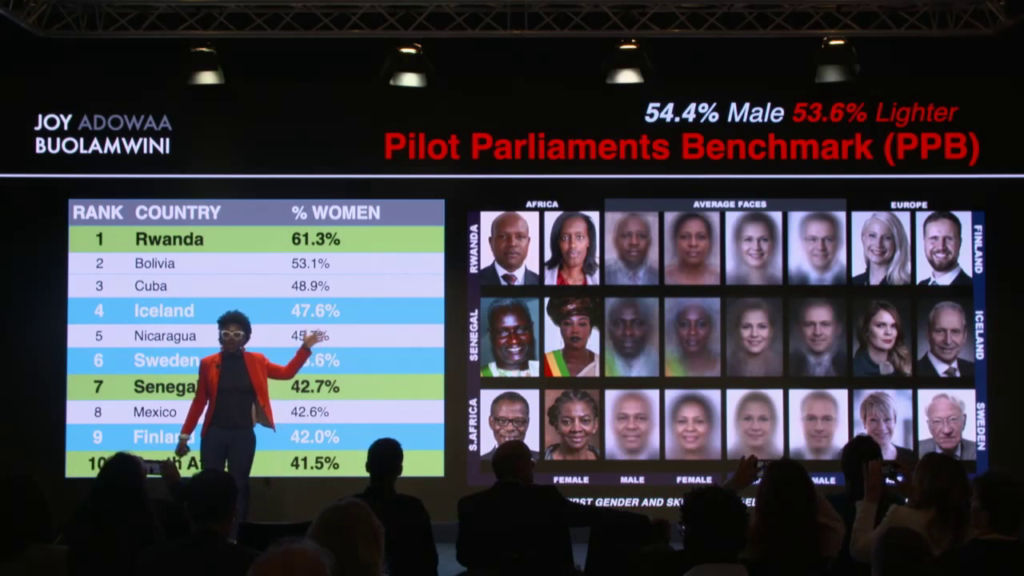
So, the first step was making a more inclusive data set, which we did, called the Pilot Parliaments Benchmark, which was better balanced by gender and skin type. The way we achieved better balance was by going to the UN Women’s web site, and we got a list of the top ten nations in the world by their representation of women; Rwanda leading the way, progressive Nordic countries in there, and a few other African countries as well. We decided to focus on European countries and African countries to have a spread of skin types.
So finally with this more balanced data set, we could actually ask the question, how accurate are systems from companies like IBM, Microsoft, Face++—a leading billion-dollar tech company in China used by the government, when it comes to guessing the gender of a face?
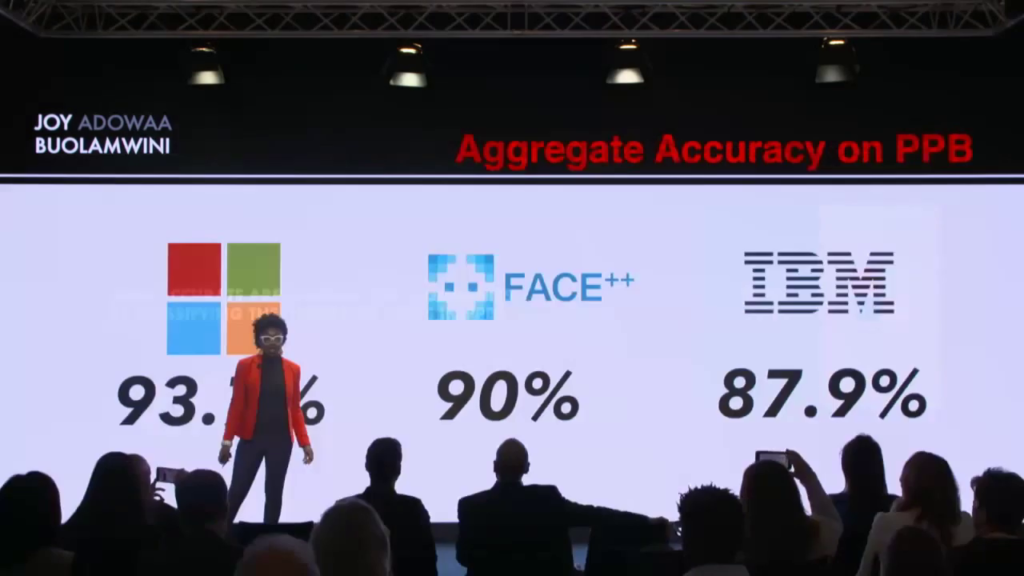
So what do we see? The numbers seem okay. 88, maybe you get a B with IBM. 94%, Microsoft is the best case overall. And Face++ is in the middle. Where it gets interesting is when we start to split it down.
So when we evaluate the accuracy by gender we see that all systems work better on male faces than female faces, across the board. And then when we split it by skin type, again we’re seeing these systems work better on lighter faces than darker faces.
Then we did something that hadn’t been done in the field before, which was doing an intersectional analysis borrowing from some of Kimberlé Crenshaw’s work on antidiscrimination law, which showed that if you only did single-axis analysis, right, so if we only look at skin type, if we only look at gender, we’re going to miss important trends.
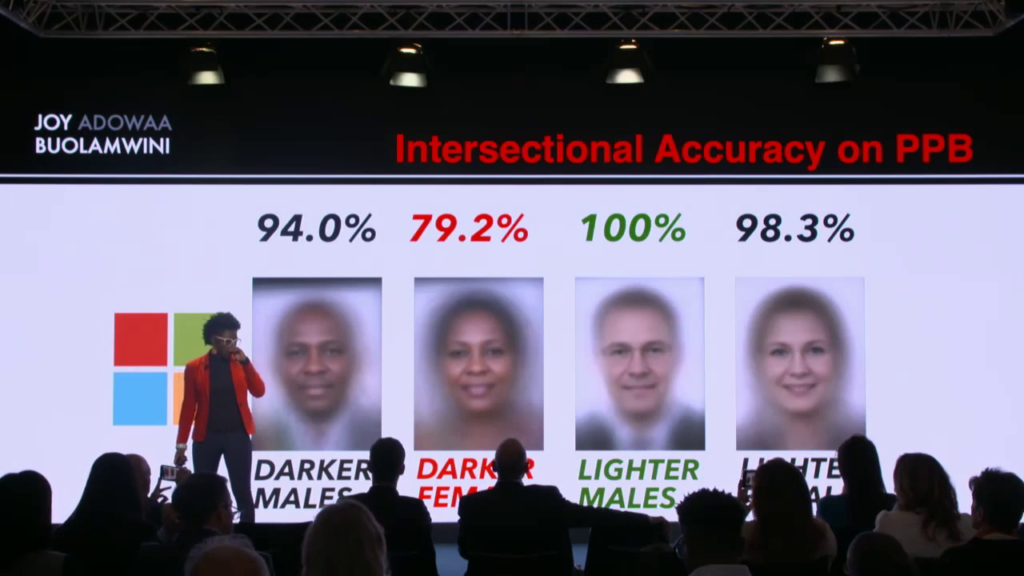
So, taking inspiration from that work, we did this intersectional analysis. And this is what we found. For Microsoft you might notice that for one group there is flawless performance. Which group is this? The pale males for the win! And then you have not-so-flawless performance for other groups. So in this case you’re seeing that the darker-skinned females are around 80%. These were the good results. Let’s look at the other companies.
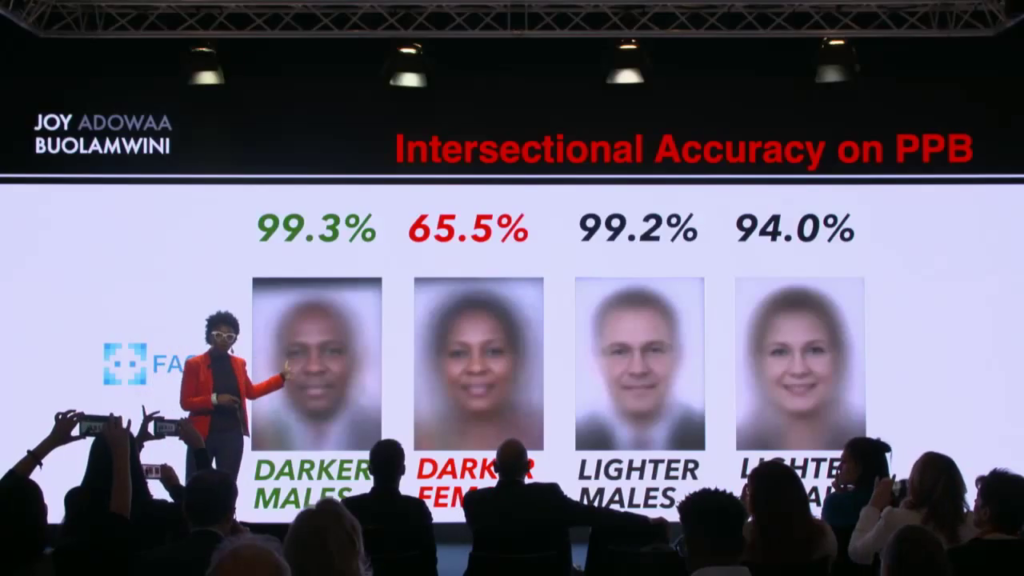
So now let’s look at Face++. China has the data advantage, right, but the type of data matters. And so in this case we’re actually seeing that the better performance is on darker males marginally. Again, you have darker females with the worst performance.
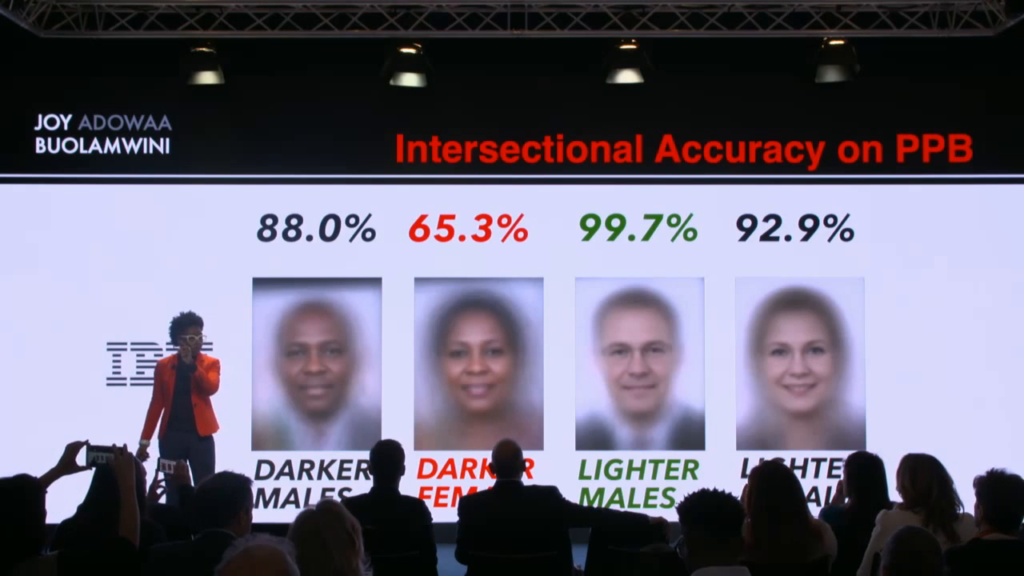
And now let’s look at IBM. For IBM lighter males take the lead, again. Here you see that for lighter females there’s a disparity, right, between lighter males and lighter females, but lighter females actually have a better performance than darker males. And categorically across all of these systems, darker females had the worst performance. So this is why the intersectional analysis is important, because you’re not going to get the full spectrum of what’s going on if you only do single-axis analysis.
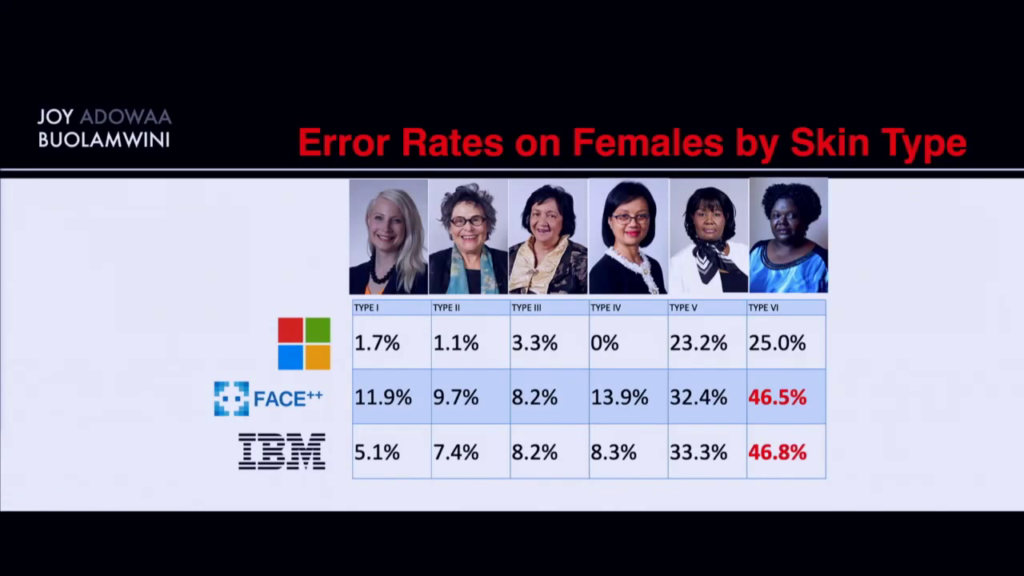
Now we took it even further and we disaggregated the results of the darker females since that was the worst-performing group. And this is what we got. We got error rates as high as 47% on a task that has been reduced to a binary. Gender’s more complex than this, but the systems we tested used male and female labels, which means they would have a 50/50 shot of getting it right by just guessing. So for these systems, we’re paying to do an audit that actually shows is marginally better than chance.
So I thought the companies might want to know what was going on with their systems, and I shared the research. IBM was by far the most responsive company; got back to us the day we shared the research and in fact released a new system when we shared the research publicly. So first we gave the research privately to all of the companies and gave them some time to respond.
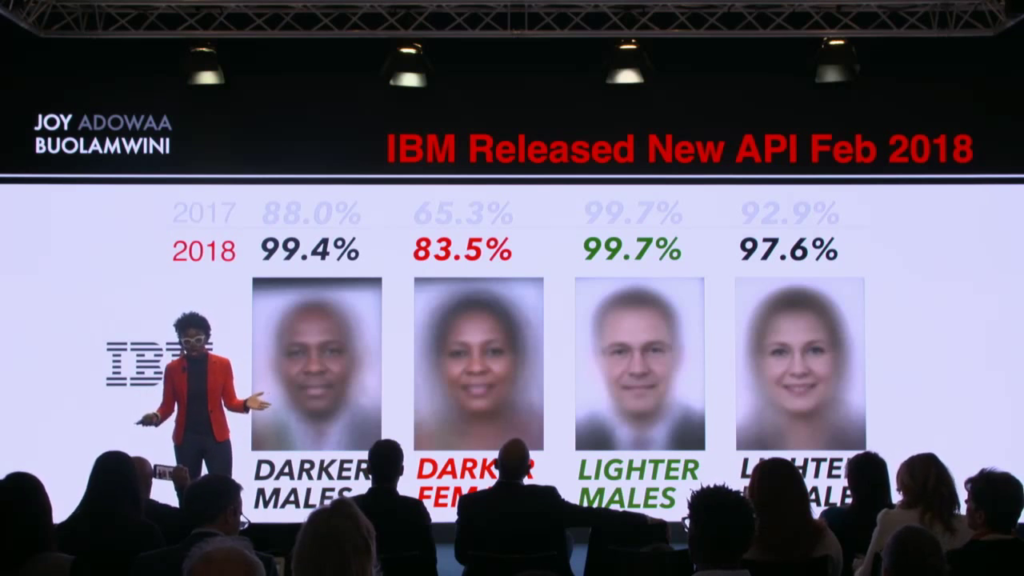
So here you can see that there’s a marked improvement from 2017 to 2018. So for everybody who watched my TED talk and said, “Isn’t the reason you weren’t detected because of you know, physics? Your skin reflectance, contrast, etc.” The laws of physics did not change between December 2017 when I did th study and 2018 when they launched the new results. What did change was they made it a priority. And we have to ask why.
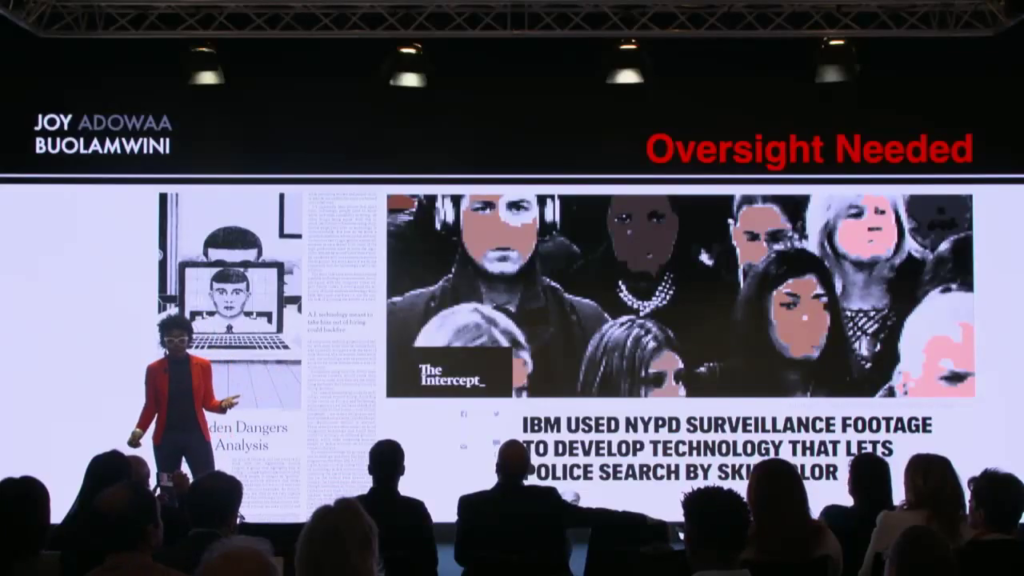
So, this past summer you actually had an investigative piece that showed that IBM reportedly secretly supplied the New York Police Department with surveillance tools that could analyze video footage by skin type—skin color in this case, and also the kind of facial hair somebody had, or the clothing that they were wearing. So enabling tools for racial profiling. And then for The New York Times I wrote an op-ed talking about other dangers [of the] use of facial analysis technologies. We have a company called HireVue, for example, that says we can use verbal and nonverbal cues, according to their marketing materials, and and infer somebody’s going to be a employee for you. And how we do this is we train on the current top performers. Now, if the current top performers are largely homogeneous, we could have some problems.
So it’s not just a question of having accurate systems, right. How these systems are used is also important. And this is why we’ve launched something called the Safe Face Pledge. And the Safe Face Pledge is meant to prevent the lethal use of facial analysis technology. (Don’t kill people with face recognition, very basic.) And then also thinking through things like secret mass surveillance, or also the use by law enforcement.
So so far we have three companies that have come on board to say we’re committed to the ethical and responsible development of facial analysis technology. And we also have others are saying we’ll only purchase from these companies. So if this is something that you’re interested in supporting, please consider going to the Safe Face Pledge site. And if you want to learn more about the Algorithmic Justice League, visit us at ajlunited.org. Thank you.
Justine Cassell: You’ve heard beautifully about one aspect of bias in companies today, and that’s algorithmic bias. In the same way we want to be taught by educators who represent us and we want politicians who represent us, we also want technology to represent us, in every sense of “represent.” We want it to look like us; we want it to mirror who we are; and we want it to stand up for us—share our values and preserve them.
But that really hasn’t been the case, yet. And that’s what I’m gonna talk to you about. I’m gonna talk about the kinds of bias that are leading to technology letting us down. I’m gonna talk about why it happens, and I’m going to start to talk about what to do about it.
But first let me take you back to France in 1984. So in France in 1984 the government set up a terminology commission. It’s a very French thing. They’d had a whole bunch of terminology commissions before. And the goal of this one la féminisation des noms de profession. That is, the invention of names for jobs traditionally done by men that may now or one day be done by women. Like doctor, professor, researcher, postwoman, and so forth.
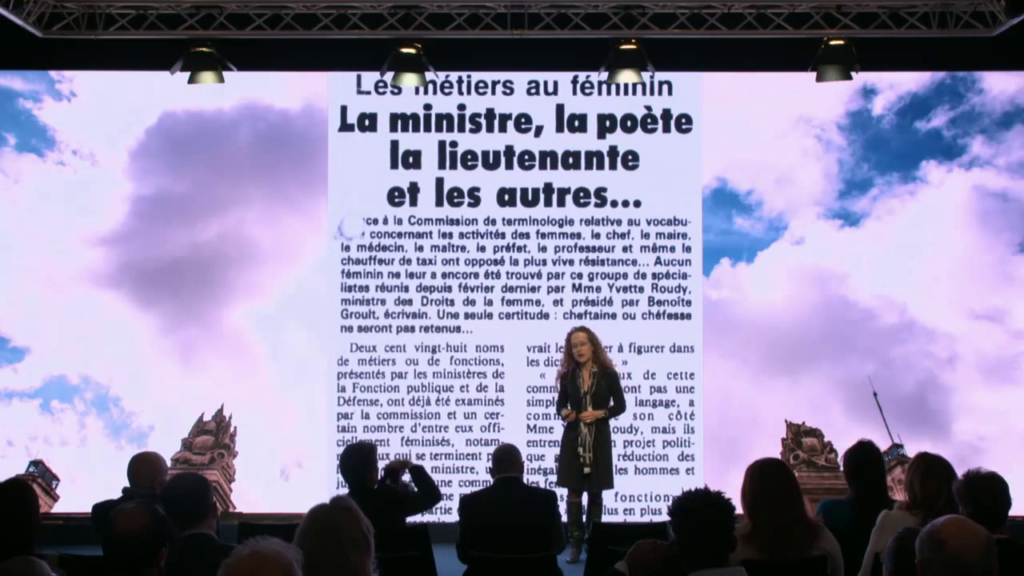
And they released a report about the words they had invented—the neologisms that they had invented for these new jobs. Like a woman doctor. Or a woman mail deliverer, and so forth.
As the report was released, there was a counter-report. (And that’s also very French.) And this counter-report was released by the Académie Française, who sees themself as in charge of the French language, standing up for and insuring that the French language remains pure. And what they said was “This very impulse of yours is honorable, but it’s going to lead to barbarisms and segregation, and worse sexism than we had before, because it’s unreflective and unmotivated by research.”
Well to any researcher, that’s a dream. So I decided to do an experiment to look to see who was right. Was the feminization of the terms for jobs going to lead to more women moving into those jobs, as the terminology commission suggested? And so I went to the US and I went to France, and I did an experiment with children of 8 or 9 years old. They’re starting to think about gender.
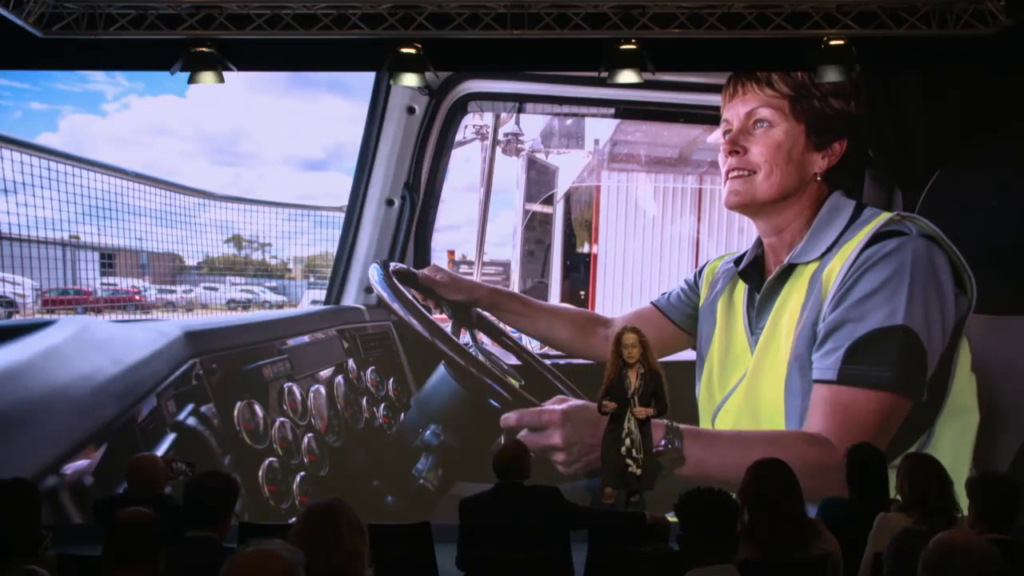
And I asked them what they would call this picture. This is a female truck driver. The word’s a little unfortunate. Luckily 8- and 9‑year-olds don’t know this. Those of you who are French speakers know that camioneusse unfortunately already has a meaning that we might not want to use. But in this instance we asked them simply what they would call this person.
What they would call this person—a woman researcher: chercheuse. What they would call this person—un doctoresse, a woman doctor. And what they would call this person—un postière, a female mail deliverer.
And in fact what I found was that it was children who scored most highly on a test of stereotype bias, those children who had the narrowest beliefs about who could do what, that used the feminized terms. And that makes no sense, you might think. Why? And I asked them: why? Why would “doctoresse” not mean a woman doctor?
They explained “doctoresse” is kind of like a doctor but not a real doctor. That’s why we call her a doctoresse.
And that’s because language reflects life, and not the other way round. And so when you want to change something, you can’t simply change the words. You can’t simply change the pictures. You have to change society. And that’s what we’re gonna turn to now.
So I cofounded, with a number of other very smart people, a nonprofit called EqualAI.org. And I invite you to join us online and also to get to speak to Miriam Vogel, the new executive director who’s here in Davos. We realized that very well-intentioned people can do very nasty things. And we started this foundation, this nonprofit, with that in mind.
We looked at the stats. We saw that the numbers of women going into computer science are going down and not up. That parents say that they’d like their children to be computer scientists so that they can earn more, but don’t want their girl children to take computer science classes. We know that in less-resourced schools computer science isn’t even taught.
And why is that? And what do we do about it? Why does it matter? A parent said to me, “I don’t understand you. Why would you want my girl to become a computer scientist? That’s a sucky profession. It’s a bunch of badly-dressed, non-washed, greasy-haired men eating Cheetos, and drinking Red Bull, and staying up all night alternating between writing code and playing video games.”
You see the problem here? So, what I suggested was I don’t want to make any girl become a computer scientist. But I want every profession to be available to girls, to people of color, to other underrepresented groups, those of different abilities. Because if not, we’re going to amplify the worst of ourselves in technology. We’re going to amplify our ability to kill. Our ability to destroy. Our ability to hate. And not the best of us. And it takes intentionality, and it takes work to create technology that amplifies the best of us. Human-centered technology.
Now Joy spoke beautifully about one aspect of that human-centered technology that has not been intentionally created. That’s relied on what’s called a “convenience sample.” My students define “convenience sample” as your two office mates and the two office mates across the hall. And that’s not really what you want when you build a piece of technology. And the people who built those algorithms grab the first data set available, and it was the pale males.
But there are other kinds of bias. As well as algorithmic bias, there’s also bias in what the workforce looks like, and bias in what the technology looks like. And in all three arenas, we are not represented. Neither what we look like, what we sound like, what our values are. And what we want them to be.
And this all happens for a reason that is not intentional for the most part. And that is because of the psychological notion of stereotype. Now when we talk about stereotypes usually what we mean is negative beliefs about a person. But that’s not what a stereotype is in technical language. A stereotype is the ability to take in information, and rather than needing to take in the huge stream of information that comes at us every second, we grab a piece of it and extrapolate. We look at an eye, for example, and we say, “Oh. Totally. Olive-shaped eye…pseudo-ethnicity: Asian. Really good at math. Mmm…not so good at interpersonal relationships. Won’t argue in public.”
Now the first part of that, the Asian, that’s an extrapolation from one little detail. What it allowed me to do was not look at the rest of that body, or hear that person talk, or have a conversation with that person, but simply to pattern-match what’s in the world with what’s in my head. And pattern-matching is a lot faster than taking in information.
But, as you saw, it has dangers. And here are some others. Because when we match a kind of person to a set of traits like “good at math” or “not willing to argue,” sometimes that’s fine. There’s a great old movie called Pillow Talk where Doris Day is talking to Rock Hudson, and he’s talking in a Southern accent. He turns out to be a con man. But she hears him speaking in his Southern American accent, she says, “He’s so cute. He’s so sweet. So naïve, so innocent.” And he’s not at all. But she extrapolated from that one datum to that.
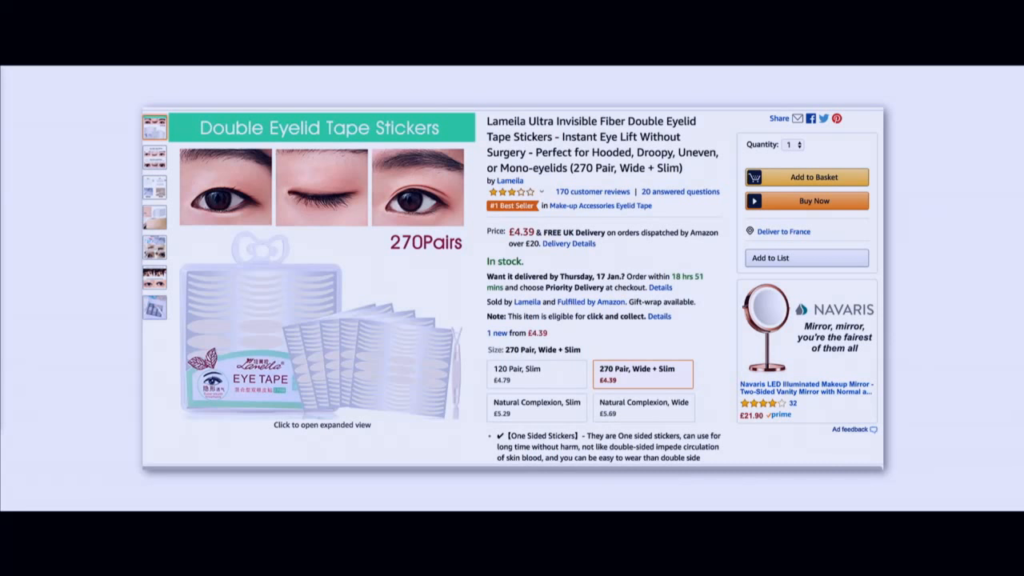
Miley Cyrus thought it was okay to represent herself with slanty eyes. But by doing that she extrapolated to all of those other traits. And that leads people in those negatively-stereotyped groups to try and become like the norm. Like Joy wearing a white mask. This is an actual product for sale to keep your eyelids looking Caucasian, non-Asian. And that’s a very sad thing.
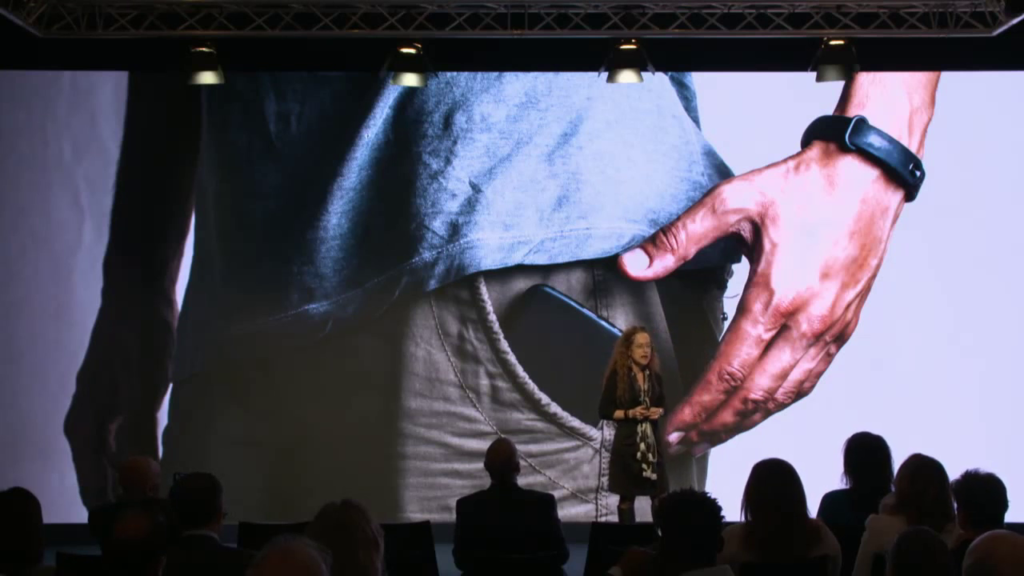
So, it leads to even worse things than that. For example, it turns out that when the hand holding a cell phone is black, people are way, way more likely to see that phone as a gun. And this is unfortunately even more true of policemen than it is of everyday people. So you can imagine a young person grabbing a phone and being shot dead. And it unfortunately has happened way too often, and continues to happen.
And things like that lead to saying, “I’m looking for people to work on my team. I need people who are gonna succeed. People who are gonna succeed like I succeeded. I’m from a group that fits here. We need people who fit in.” And only a few weeks ago a friend of mine went for an interview in Silicon Valley, and when she didn’t get the job she said, “Can you tell me why?” And the hiring manager said, “You just don’t fit.”
Yeah, you’re right, I don’t. I don’t wear the same size. I’m not the same height. My skin happens to be a different color. And you need that. Why do you need that? Because a bro culture, a culture where everyone looks alike, which is what Silicon looks like now, creates bro products. Our students from Carnegie Mellon come back and tell me—and this has changed over the last couple of years—that their bosses tell them to create for themselves. “Design technology that you would love.” Even narrowing that field of technology.
And yet we know that diversity in teams creates innovation. That is, it has been shown, as definitively as we can show anything, that the more perspectives, the more different points of view, different kinds of people we have on a team, the more objective technology innovation will be created.
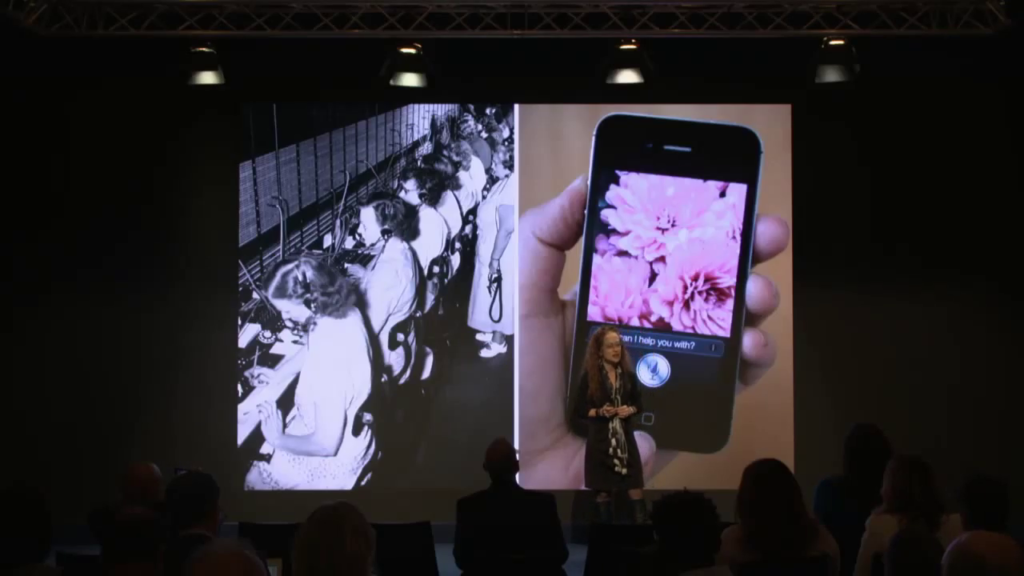
This is not an example of that. This is an example of creating a technology that fits a stereotype. That is, Alexa is a servant. In the same way that girls (those are young women), were asked to be phone operators because they had soft voices and gentle temperaments, and could serve those people who use the phone, Alexa does the same thing. And that’s why in the UK, Siri had a male voice. Because there the male valet, or butler, was enough of a stereotype to allow men to serve. But that disappeared in the face of US Alexa, and it’s now a female voice. This stereotype gets more and more noxious. Taxi drivers in Germany refuse to have a GPS with a female voice. They refuse to take instructions from her on how to drive.
And an even uglier example, unintentionally for sure, comes from a paper on virtual tutors teaching children math. These are four “virtual tutors,” four representations. Children were allowed to choose—this is not my work. Children were allowed to choose whichever one they wanted. And they chose the one to use first that looked like them—same gender, same ethnicity. But they learned more math from the white male.
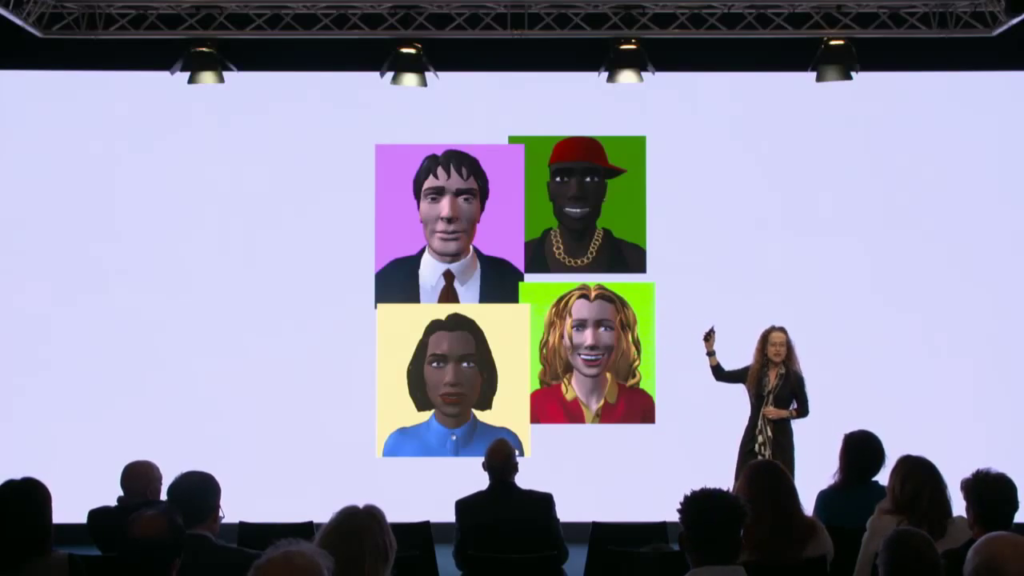
And that’s not surprising. If you look at the representation in these pictures, these are stereotypes of what a scientist sitting in his armchair believes black men and women and white women and white men look like.
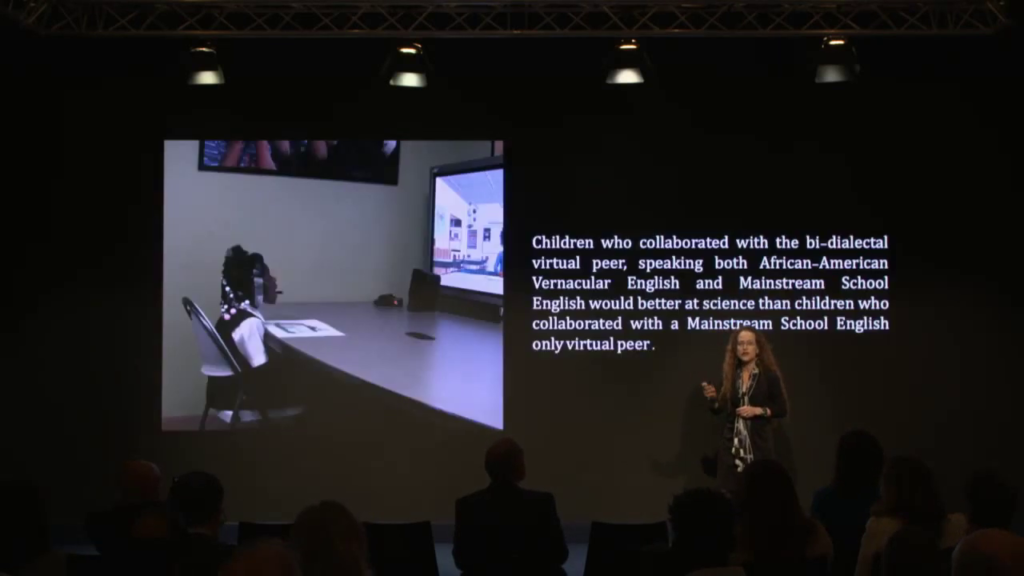
So we did an experiment. We built two versions of a piece of knowledge. They looked identical but one spoke the same dialect as the children we were working. Took us two years to build a grammar of that dialect. And the dialect is just language without an Army and a Navy. Two versions of that piece of technology. And children worked with the technology to do science. And it turned out that the children who worked with the technology that sounded like them learn more science. So this has real-world consequences that we have to pay attention to.
So what do we do? We’re at an inflection point, and it’s both a risk and an opportunity. And this is a fourteen-minute talk and not fourteen hours, and I’m happy to talk to anyone who wants to know more. But the inflection point is that the future of work is not gonna be like the past of work. We have to grab hold of that. We have to have inclusion and diversity officers on the team that digitizes the company. That builds the platforms, the performance algorithms, the metrics, and the policies that govern change. It’s an opportunity. Because all of our companies and our universities are in the middle of change. And women, people of color, people of different abilities, need to be at every stage of that change. Not just women engineers but women product designers. Women marketers. Unless we do that, we won’t have a diverse group like this.
And I want to say something that doesn’t get said often enough. We need to invest in the pipeline. We need to make it okay for girls to be engineers without having to have greasy hair, like Cheetos, or drink Red Bull. It has to be okay. But, the pipeline is leaking just as badly at the top. Senior women when they get to senior positions are told that they’re difficult. They’re hard to manage. They’re just not right. And they don’t stay in those positions because they’re kicked out.
So you can’t just hire women. You need to keep them. And to do that you need what’s called the cohort effect. Not one but a minimum of three. Not one person of color but a minimum of three. For anyone who’s seen The Intern, not one old man but a minimum of three. And if you do that, if you have older women and younger women, then you’ll have role models for people to look up to. You’ll have cohorts to talk to one another.
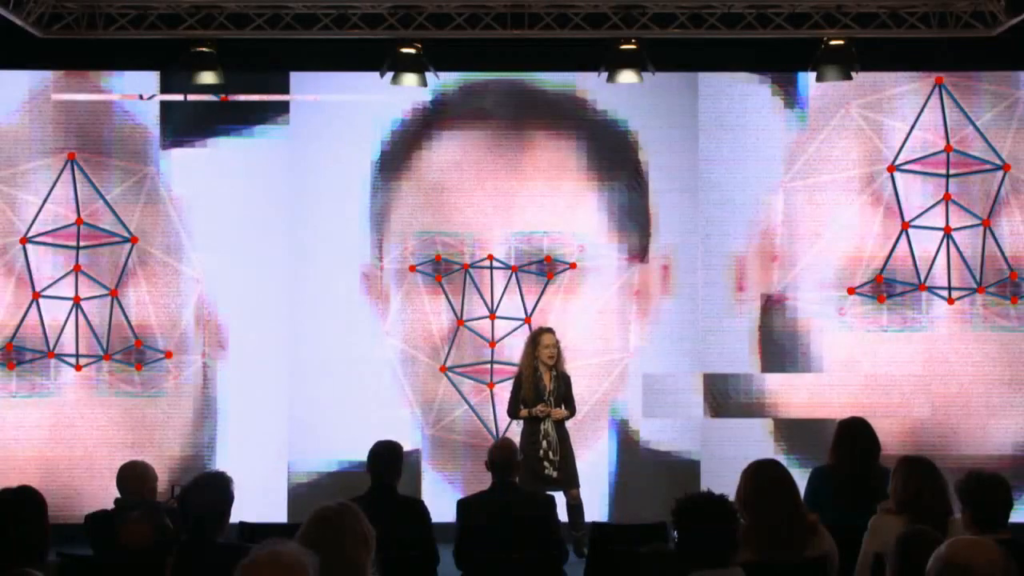
So we’ve talked about two kinds of bias. And here’s the third. One is the representation of us in the workforce. The second is the representation of us in the technology that we use, such as Siri and Alexa. And the third is algorithmical bias. If we pay attention to this, we can have a workforce that looks like the people that we’re building for. And all of us win if that happens. Thank you.
Gideon Lichfield: Okay. So thank you very much Justine and Joy. So, as a reminder, you can— I’m going to do some audience Q&A in a moment. And if you want to jot down some questions and put them in so they’ll show up on my screen you can go to the wef.ch/betazone. But I will also take questions in the analog method.
But first I’m going to try to formulate a question… And I’m not sure if I’m going to formulate it very well, but I’ll try to formulate a question that is to both of you that kind of encompasses what you were both talking about.
We are now…we’re in an era and we’re moving into an era of ever more personalization and optimization. And this is true in the algorithms that figure out what we might want to buy and how to sell it to us. And what clothing will fit us best and will match the purchases we’ve made in the past and so on. It’s also going to be true of the software that is going to help employers make decisions about who they should look for and where they should recruit. It’s in the software that is going to be increasingly used to monitor how people perform at work, and how they could perform better and more optimized. And inherent in all of that optimization and personalization, inevitably there are going to be correlations between certain kinds of behavior. And whether gender, or race, or socioeconomic class, or other things. So I think the question I’m trying to formulate is, how in this world of increasing optimization where the algorithms will be accurate… They’ll increasingly be accurate. But their application could lead to discrimination. How do we stop that?
Justine Cassell: Do you want to go first?
Joy Buolamwini: Sure. So, accurate algorithms can be abused but we always have to remember that accuracy is always relative. And as we learn more the systems that we thought would be more precise might not actually be doing what we think. So let’s take the example of precision medicine. The right medicine for the right person, at the right time. And when you look at what some startups are doing, they’re saying okay we have all of this clinical data. Let’s train on that so we can make better predictions.
In the US case it wasn’t even until 1993 that women and people are color were mandated to be part of clinical trials. If you look at cardiovascular disease, one in three women die of this, but less than a quarter of research participants are women, and the way in which heart disease manifest in women and manifest in man is not necessarily the same.
So as a result you might think you’re getting more and more accurate but it could be just for a small sliver of society. So I’m always thinking about what does full-spectrum inclusion look like? And if we’re talking about precision, precisely who are we benefiting and precisely who are we harming?
Lichfield: Mmm, Justine.
Cassell: That example’s a great example, because one of the things we know about research participants is that low socioeconomic status citizens often don’t want to be part of research. Because they don’t want their data to be stolen. They don’t want people to make money off of their personal data. And that’s a tension, because if their data isn’t used; they own it, they’ve kept it; but they’re also not part of that data set. And it’s a complicated question.
But I want to talk about another aspect of personalization. What I thought you were going to say was aren’t we going to build more and more instances of technology? A white pale male, a white woman who’s old, a white woman who’s young. And there I was going to say that in my own work I’ve been working very hard to build gender ambiguous, ethnicity ambiguous, representations of technology. And what we find is—and we’ve done this mostly for children—is that children attribute their own ethnicity and their own gender-binary gender to those pieces of technology. In fact the only gendered piece of technology I’ve ever built, that looks like a woman, is SARA (for any of you who interacted with her two years ago), the socially-aware robot assistant. Other than that, all of our work has gender-ambiguous names, ethnicity-ambiguous names, and looks, so as to not fall into stereotypes that I know I have as a scientist.
And I was going to stand up here and make you all take the implicit association test to have you all see the way I’ve seen the stereotypes, the noxious stereotypes, you carry with you. The only thing that we can do is realize them, and then decide what to do about them. And until we do that, we’re capable—I’m capable—of hopefully nothing as really ugly as those math tutors, really negative as those math tutors. But stereotypes nonetheless and so I try and stay away from personalization in the realm of what technology looks like.
Lichfield: Do you feel like the companies that’re building these algorithmic tools have gotten better about issues of bias since you and other people started raising it? And also, other than IBM how did the other companies react when you approached?
Buolamwini: Sure, so we got a range of reactions. One reaction was no response. Another was a very cautious corporate response. We have a new paper that’s coming out where we look to see if our process actually made a change. And so after we did the Gender Shades research which showed racial bias, that showed a gender bias and it also showed intersectional bias, all the companies that we audited within seven months made significant improvements.
So then we decided to look at companies that we didn’t audit in the first place. So we didn’t include Amazon, for example, but Amazon is selling their technology to law enforcement. And we found out that technology has racial bias, has gender bias, and it was close to the level of the companies a year before. So here we’re seeing the companies that were checked, right, are trying to make some kind of improvement. And then the companies that weren’t weren’t held to the fire. So it definitely makes a difference. Some people are paying attention, not enough people are paying attention, but we’ll keep watching.
Lichfield: Right, so that then rai— Oh, sorry. Justine.
Cassell: I was going to say since I build personal assistants I’ve looked a lot at the personal assistants on the market, and over the last six months I’ve done a lot of press interviews. And I’ll leave the company nameless but I can say that one company, you would tell the personal assistant to go away and the personal assistant would reply, “Why? Can’t we just stay friends?” And I quoted this in the interview and it disappeared a week later. So, we can do good but we shouldn’t have to follow companies around letting them know they’re being watched.
Lichfield: Right. So who in the end should be doing this? I mean, what is the role of of law and regulation in setting how algorithms should work, especially—and this is like a perpetual problem now with technology—especially when the tech itself is moving way faster than lawmakers and legislators can.
Buolamwini: Absolutely. We have to definitely think about the maturity of a specific technology. So going back to facial analysis. With what we know about the flaws it’s completely irresponsible to use it in a law enforcement context, yet we see companies selling it in that space. So I believe one of the first legislative steps that we can take, as others have called for, are moratorium’s until we have a better understanding of the real social impact of some of these technologies.
Also making sure that there’s something called affirmative consent, where we know if I’m going into an interview and they’re going to analyze my face? I have some way of pushing back. There’s some kind of due process and I have to say yes. Right now you might have opt-out, at best? but oftentimes you’re channeled into these systems. So that’s a place where you could have regulation come in to increase transparency but also have affirmative consent.
Cassell: I’m very American in my perspective. Regulation’s okay but I’d like to diminish it as much as possible and rely on education. And I think this is an area where we don’t educate our young people. We don’t educate our old people very well about it either. And we need to introduce into the discussion about AI, into the education of AI researchers, a lot more information about society. We talk about human-centered computing. We’re starting to talk about human-centered AI. That includes a lot more than building a tool that can recognize when you’re having trouble walking and sending a wheelchair. It means knowing who people are; how they are; how they behave, with the finesse and the depth of precision that’s needed in order to design for them in a truly justice-oriented way.
Lichfield: But that requires a whole lot more data on them.
Cassell: It does, and we have those data.
Lichfield: Right.
Cassell: We’re not missing the data, we’re not using them. We’re using my two officemates and the two guys across the hall: convenience sample.
Lichfield: Right. But that’s what I mean, is because we need that much more data then it raises that many more privacy questions.
Buolamwini: And we are missing a lot of data. So let’s go and look at the Human Genome Project, right. So, let’s say people who are African or of African diaspora, about 20% of the global population. Less than 5% of what we have for the genome represents those populations. So you have severe underrepresentation of many different kinds of Asians as well. So we do have intense data gaps, but we also have to think about agency when people get to decide if they participate or not.
So earlier you were talking about various people in lower socioeconomic status not trusting. But there’s also a reason for not trusting. If you have a case where you have a population with syphilis and you don’t tell them, as happened with the Tuskegee experiments, there are very valid reasons not to trust—
Cassell: To go ahead believing that. Yeah, for sure.
Buolamwini: Absolutely.
Lichfield: There’s a question here from someone in the audience, Abinav? Is Abinav in the room? No? Okay. Because otherwise I would have them explain their question, but as it is it doesn’t really quite make sense to me. Are there any other any other questions in the room here?
Audience 1: Yes. A comment. I’m very much in Joy’s camp in terms of thinking about how the data’s actually used. Because I think in terms of a computer, having the nuance to make some of the distinctions based upon the data that it has, I don’t know that computers are there yet. So even for like, my son who has more privilege than he knows what to do with, we tell him when he goes out, “Don’t wear a hoodie,” you know. “Don’t do this, don’t do that,” because a computer isn’t going to say you’re a black boy of privilege. It’s going to you’re a black boy who’s six two who looks like this. Right?
Cassell: Stereotype.
Audience 1: So there’s a lot of nuance that needs to go behind the data. And I tell him you know, “You’re going into a world where the data might be used in a police situation, right. And the computer’s going to make a very quick decision because the computer didn’t go to school with a guy like you. The computer’s not making that nuanced decision.” And that’s something that I think that we need to work on, and that needs to be part of the discussion.
The second thing I would just say is you know, when we look and say we need to have more people of color, more women, more diversity in the programmers and the people who are doing these algorithms so that they’re thinking about these other people and the distinctions, that’s a long-term play. And I’d be interested to know what are the things that we need to do now to start to make those corrections?
Cassell: Love that question. So, CMU this year attained 50% of the entering class in computer science as women. [Buolamwini snaps fingers] Yeah. And there’s this beautiful mural… And it’s only in the women’s room and I’ve been talking to them about this. But it says “Computer science: an old boys club? Not at Carnegie Mellon.” And I love that. It took us…years. Not a very very long period of time, but a few years to accomplish that. But we accomplished it by investing in the pipeline young. In going into grade schools, and middle schools, and high schools, and working with children, insuring— Jane Margolis has written a beautiful book called Stuck at the Shallow End about race and computing. And she’s looked at the LA school systems. There is no AP computer science in inner city schools in Los Angeles. Or there wasn’t till she arrived. She had done the same thing with women before she wrote Stuck at the Shallow End. She wrote Unlocking the Clubhouse.
So there are things we can do. Yes, we’re starting now with kids who are 7 or 8 and so it’s not going to be right away that we’re going to hire. But, I want to point out that we make a mistake, we make a terrible mistake that backfires, when we think about hiring diverse people.
So, I once had a job as a faculty member at a high-status university. And the head of my department came up to me and the one black faculty member on the faculty and said, “I give the two of you six months to diversify the faculty.” Yeah. Okay. Now, it happened to be a topic that I had been looking at for twenty-three years. Not my colleague; it wasn’t his topic of interest, and certainly we couldn’t do anything in six months.
I was offered a position as diversity officer at another university. And I said no, despite the fact that this is something I care very deeply about. Because when I said, “Okay. We need to think about a pronoun policy. We need to have nonbinary bathrooms. Half our buildings are not accessible, we need ramps up right away.” And the provost said to me, “We’re just going to start with women.”
But intersectionality, Kim Crenshaw’s work, tells us that you can’t just work along one axis. You have to consider for example first-generation college attendees. You need to consider the full range of peoples, not only the diverse people (which usually means black or women), but diverse teams because that’s where creativity comes. And the only way this is going to get done, you all know it, is if we show value. And we can show dollars raised. 85% of consumers are women. And so few women are product designers or marketers or engineers. And we could sell more—our creativity will go up, our innovation will go up, if we hire diverse teens. And if we work in collaborative teens.
Buolamwini: On the point of representation. I’m a computer scientist, right. But I don’t fit the many stereotypes that you listed off in your original talk. And I think the representation of what it looks like to be a computer scientist— I’m also an athlete, pole vaulter, all of these other things. But inviting people in who don’t necessarily look like the stereotype and saying, “You too matter. What you’re doing is valuable. And also your perspectives matter.” The paper I wrote, Gender Shades, I did it in collaboration with Dr. Timnit Gebru who was at Stanford. And she is from Ethiopia. It’s no surprise that as dark-skinned women we found the problems that we did. So it’s also being able to elevate the work that is there as well.
Lichfield: Unfortunately that is all the time that we have. So, Joy and Justine thank you so much for being here.