Jillian C. York: Hello. So when we thought about this theme… We were both at re:publica in Dublin last year. We got to see the announcement of this them of re:publica, and the first thought that we had was how can you love out loud in a time of ubiquitous surveillance? And so as we’ve created this talk we’ve set out to answer that question. I am going to say I’m not representing my organization today, I’m just speaking from my personal perspective. And I think yeah, let’s take it away.
Mathana: We have a lot of content we’re going to cover quickly. If anybody wants to discuss things afterwards, we’re more than happy to talk. We built kind of a long presentation so we’re going to try to fly through, be as informative and deliberate as possible.
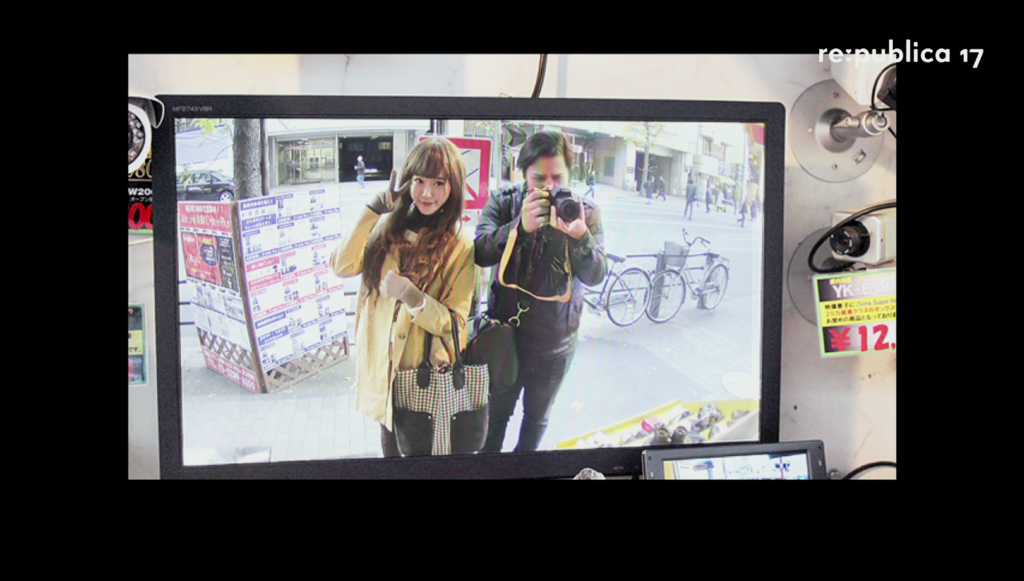
So one of the things that we wanted to discuss is the way in which facial recognition technologies are surrounding us more and more. It’s a number of things. One, retrofitting of old, existing systems like CCTV systems in subways and other transportation systems. But as well as new and miniaturized camera systems that are now propagated to a backend of machine learning neural networks and new AI technologies.
York: There’s a combination of data right now. Data that we’re handing over voluntarily to the social networks that we take part in, to the different structures in which we participate, be it medical records, anything across that spectrum. And then there’s data, or capture, that’s being taken from us without our consent.
Mathana: So our actions captured on film paint a startlingly complete picture of our lives. To give you an example, in 2015 the New York metro system, the MTA, started installing around 1,000 new video cameras. And we think about what this looks like on a daily basis. The things that can be gleaned from this. Transportation patterns. What stations you get on and off at. If you are able to be uniquely identified by something like a metro CCTV integrated system, even when you wake up; the stops that you go to; if you are going to a new stop that you may not normally go to. That we’re actually even in this one system, a startlingly clear picture starts to emerge of our lives in the passive actions that we take on a daily basis, that we’re not even really aware that are being surveilled, but they are.
York: And then when that data’s combined with all of the other data, both that we’re handing over and that’s being captured about us, it all comes together to create this picture that’s both distorted but also comprehensive in a way.
So I think that we have to ask who’s creating this technology and who benefits from it. Who should have the right to collect and use information about our faces and our bodies? What are the mechanisms of control? We have government control on the one hand, capitalism on the other hand, and this murky grey zone between who’s building the technology, who’s capturing, and who’s benefiting from it.
Mathana: This is going to be one of the focuses of our talk today, kind of this interplay between government-driven technology and corporate-driven technology—capitalism-driven technology. And one of the kind of interesting crossovers to bring up now is the poaching of universities and other public research institutions into the private sector. Carnegie Mellon had around thirty of its top self-driving car engineers poached by Uber to start their AI department. And we’re seeing this more and more, in which this knowledge capacity from the university and resource field is being captured by the corporate sector. And so when the new advances in technology happen it’s really for profit companies that are the ones that are kind of the tip of the spear now.
York: And one of those things that they do is they suck us in with all of the cool features. So just raise your hand real quick if you’ve ever participated in some sort of web site or app or meme that asked you to hand over your photograph and in exchange get some sort of insight into who you are. I know there’s one going around right now where it kind of changes the gender appearance of a person. Has anyone participated in these? Okay, excellent. I have, too. I’m guilty.
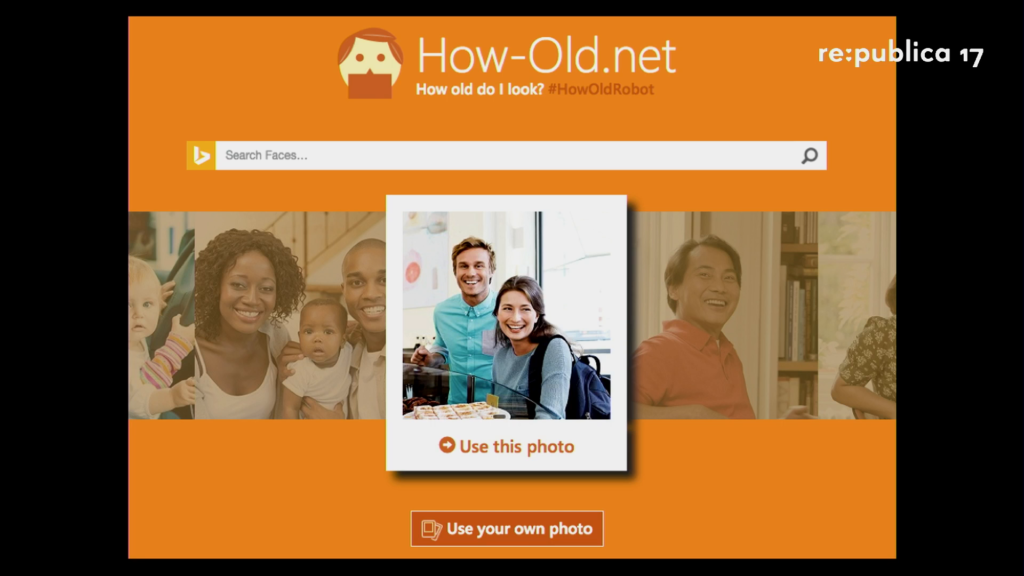
Does anyone remember this one? So this was this fun little tool for endpoint users, for us to interact with this cool feature. And basically what happened was that Microsoft, about two years ago they unveiled this experiment in machine learning. It was a web site that could guess your age and your gender based on a photograph.
He thought that this was kind of silly to include, like this isn’t a very common example. But I was actually reminded of it on Facebook a couple days ago, when it said oh, two years ago today this is what you were doing. And what I was doing with this, and what it was telling me was that my 25 year-old self was like 40 years old.
So it wasn’t particularly accurate technology but nevertheless the creators of this particular demonstration had been hoping “optimistically” to lure in fifty or so people from the public to test their product. But instead within hours the site was almost struggling to stay online because it was so popular, with thousands of visitors from around the world and particularly Turkey for some reason.
There was another website called Face My Age that launched more recently. It doesn’t try to just guess at age and gender, but it also asks users to supply information like their age, like their gender, but also other things like their marital status, their educational background, whether they’re a smoker or not. And then to upload photos of their face with no makeup on, unsmiling, so that they can try to basically create a database that would help machines to be able to guess your age even better.
And so they say that okay well, smoking for example ages people’s faces. So we need to have that data so that our machines can get better and better learning this, and then better and better at guessing. And of course because this is presented as a fun experiment for people, they willingly upload their information without thinking about the ways in which that technology may or may not be eventually used.
So Matthew, I’m going to hand it over to you for this next example because it makes me so angry that I need to cry in a corner for a minute.
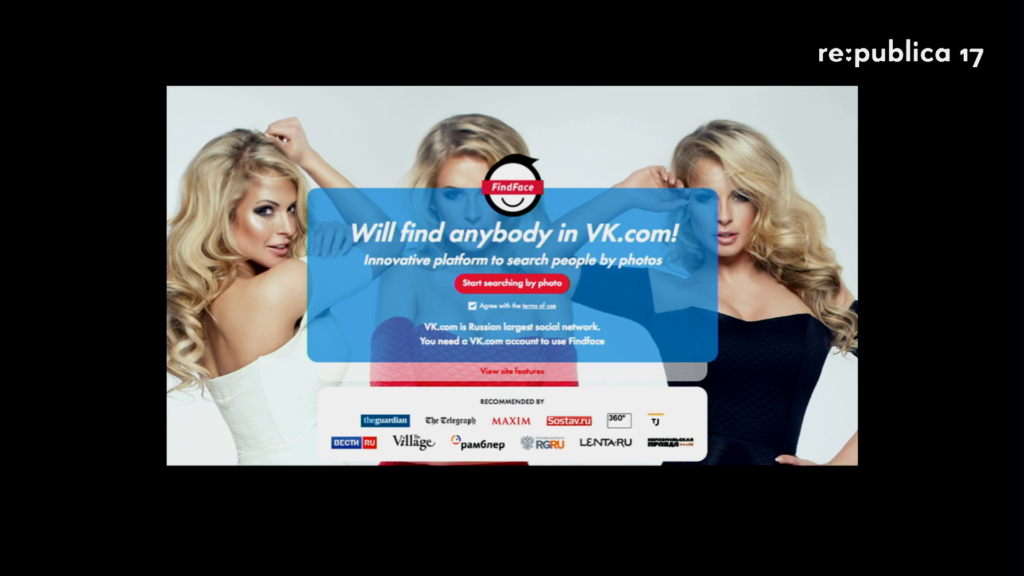
Mathana: So, FindFace. VKontakte, VK, is the Russian kinda Facebook clone. It’s a large platform with hundreds of millions of users—
York: 410 million, I think.
Mathana: What someone did was basically— How’d it go? The story goes they got access to the photo API. So they had kinda the firehose API. They were able to have access to all the photos on on this rather large social media platform. Two engineers from Moscow/St. Petersburg wrote a facial recognition script that basically to date is one of the top facial recognition programs. And they were basically able to connect this on the frontend, which is an app used by users, to be able to query the entire VK photo database and in a matter of seconds return results. So it gives you as a user the power to take a photo of somebody on the street, query against the entire social media photo database, and get matches that are either the match of a person or you can find people that look similar to the person that you’re trying to identify.
York: So NTechLab, which builds this, won the MegaFace Benchmark (love that name), which is the world championship in face recognition organized by the University of Washington. Do you see the interplay already happening between academia and corporations? The challenge was to recognize the largest number of people in this database of more than a million photos. And with their accuracy rate of 73.3%, they bypassed more than a hundred competitors, including Google.
Now, here’s the thing about FindFace. It also as Matthew pointed out looks for “similar” people. So this is one of FindFace’s founders (that is a mouthful of words), Alexander Kabakov. And he said that you could just upload a photo of a movie star you like, or your ex, and then find ten girls who look similar to her and send the messages. I’m…really not okay with this.
And in fact I’ve got to go back a slide to tell you this other story, which made me even more sad, which is that a couple years ago an artist called Igor Tsvetkov highlighted how invasive this technology could be. He went through St. Petersburg and he photographed random passengers on the subway and then matched the pictures to the individuals VKontakte pages using FindFace. So, “In theory,” he said, “the service could be used by a serial killer or collector trying to hunt down a debtor.”
Well he was not wrong, because what happened was that after his experiment went live and the media covered it—there was a lot of coverage of it in the media as an art project—another group launched a campaign to basically demonize pornography actors in Russia by using this to identify them from their porn and then harass them. And so this has already been used in this kind of way that we’re pointing out as a potential. This is already happening. Stalkertech.
But you know, I think that one of the really interesting thing that Matthew found is that as we were looking through these different companies that create facial analysis technology and emotional analysis technology, the way that they’re branded and marketed is really interesting.
Mathana: We can go through some of these examples, even just slide to slide and see. These are some of the top facial recognition technology companies out there. And what’s interesting, we’re not going to be talking so much about Google, about Facebook, about Amazon, although these companies are important. But we’re here highlighting one of the kind of understated facts in the facial recognition world, is that there are small companies popping up that’re building incredibly powerful and sophisticated algorithms to be able to find facial recognition matches, even in low quality, low light, rerendering, and converting from 2D to 3D. These sort of things whose names we don’t know. And we can sit back and kind of demonize the large technology companies, but there is a lot to be done to hold small companies accountable.
York: And I think you can see the familiar thread through all of these, which is what? Anyone want to wager a guess? Smiling happy faces. Usually beautiful women, smiling and happy, as we saw back on VKontakte’s page.
So, rather than focusing on the bad guys, they’re focused on this “Oh look! Everyone’s really happy when we use these facial recognition technologies.” But what a lot of these technologies are doing is in my opinion dangerous. So for example Kairos, which is a Miami-based facial recognition technology company, they also own an emotional analysis technology company that they acquired a couple of years ago and they they wrapped into their core services.
And their CEO has said that the impetus for that came from their customers. Some of their customers are banks. Specifically that a bank teller, when you go to the bank, maybe a bank teller could use facial recognition technology to identify you. And that would be a better way than you showing your ID or signing something or maybe even entering a PIN.
But, sometimes you could have someone maybe that day who comes in to rob the bank and their face is kind of showing it. And so with that emotional analysis technology, the bank teller could have it indicated to them that today is a day that they will refuse you service.
But my immediate thought when I read that was what about people who live with anxiety? What about people who are just in a hurry that day? So you could literally be shut out of your own money because some algorithm says that you’re anxious or you’re too emotional that day to be able to do that.
Another example that I found really troubling was this one, Cognitec, which is I think a Dutch company. Theirs does “gender detection.” So this is used by casinos in Macau as well as in other places. I thought gender detection was a really funny concept, because as our society’s become more enlightened about gender and the fact that gender is not always a binary thing, these technologies are basically using your facial features to place you in a gender.
And I’ve tested some of these things before online where it tries to guess your gender, and it often gets them wrong. But in this case it’s actually where does our gender autonomy even fit into this? Do we have gender autonomy if these systems are trying to place us as one thing or another?
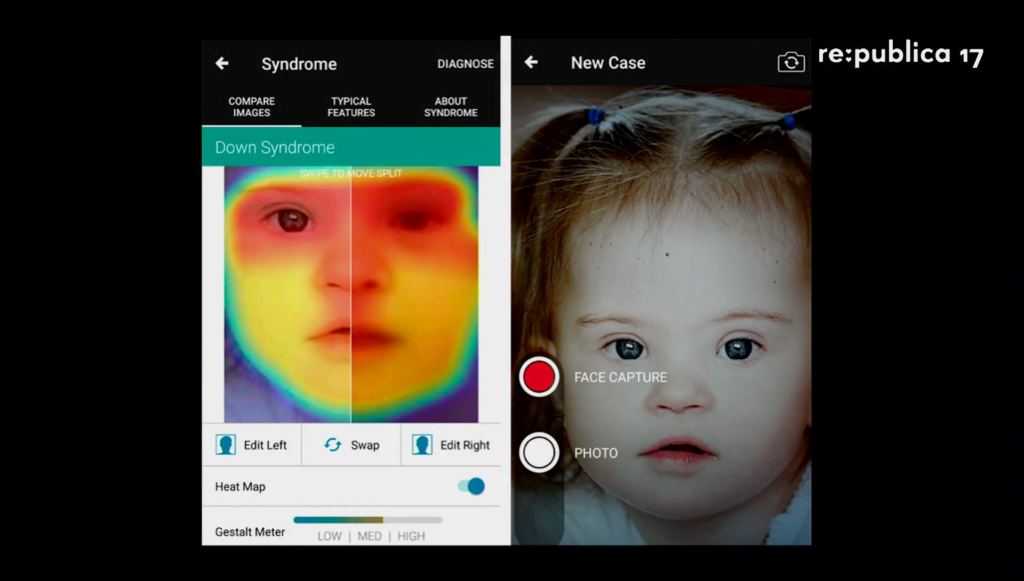
Mathana: So one of things that is really quite startling about this is the culmination of data points. When we’re talking about the way in which young people are having their faces scanned earlier, in which identification software is being used using facial recognition technology, that no one action may be held in a static kind container anymore. That we’re now dealing with dynamic datasets that are continuously being built around us.
Algorithms learn by being fed certain images, often chosen by engineers, and the system builds a model of the world based on those images. If a system is trained on photos of people who are overwhelmingly white, it will have a harder time recognizing nonwhite faces.
Kate Crawford, Artificial Intelligence’s White Guy Problem, New York Times [presentation slide]
And one of the issues is that there is an asymmetry of the way in which we’re seen by these different systems has, as Kate Crawford has said— (She presented last year on stage one. Some of y’all may have caught that.) And a lot of her research has gone into kind of the engineering side. Looking at the ways in which discrimination bias replicates inside of new technologies. And as facial recognition technologies are just one vector these discriminatory algorithms, it’s more and more important that we take a step back and say well, what are the necessary requirements and protections and safeguards to make sure that we don’t end up with with things like this:
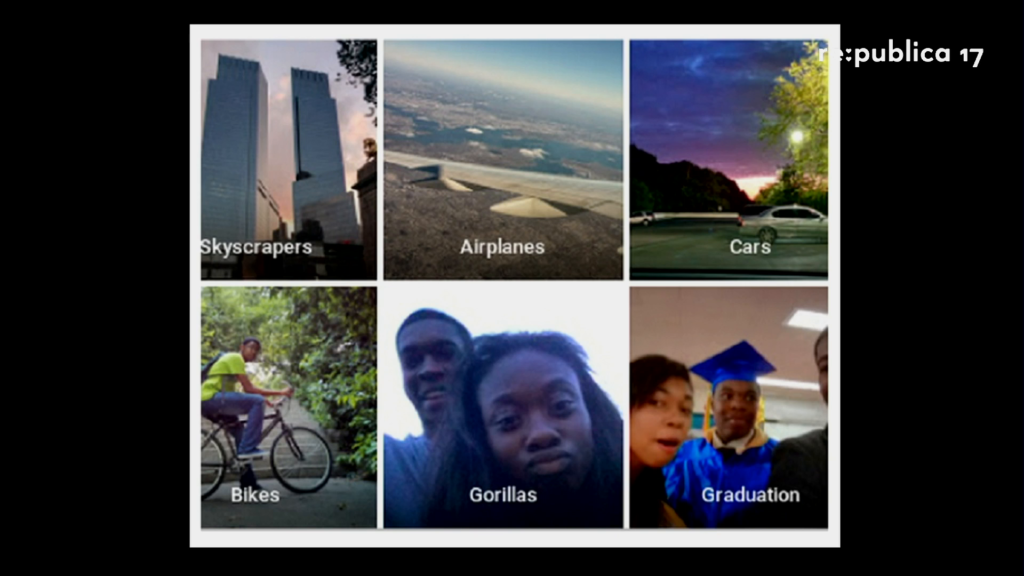
With a Google algorithm saying that a black couple are gorillas. And there’s a lot of other famous examples of this. But if engineering teams and if companies are not thinking about this holistically from the inside, then there may PR disasters like this, but the real-world implications of these technologies are very unsettling and quite scary.
York: Now, Google was made aware of this and they apologized. And they said that “there is still clearly a lot of work to do with automatic image labeling, and we’re looking at how we can prevent these types of mistakes from happening in the future.” And they have done great work on this but I still think that part of the problem is that these companies are not very diverse. And that’s not what this talks about but I can’t help but say it. It’s an important facet of why these companies continue to make the same mistakes over and over again.
But let’s talk a little bit about what happens when it’s not just a company making a mistake in identifying people in an offensive way, but when the mistake has real-world implications.
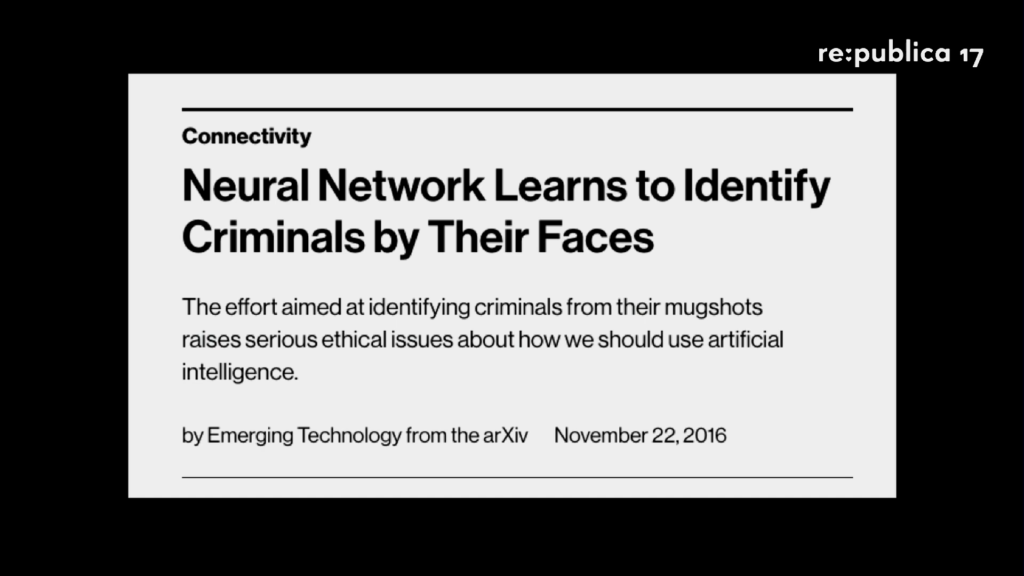
Mathana: So, one of things that is really quite startling-interesting— And we were talking about some of the different algorithms for VK. But here is another example, that now scientists around the world and technologists are training facial recognition algorithms on databases. In this case a group of innocent people, a group of “guilty people,” and using machine learning and neural networks to try to discern who is guilty out of the test data and who is [innocent].
York: Incidentally, their accuracy rate on this particular task was 89.5%.
Mathana: So 89.5%. I mean, for some things almost 90%…not bad. But we’re talking about a 10% rate of either false positives or of just errors. And if we’re thinking about a criminal justice system in which one out of every ten people are sentenced incorrectly, we’re talking about a whole tenth of the population which at a time in the future may not be able to have access to due process because of automated sentencing guidelines and other things.
And it’s happening now. Nearly one half of American citizens have their face in a database that’s accessible by some level of law enforcement. And that’s massive. That means that one out of every two adults in the US, their face has been taken from them. That their likeness now resides in a database which is able to be used for criminal justice investigations and other things. And so you may not even know that your face is in one of a number of different databases, and yet on a daily basis these databases maybe crawled to look for new matches for guilty people or suspects. But we’re not aware of this a lot of times.
York: And it’s not just our faces, it’s also other identifying markers about us. It’s our tattoos, which I’m covered with which and which now I know— I didn’t know when I got them, but now I know that that’s a way that I can be identified by police so I’m going to have to come up with some sort of thing that covers them with infrared—I don’t even know.
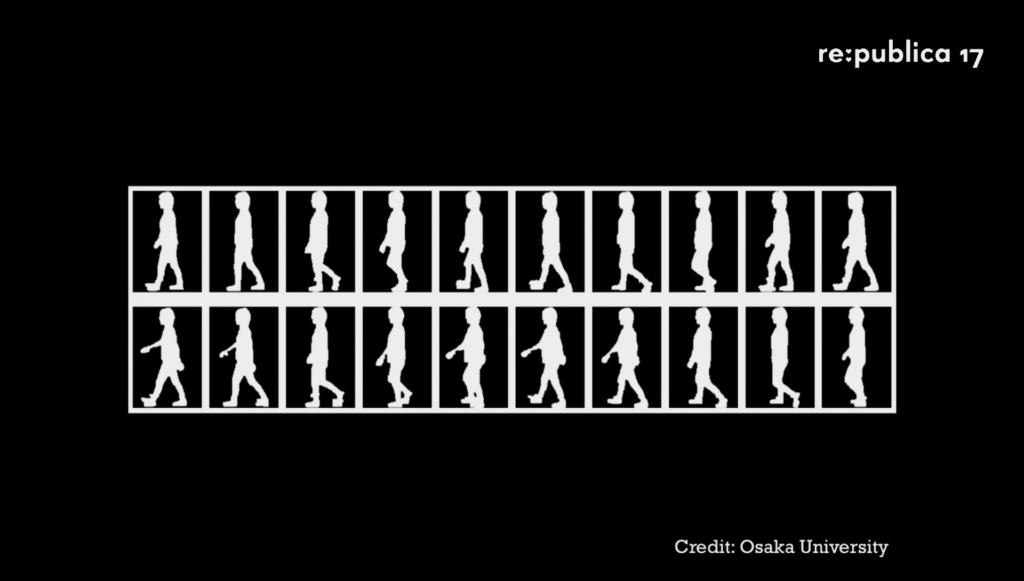
Image: OU-ISIR Biometric Database
It’s also our gait. It’s the way that we walk. And one of the really scary things about this is that while facial recognition usually requires high-quality images, gait recognition does not. If you’re walking on the subway platform and the CCTV camera picks you up—and you know that the U‑Bahn stations are covered with them—if you’re walking that way, a low-bandwidth image is enough to recognize you by your gait. Yesterday, my boss from across the room recognized me by my gait. Even our eyes can do this. So it’s possible.
The machines have eyes, and in some ways minds. And as more of these systems become automated, we believe that humans will be increasingly place outside of the loop.
Mathana: So just another example, going back to New York, to show how these things really don’t just… We’re now in this interconnected world. I don’t know if you all are familiar with stop-and-frisk. It was an unpopular law in New York City which allowed police officers to essentially go up to anyone they might be suspicious about and ask them for ID and pat them down.
What happened, why this was eventually pulled by the police department, there were some challenges in court saying it was unconstitutional. The policy was was rescinded before it went to court. But the idea of…there are now records from the time that was in place of the people who were charged under this policy. It was found to be very discriminatory in the sense that young men of color were disproportionately targeted by this program.
So if we’re looking at crime statistics, let’s just say a readout of the number of arrests in New York City. And if we were to put demographic data with that and then feed this into a machine learning algorithm, if the machine learning algorithms sees that a large percentage of individuals are young black and brown men, what is the machine learning algorithm to think except that these individuals have a higher likelihood of committing crimes?
In the real world, it was real-world bias by police officers that were targeting minority communities. But a machine algorithm…if we’re not weighting this sort of information in the test and training data, there’s no way for a machine to logically or intuitively see a causal relationship between segregation and bias in the real world, and the crime statistics that are the result from that.
York: So with that in mind, we want to talk a little bit about how we can love out loud in a future where ubiquitous capture is even bigger than it is now. So Matthew, tell me a little bit about this example from Sesame Credit. Has anyone heard of Sesame credit? Okay, so we’ve got a few people who are familiar with it.
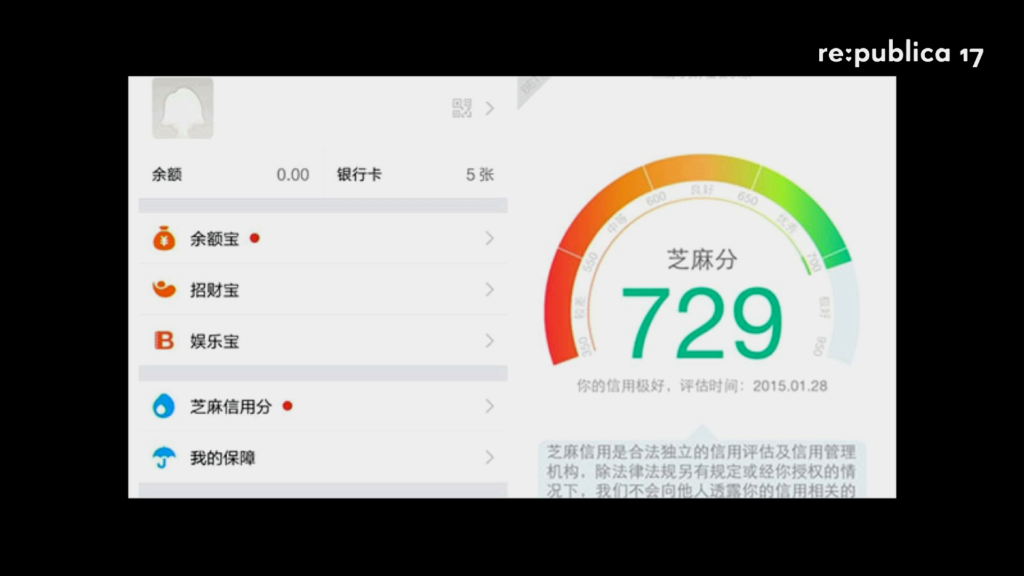
Mathana: So Sesame Credit is a system that’s now been implemented in China—there’s an ongoing rollout. It’s a social credit rating, essentially. It uses a number of different factors. It’s being pioneered by Ant Financial, which is a large financial institution in China. It’s a company, it’s not technically a state-owned enterprise, but it’s fuzzy when it comes to the ruling Chinese Communist Party.
The interesting thing about this new system is that it uses things like your… It’ll look into things like your WeChat account and see who you are talking with. And if people are discussing sensitive things, your social credit rating can be docked.
So now we’re seeing this system developed right now in China that brings in elements from your social life, your network connections, as well as things like your credit history, to paint a picture of how good of a citizen are you. Alipay is now launching the US. I think it was announced today. And so they’re now pioneering some very sophisticated technology like iris scans. And the contactless market in China has exploded from a few hundred millions of dollars now into the hundreds of billions of dollars. And so this technology in the last even twenty-four months has become much more prevalent and has become much more ubiquitous for its capacity for individual surveillance.
York: And this is where life begins to resemble an episode of Black Mirror. So what we’d like to remind you is that digital images aren’t static. With each new development, each sweep of an algorithm, each time you put something there you’ve left it there. I know that I’ve got hundreds, possibly thousands of images sitting on Flickr. With each new sweep of an algorithm, these images are being reassessed. They’re being reconsidered and reidealized to match other data that this company or X Company or a government might have on you. What you share today may mean something else tomorrow.
So right now we feel that there’s no universal reasonable expectation that exists between ourselves and our technology. The consequence of data aggregation is that increased capture of our personal information results in this more robust, yet distorted, picture of who we are that we mentioned at the beginning.
And so I think that we’ll take the last few minutes, and we’ll try to leave a few minutes for questions, just to talk about that emerging social contract that we would like to see exist, we would like to see forged between us and technology companies and governments.
We can’t see behind the curtain. We have no way of knowing how the collection of our visual imagery is even being used, aggregated, or repurposed. And we want to remind you also that these technologies are mechanisms of control. And so the first question that I want to ask, personally, is what kind of world do we want? I think that’s the starting point, is asking do we want a world where our faces are captured all the time? Where I can walk down this hallway and have different cameras that are attached to different companies that have different methods and modes of analysis looking at me and trying to decide who I am and making determinations about me.
But perhaps we’re past that point, and so we’ve decided to be pragmatic a little bit in trying to formulate some things that we can do. So in terms of what we want, we want active life that’s free from passive surveillance. We want more control over our choices and over the images that we share. And we want a technology market that isn’t based on selling us out to the highest bidder. Luckily there are some people working on all of these things right now, not just us, and so we feel really supported in these choices. And we’ll turn to you for regulation.
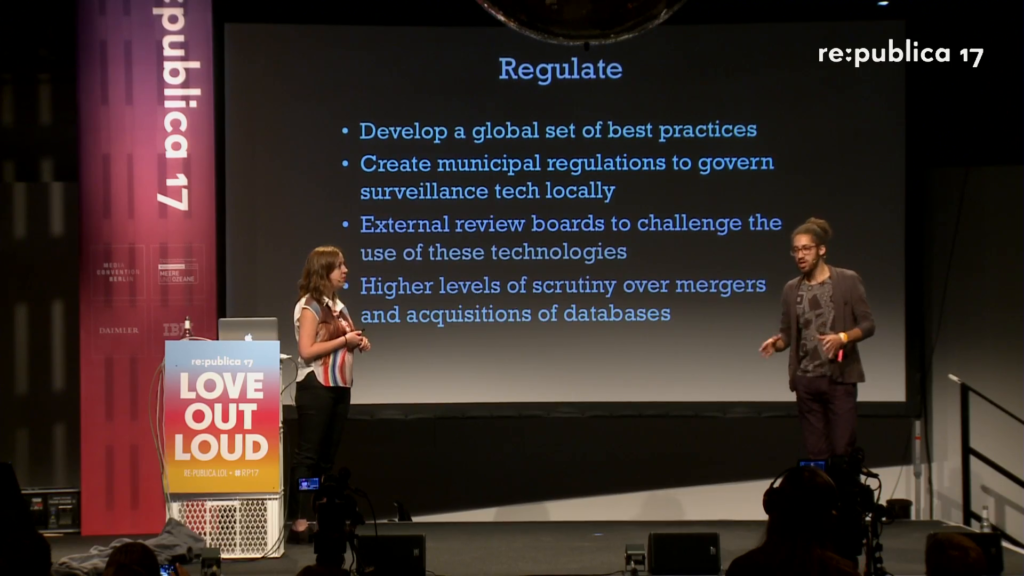
Mathana: I’ve had the opportunity to sit in on a couple of smart cities sessions yesterday, talking between development of smart cities in Barcelona and Berlin, as well as smart devices yesterday. We are I think seeing to some degree a development of a global set of best practices, but very piecemeal and fragmented. And I think that if we think about image recognition technology as kind of a layer that fits on the many of the other modes of technological development, that it becomes clear that actually we need to have some sort of best practices as biometric databases continue to be aggregated.
It’s very difficult for one person in one country and a different person in a different country to have reasonable expectations of what the best practices are going forward. I mean, maybe today it’s possible but twenty years from now what’s the world look like that we want to live in?
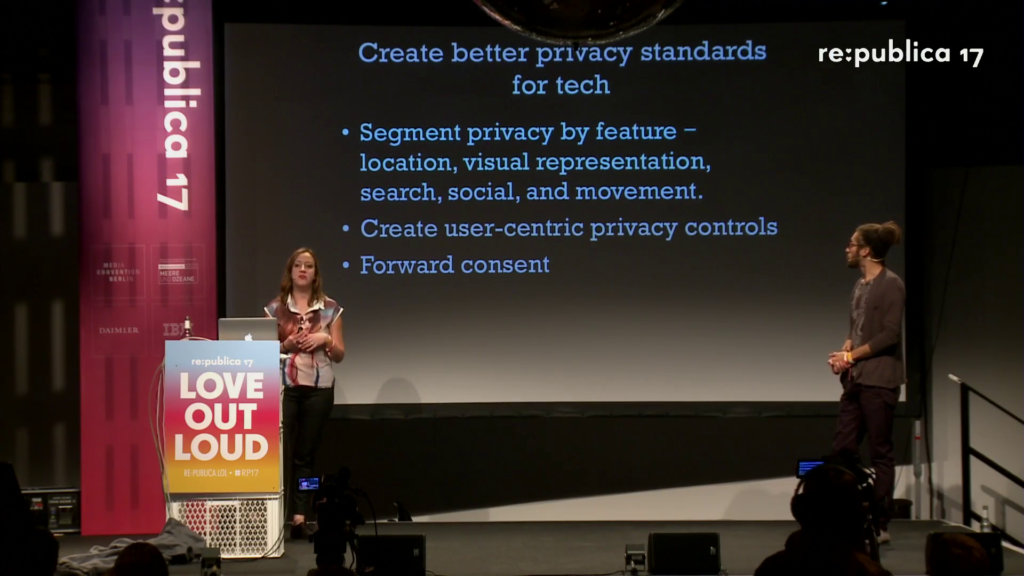
York: So these are some of the areas that we think governments, and particularly local governments, can intervene. We also think that we can have better privacy standards for technology. And we know that there are a lot of privacy advocates here and that those things are already being worked on, too. So we want to acknowledge the great work of all the organizations that are fighting for this. But we see one way to this is segment privacy by feature—location, visual representation, search, social, and movement, all of these different areas in which our privacy is being violated.
We also think user-centric privacy controls… Right now, most privacy controls on different platforms that you use are not really user-friendly. And trust me, I spend a lot of time on these platforms.
And then another thing, too, that’s really important to me—and the rest of my life I work on censorship—but I think forward consent. I don’t feel that I am consenting to these fifteen-page terms and conditions documents that companies try to make as confusing for me as possible. And so I think that if companies keep in mind forward consent every time that you use their service, that’s one way that they can manage this problem.
But also, we think that you have to keep loving out loud. That you can’t hide. That you can’t live in fear. That just because these systems are out there, yes of course we have to take precautions. I talk a lot in my day job about digital security. And I think that this is that same area, where we have to continue living the way that we want to live. We can take precautions, but we can’t sacrifice our lives out of fear.
And so one thing is photo awareness. We’re really glad to see that at a lot of conferences recently there’ve been ways of identifying yourself if you don’t want to be photographed. But also in clubs in Berlin if anyone’s ever gone clubbing (and if you haven’t you probably should), usually they put a sticker over your camera when you walk in the front door. And that to me… The first time I saw that I was so elated that I think I danced til 7AM. I mean, that’s not normal.
We also think that you know, regulate your own spaces. I don’t want to get into this division of public/private property. That’s not what I’m here for. But I think that we have our own spaces and we decide inside of those what’s acceptable and what isn’t. And that includes conferences like this. So if we feel in these spaces—I don’t really know what’s going on with cameras here, if they exist or not. But if we feel in these spaces that that’s something we want to take control of, then we should band together and do that.
Put static in the system? [Gestures to Mathana.]
Mathana: This is a general point but in any system that is being focused on, how can we find ways to put static in the system, whether this is tagging a photo that’s not you with your name on Facebook, or whether that’s… Yeah, these sort of strategies, right. So it’s ways in which, how can we think a little more outside to actually confuse the algorithms or to make their jobs a little more difficult. And there’s different ways to do this, whether it’s wearing reflective clothing, anti-paparazzi clothing in public, or tagging things under one label that may not be that.
York: It also means you know, wearing those flash-proof garments, covering your face, going to buy your burner phone in the store and wearing a Halloween costume. I’m not saying you should do that [whispering] but you should totally do that.
And continuing to love out loud and not live in fear. I can see that we’ve completely run out of time because I think the schedule’s a little bit behind. But there’s some good news. If you want to keep talking to us about this, we’re both pretty easily accessible. But we’re also going to take advantage of that sunlight that didn’t exist yesterday and go out back for a celebratory beer. So if you want to keep talking about this subject you’re welcome to join us out there. Thank you so much.