David Cox: Quick audience participation. How many of you—raise your hands if you have one of these.
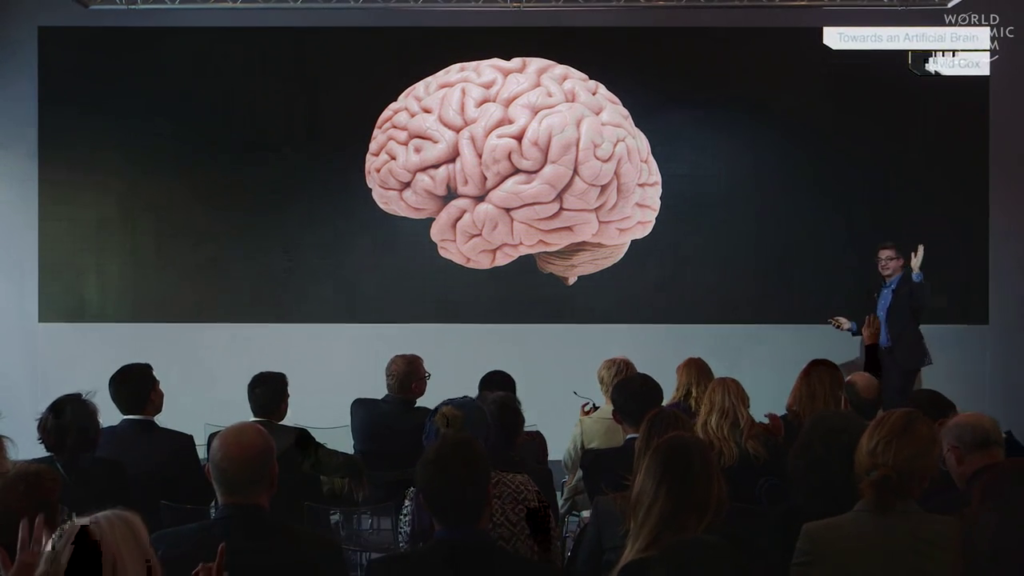
Keep your hands up if you’re quite attached to it. Good. I’m attached to mine as well. So, usually when we think about brains, we think about the naturally-occurring kind. But what we’re gonna talk about today is the prospect of building artificial brains. And the only reason we can really have this conversation today is because there are two fields that are exploding right now and are on a collision course with one another. On one hand we have neuroscience, the study of the brain. And the other side, we have technology. And in particular computer science.
Now, it might seem weird to sort of connect technology and neuroscience. But it’s actually something we’ve been doing for a very long time. So if we look through history, we’ve always looked at the mind and the brain through the lens of the current technology of our day.
So in the era of Descartes, hydraulics was high technology. And Descartes imagined that there was an animating fluid that would flow through the body to move the body. So using technology to think about, as a metaphor for our mind.
Move to the era of Freud, steam is the technology of the day. And we start thinking about the mind building up pressure and letting off pressure, and start thinking about stoking the engines of cognition.
Fast-forward to the electronics era and radio. We have crossed wires and being on the same wavelength. Our language follows our technology. And today, hands down, the technology of the day is the computer. From its humble beginnings mid-century of last century, to today where we all carry computers around in our pockets and we slavishly look at them all the time, now computers are the lens through which we see our brains.
And these metaphors can lead us astray and lead us to think the wrong things about the brain. So if you think about the central processing unit being separate from memory, that’s not actually how our brain works. And as appealing as it might be to get a memory upgrade to our brains, that’s just not the way it’s going to happen.
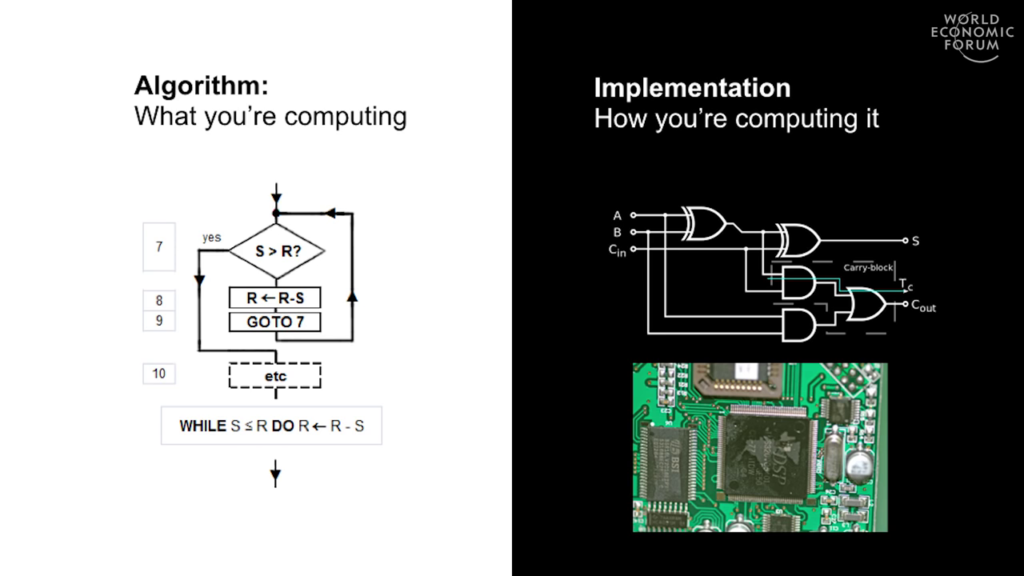
But I would argue that this metaphor is very different. And the reason is that computer science is a field actually of applied mathematics that lets us reason about the algorithm, which is what we’re computing, separately from the implementation, which is the hardware we use to do that computation. And what computer science does is it gives us an equivalence where we can think about different kinds of algorithms running on hardware that maybe wasn’t the original hardware that it ran on. If we understand the algorithms of the brain, can we think about running that other hardware that we have, silicon hardware. And this is a quiet revolution that’s happening right now.
So, there’s something called deep learning, or neural networks. It’s actually quite an old technology, but in the last five years it’s been making incredible strides driven by the availability of incredibly advanced compute and the availability of tons of data. So just five years ago it would’ve been unthinkable that a computer vision system would be able to recognize an object except on a sort of blank background.
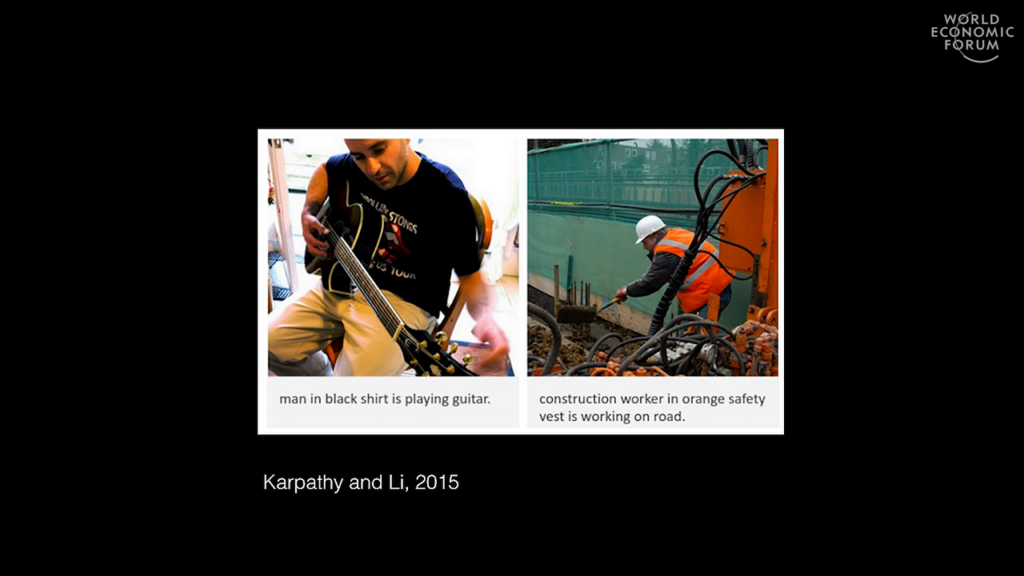
But nowadays, this is a system from Andrej Karpathy and Fei-Fei Li’s lab. Now we can have computers that look at a picture and can actually build a caption. So here, this computer just looked at the picture and generated the caption “Man in in black shirt is playing guitar.” Or “construction worker in orange safety vest is working on road.” So this is amazing what’s happened in just the last five years.
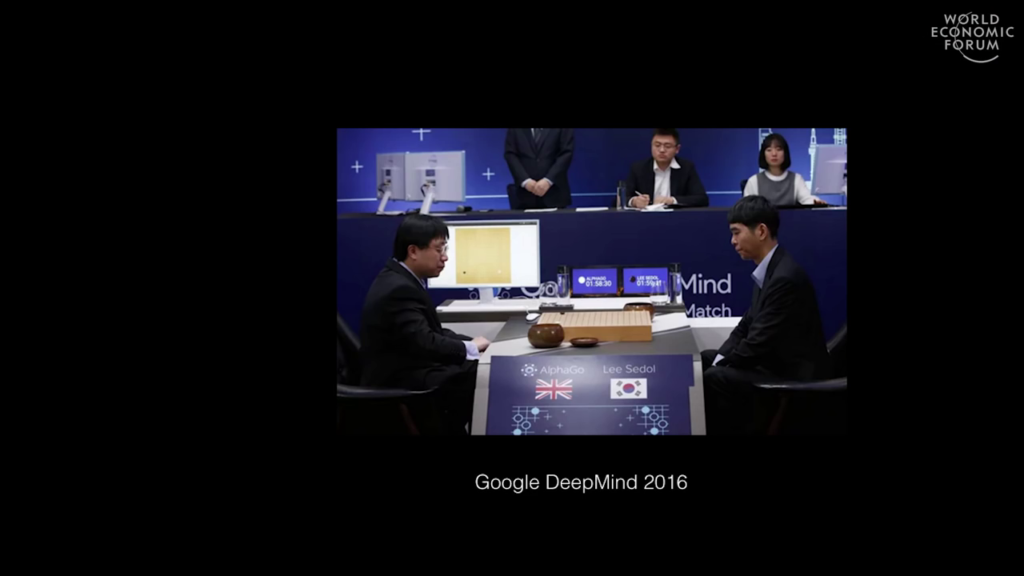
We also have very high-profile things like Google DeepMind’s AlphaGo that beat the world grandmaster in Go. This was basically one of the last games that humans were any good at relative to computers. It was very exciting.
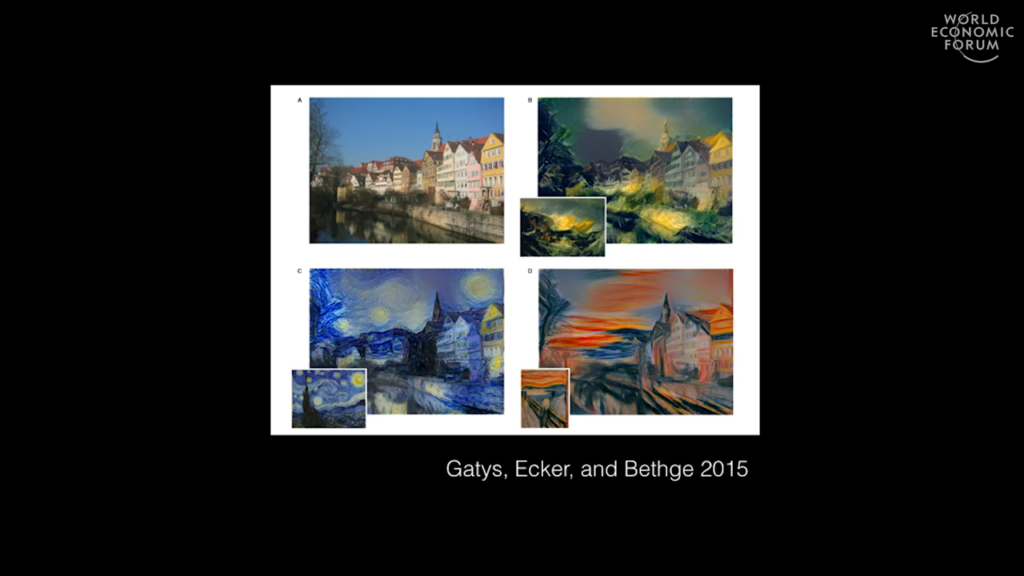
And then we even have computers starting to make art. It’s not all good art but you know, we can start to see that all these domains that we thought as being solely human are now increasingly being encroached upon by machine learning systems.
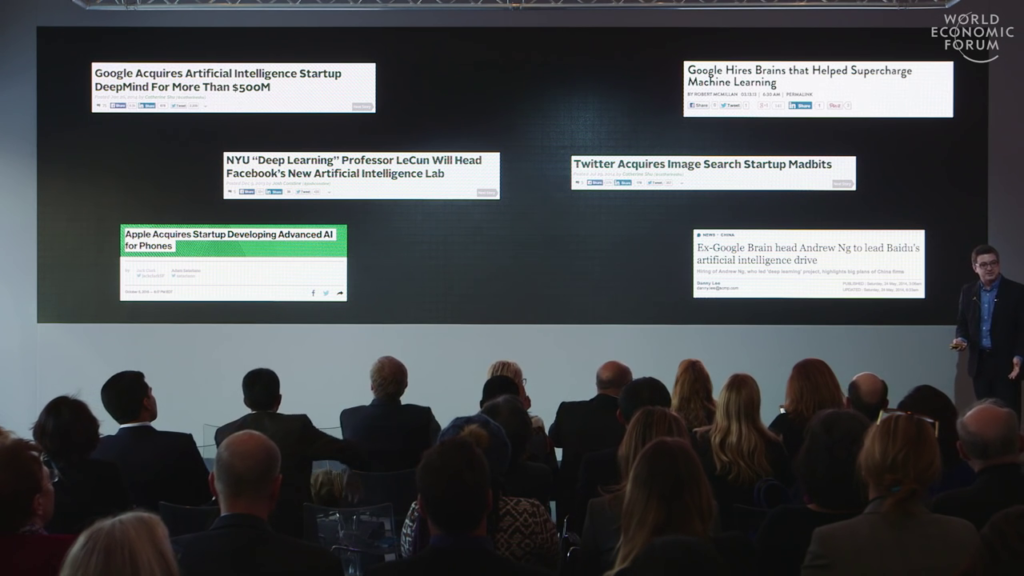
And of course this has caused a tectonic shift in the field. So there’s been massive investment by industry into this field. Billions of dollars from the likes of Google, and Apple, and Baidu. Basically an entire academic field has been privatized and brought in. And sometimes I feel like I’m going be the only person left studying this. And if you’re a representative of one of these companies and you’d like to buy out my lab, we can talk later.
Of course not everyone thinks this is a good idea, or this is a good thing. So Elon Musk is famous for saying that building artificial intelligence is equivalent to “summoning the demon.” Which is awesome when your life’s work is compared to “summoning the demon.” But thankfully there are other people, cooler heads that have commented on this. Like Stephen Hawking saying that artificial intelligence would end mankind.
But I’m here to tell you that we’re not quite there yet. So this is an image from the 2015 DARPA Grand Challenge for robotics. And basically what this is is robots that have to operate in environments that are uneven terrain.
And you know, this is hard. And I’m not knocking any of these robots. These are amazing robots. But a lot of the things we take for granted and we think are simple, are only simple because we have the solution to the problem in our heads. And evolution gave us our brains and that’s what we have.
There are also other examples. So before I showed you these wonderful captions that seem miraculous, that these computers were able to generate. But if you dig a little bit deeper and you choose your images carefully, sometimes you find funny things.
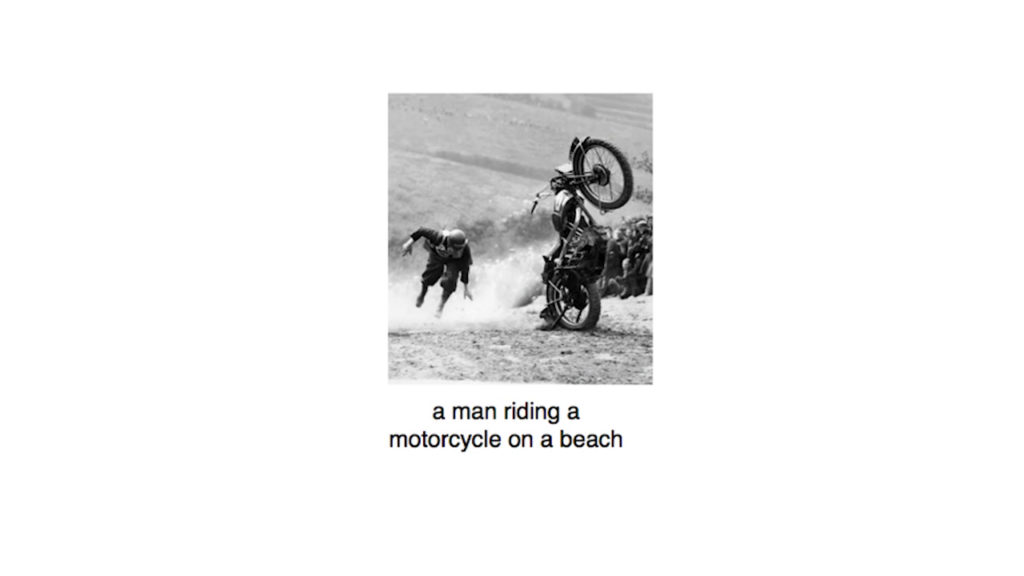
So, this image is “a man riding a motorcycle on a beach,” which sounds like fun.
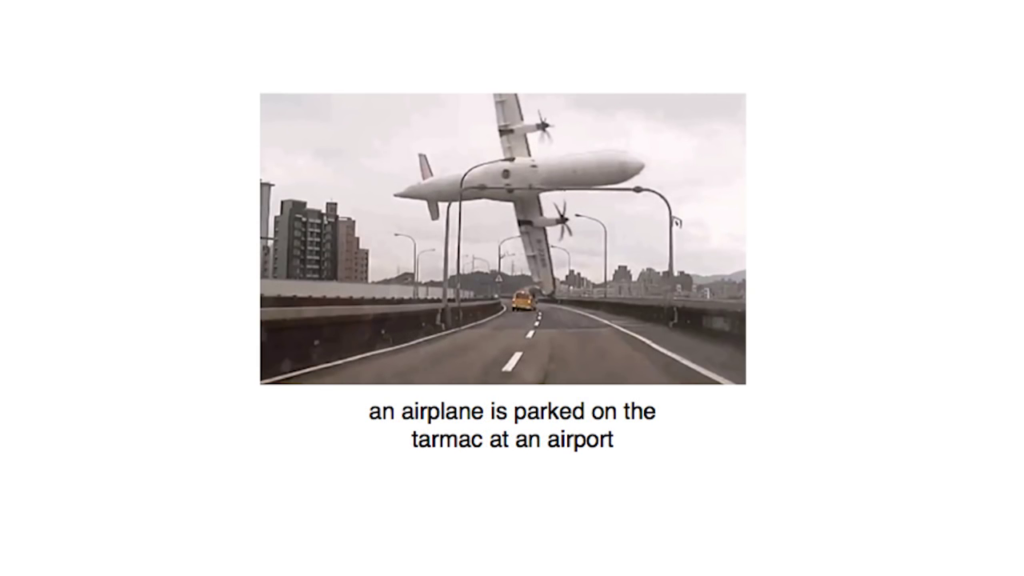
This is “an airplane parked on the tarmac at the airport.” I would say that pilot needs to be fired.
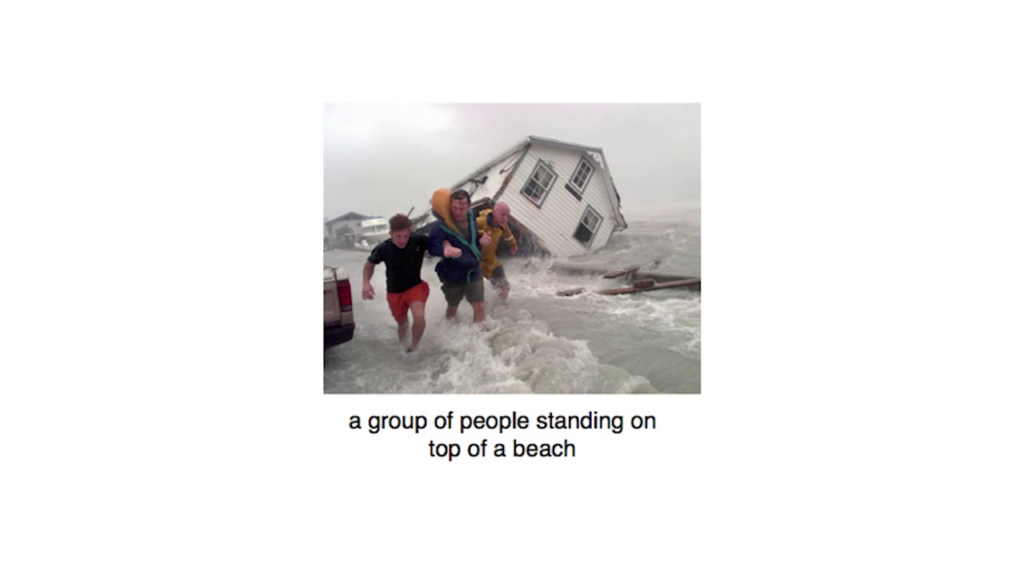
And “a group of people standing on top of a beach,” which sounds like a fun day out on the weekend.
So, there’s a sense in which the systems are truly amazing. And I don’t mean to belittle them. But there’s a sense in which we haven’t gotten the whole story yet, and there’s something still missing. These systems aren’t really understanding the way that we conventionally think about understanding.
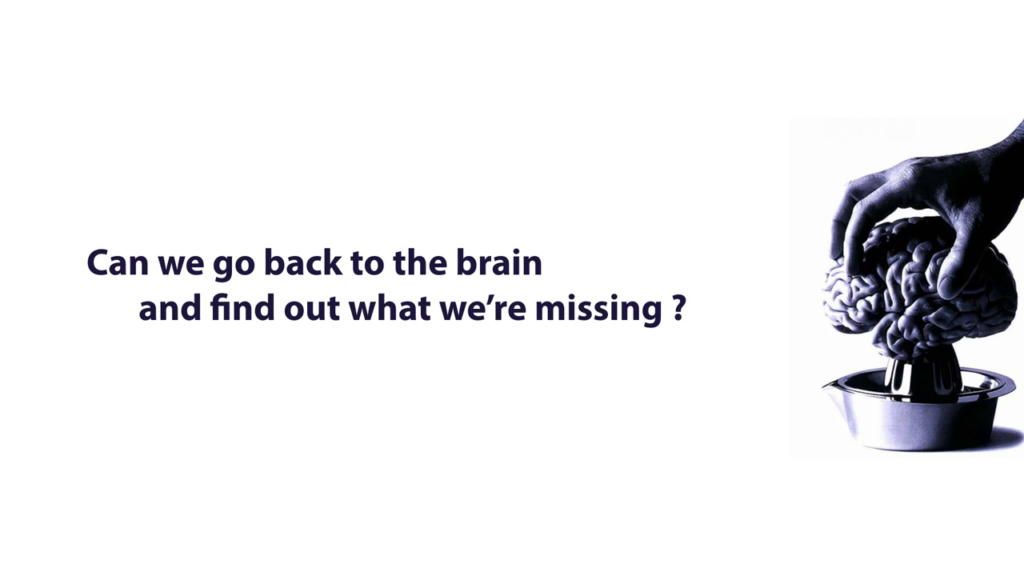
So, what my lab does, and what I’m interested in doing, is going back to the brain to squeeze out some more inspiration. What are we missing from the brain, that we can build into our artificial systems?
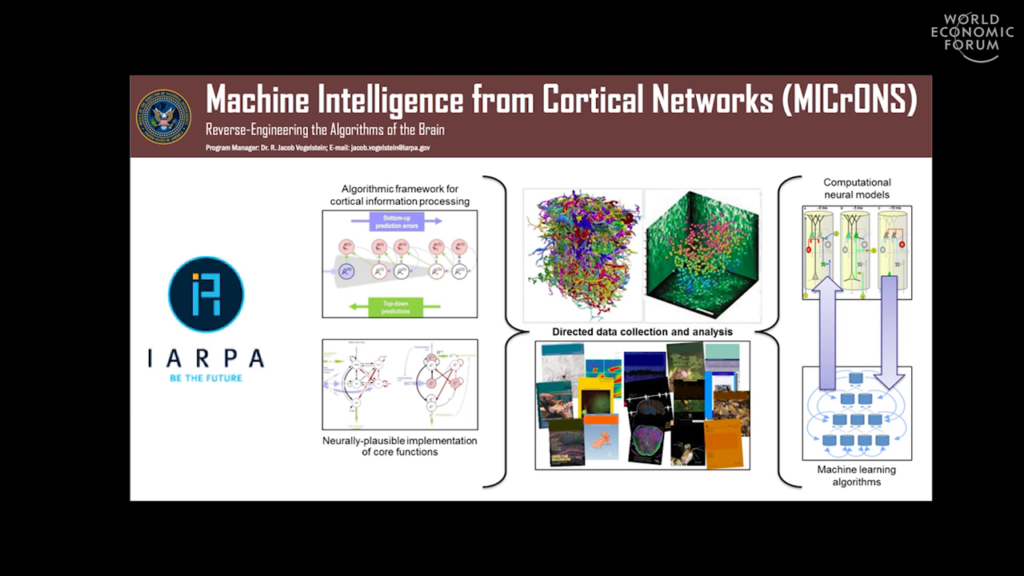
And luckily for me, around 2014 a big fish got interested in the same problem. So IARPA, the Intelligence Advanced Research Projects Activity, which is the high-risk, high-reward arm of the intelligence community of the United States, which is analogous to DARPA is the defense version and they’re famous for funding the creation of the Internet, they started a program that was basically right up my alley. They basically proposed my research program, and a program called Machine Intelligence from Cortical Networks, or MICrONS.
And the goal of MICrONS is threefold. One is they asked us to go and measure the activity in a living brain while an animal actually learns to do something, and watch how that activity changes. Two, to take that brain out and map exhaustively the “wiring diagram” of every neuron connecting to every other neuron in that animal’s brain in the particular region. And then third, to use those two pieces of information, those two experimental datasets, to build better machine learning. To find what deep learning and neural networks today are missing, so that we can close that gap.
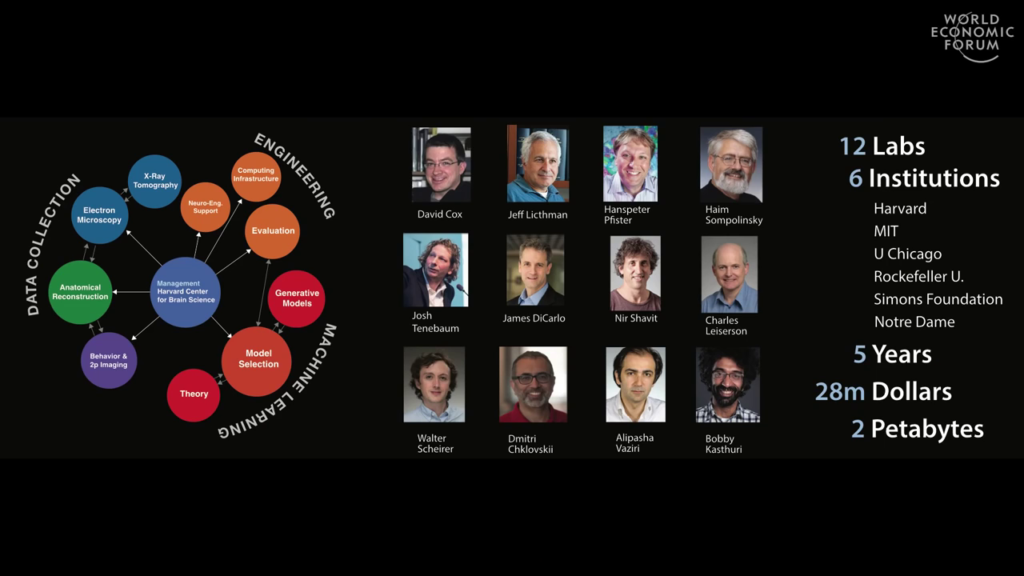
So let it never be said that IARPA is unambitious. This is an incredibly difficult thing they’ve asked us to do, but fortunately I was able to put together a dream ream to work on. This is an enormous undertaking. So we are crossing twelve labs in six institutions, with a heavy concentration of work at Harvard and MIT. We’re gonna work on this for five years, $28.7 million. And by the time we’re done we’ll have collected two petabytes of data, which is one of the largest neuroscience datasets ever collected.
Across this team, we have expertise in neuroscience, and physics, in machine learning, and in high-performance computing. So this is really a moonshot effort, of the ambition sort of scale of the Human Genome Project to really take a real crack at reverse engineering the brain.
So, I’m gonna walk you through a little bit of how this goes. The experiment starts on the second floor of the Northwest Labs, where my lab is. And this is going to be a sort of unusual epic journey that a brain is gonna take.
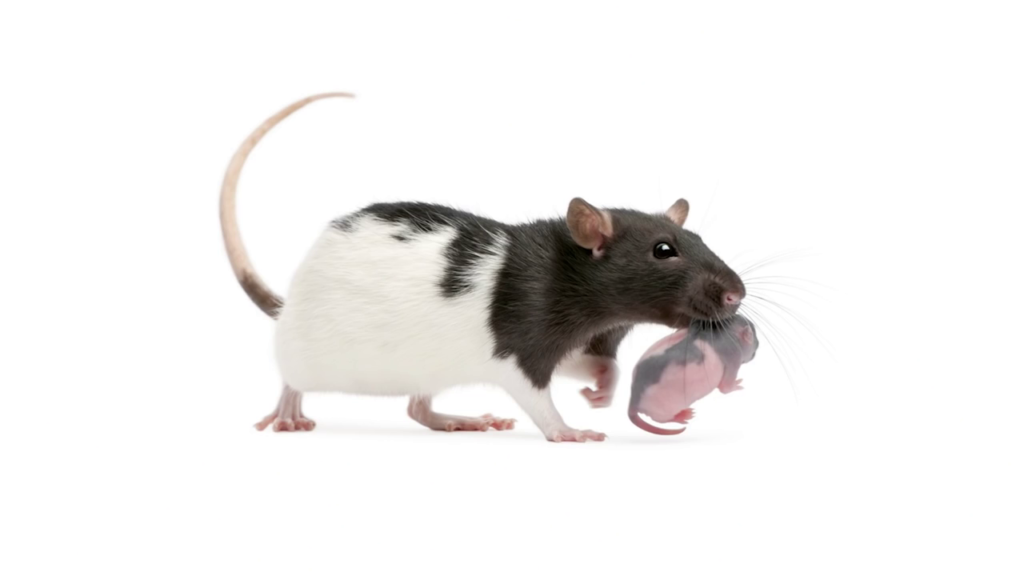
So we start not with humans, but with rats. This one’s slightly larger than life-size on this screen. The reason we’re looking at rats is we need to walk before we run. We’re not ready to do this experiment with humans yet. Finding human volunteers is also somewhat challenging because we take the brain out as part of this. So we start with a rat. In many cases, rats born in my laboratory for this purpose. And if you think that rats are dumb I just want to share with you an anecdote.
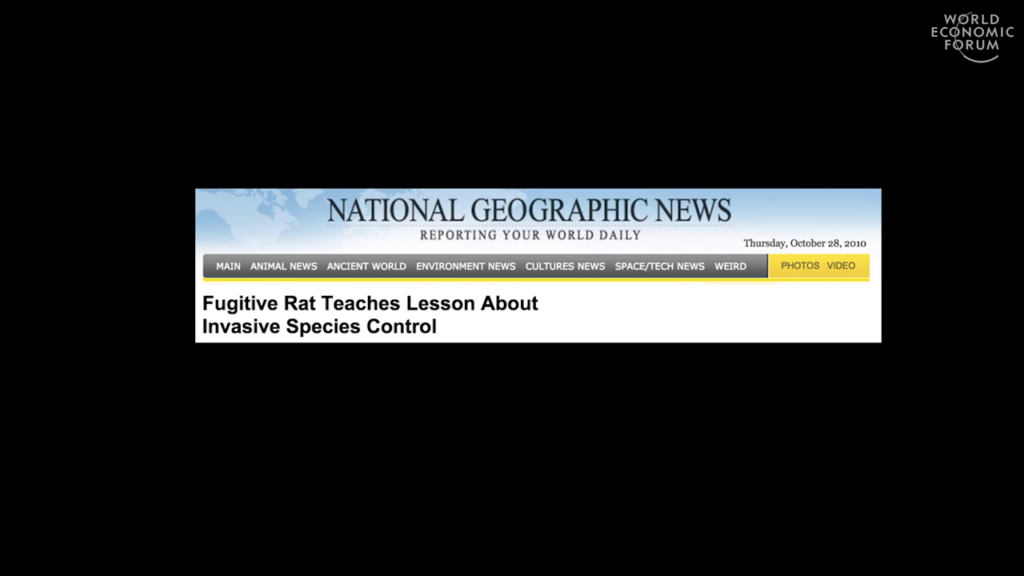
A few years ago, a group that was studying invasive species released a rat onto a deserted island with various pest control measures planted on the island, and they put a radio collar on the rat to try and test how easy it was to eradicate an invasive rat infestation. So this was an experiment, they were interested in sort of the ecology of the situation.
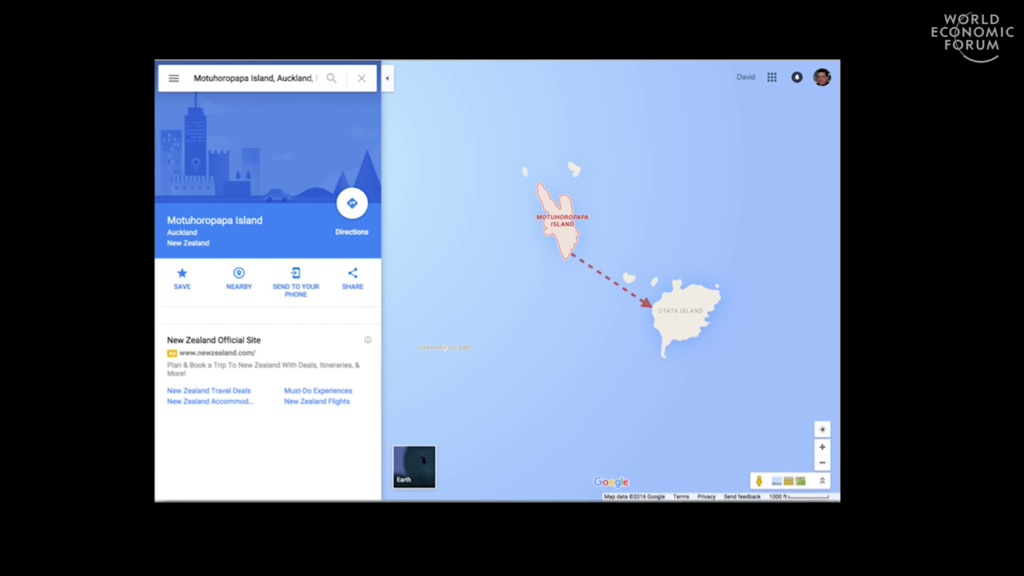
And this experiment went for a while. They tracked the rat for a while. And it was on this island here. After a week, the radio collar signal disappeared. And they went, they scoured the island, they couldn’t find the rat anywhere on the island. Even though the item was covered in traps.
And it turns out the rat had decided to swim on the open ocean to an adjacent island and was later found having swam several miles through the open ocean. So these are scrappy creatures. These are not dumb animals. And what we want to do is we want to take that scrappiness and that intelligence and understand how that learning happens and understand how that scrappy brain works.
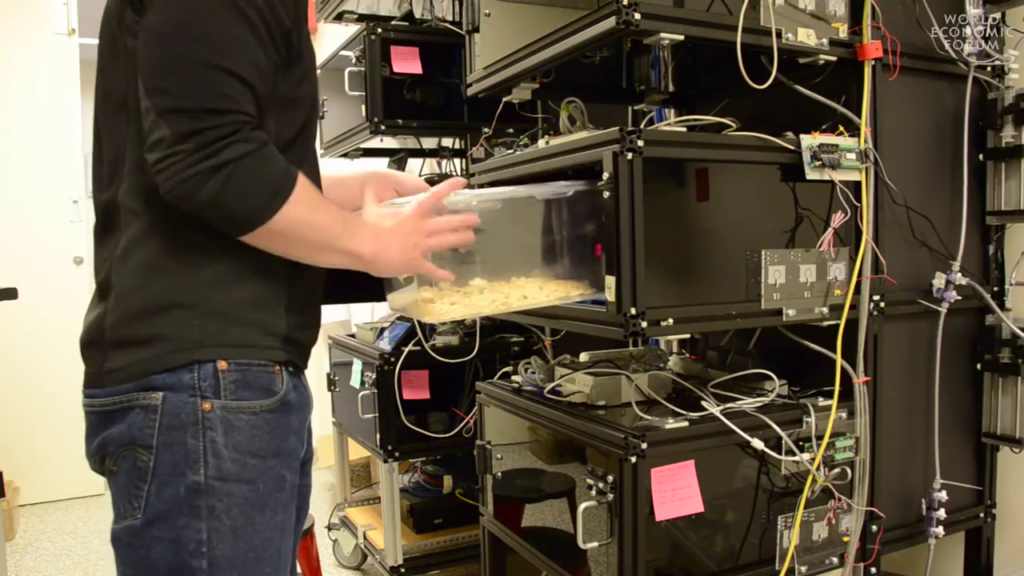
And we do that in the controlled setting in my laboratory. So these are where we train rats to do tasks. So this is basically a video arcade for rats. So each one of those boxes is a computer-controlled training rig. And we put the rat in, and then a computer takes over and trains the rat to do pretty much anything we want it to do.
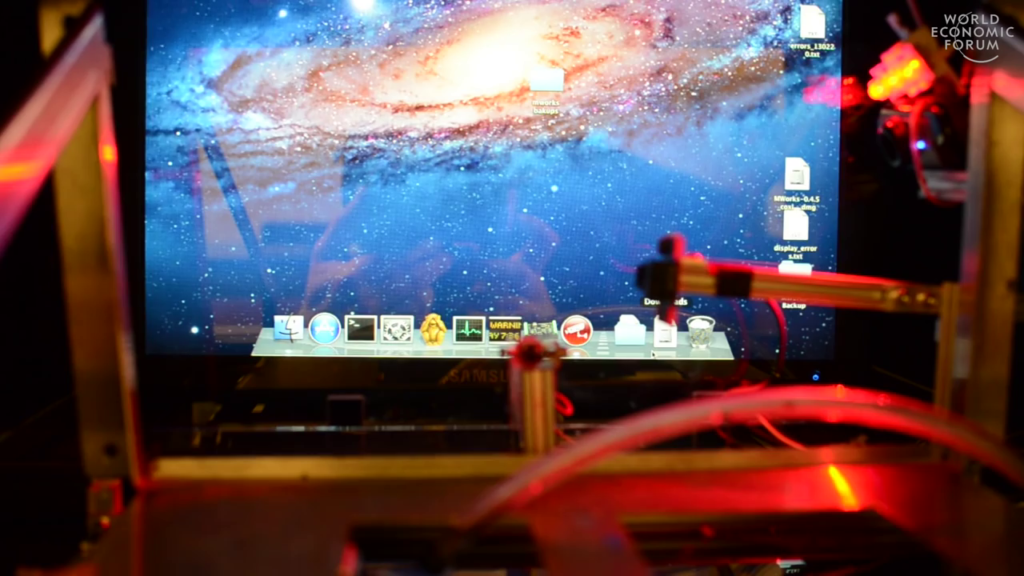
So this is what it looks like inside. And you can see the little lick tube here that the animal can lick to give us responses. We have some sensors. And then there’s a monitor, and what we can do is we can show the animal different stimuli, or different objects on a screen, and we can train them to do different things. And then we can ask, how does their brain look before they learn how to do that task versus how does it look after they learn how to do that task.
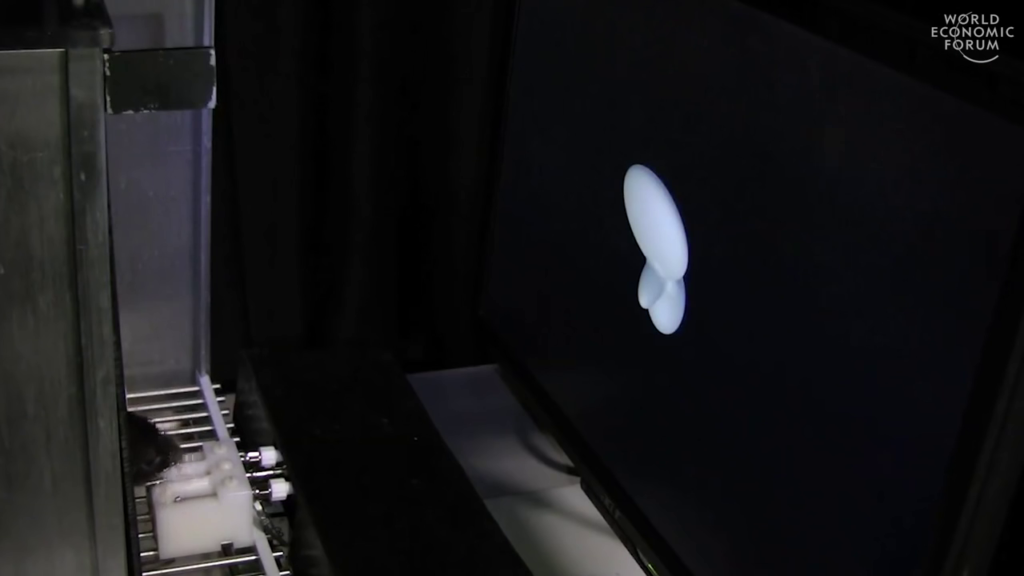
Here’s a little video of a rat doing a task. So just to orient you, here’s the animal’s nose. And you can see the rat’s happily licking here. And then objects appear on the screen. And then when he makes the right response, he gets a reward of juice that he likes. And then he gets it wrong, he gets a little short time out. So we can basically the train the animal to do these video games, and we can ask what changes in the brain when the animal knows how to do the task versus when the animal doesn’t know how to do the task.
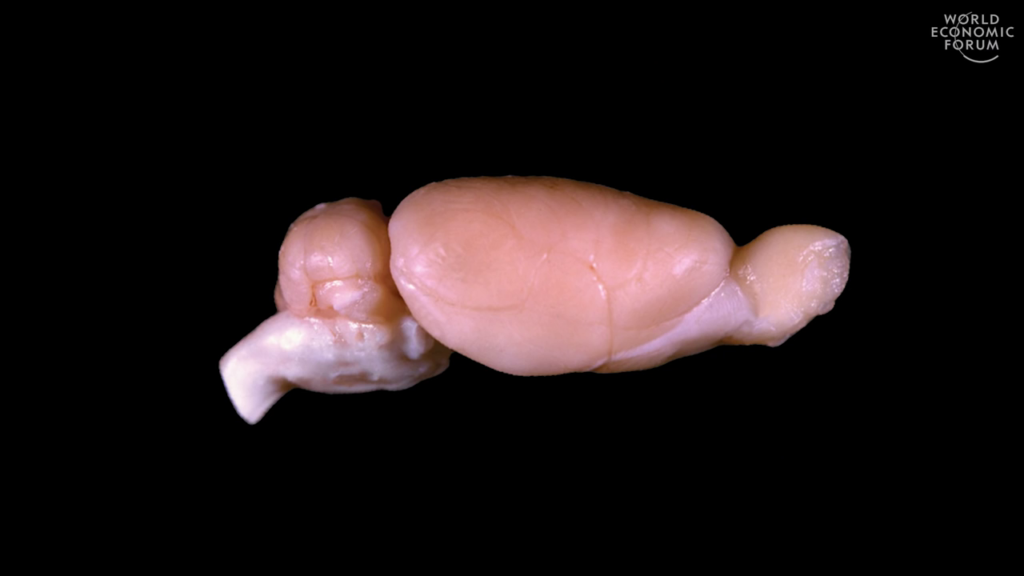
We’re not just interested in training rats, even as fun as that is. What we really want to do is we want to look at the brain and see how the brain changes. So this is a rat’s brain. And we want to look at it while it’s still in the animal’s head, while the animals actually doing something. So we need a technology to be able to sort of peer inside the brain.
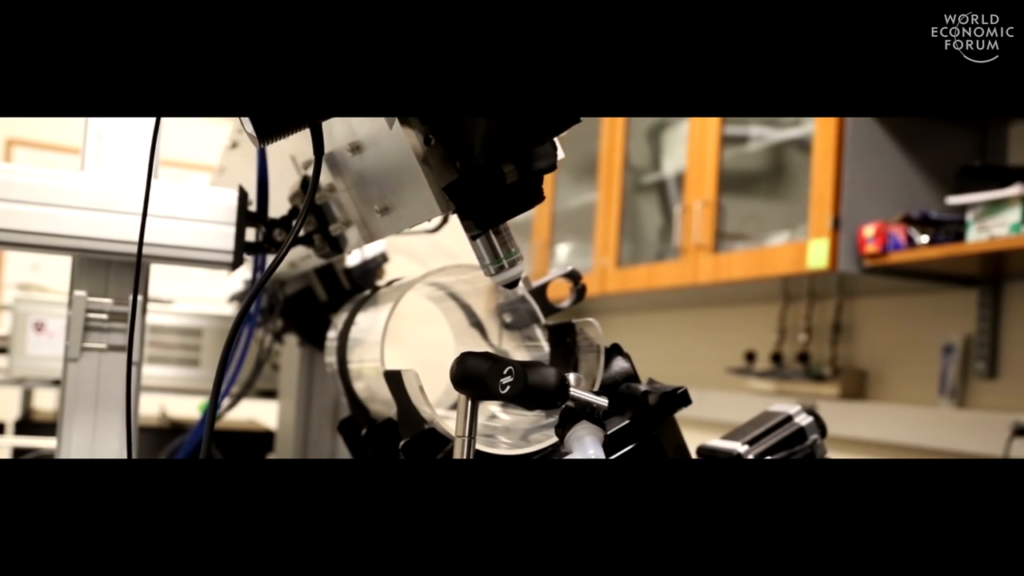
And that’s what this is. This is a two-photon excitation microscope. So this is a microscope that’s powered by a very powerful invisible laser. And we shine it into the brain. We actually have the world’s fastest two-photon microscope now, from our collaborator Alipasha Vaziri, that can basically record movies of the activity of large numbers of cells at individual cell resolution and see the patterns of activity.
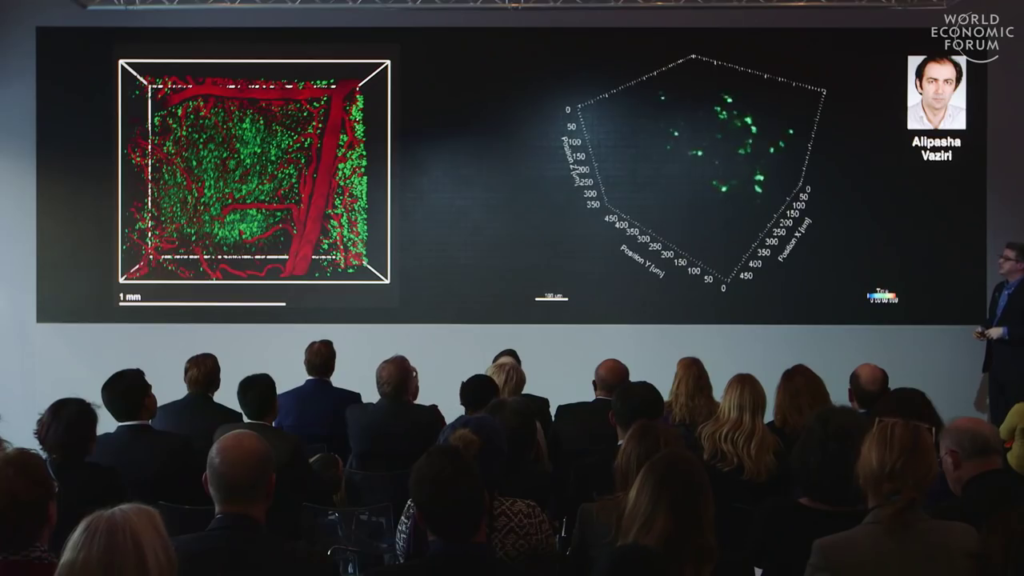
So this is what this looks like. So, you can see these flashing green dots. Every time one of those flashes happens, that’s a neuron firing in response to something in the environment. So you’re watching a rat, or in this case actually this is a mouse, having a thought. So we can actually see thought, and look at the patterns of activity, and we can see how those patterns change as we go from an animal that doesn’t know how to do something to an animal that does know how to do something.
Now we’re gonna go one step further, and we’re gonna take that brain out, and we’re gonna basically reconstruct all of the wiring between all of the neurons in the brain. So take the brain out, we soak it in heavy metals—in this case osmium, and then we put it in a FedEx box and we ship it to Argonne National Laboratory. And in particular we ship it to the Advanced Photon Source. So the Advanced Proton Source, this is an electron ring that slams an electron into a filament of metal, and then produces incredibly bright, brief pulses of x‑ray radiation. And this is basically the world’s most advanced CT machine. So if you’ve gone to a hospital and had a CT of your head, perhaps after an injury, this is basically the same thing but on an incredibly small microscopic scale.
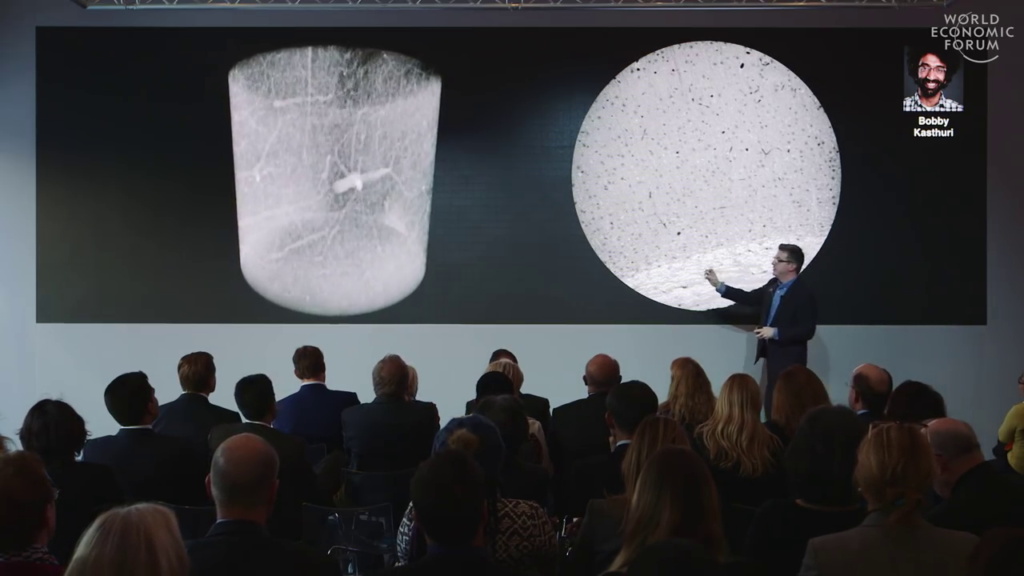
And what this lets us do is to see inside a piece of the brain. So if we have a cylindrical sort of core of the brain, we can look inside of it without cutting it, and then we can look and see every single cell in the brain, and we can see some of the vasculature, the blood vessels that serve this, and then also some of the wiring. And this give us a high-resolution picture of the brain that we can orient ourselves. But that’s not enough because IARPA asked us to actually figure out every single connection and every single wire between every neuron in the brain.
So what we need to do is we need to put it back in a FedEx envelope, send it back to Cambridge to the lab of Jeff Lichtman, who’s a close colleague of mine, to do something called serial-section electron microscopy. So, here we wanna actually see individual connections, and these are incredibly small. These are so small in fact that you literally can’t see them with light. The wavelength of light is too big to interact with how small these things are. So we need to cut them—it’s sort of like imagine a big bowl of spaghetti, but on a nano scale. And we need to slice it up into tiny slices and then image it. And in this case we use electrons to image it.
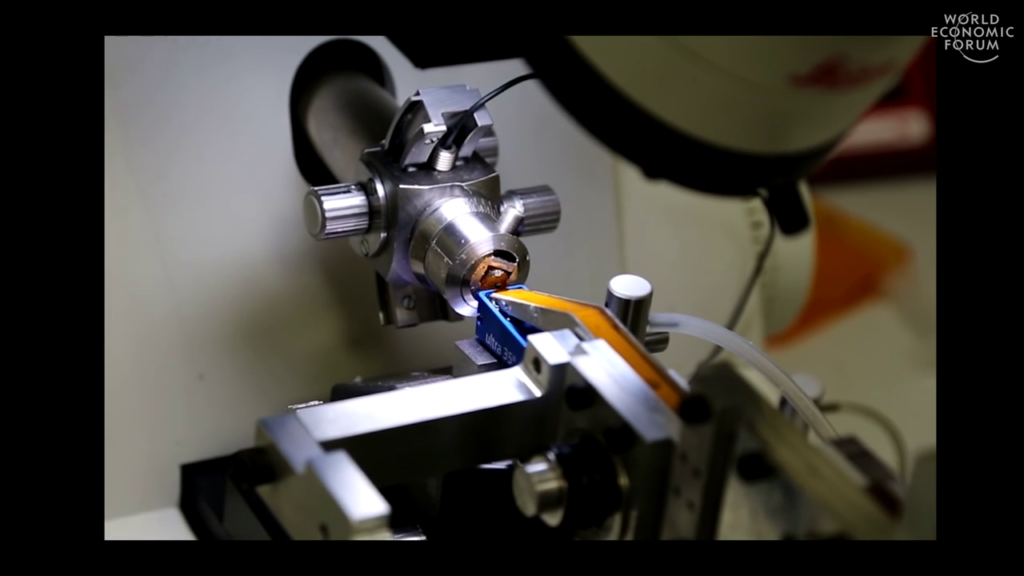
And what you’re seeing now is the world’s most sophisticated deli slicer. So, this block here is a brain, a piece of a brain that’s been embedded in plastic. And then it’s slowly carving off a slice of the brain which is then being collected onto this tape.
And to give you a sense of how thin these slices are, if we blew up a human hair, so just a hair out of our head, it’s about twenty, thirty microns wide. So this is sort of a very very very zoomed- up picture of the shaft of a hair. This is about ten microns, this white bar, so about about a hundredth of a millimeter. And then if you wanted to see how big blood cells were, that’s about how big blood cells are. And then if we zoom in even further, then this line is how thin the slices we’re cutting with that deli slicer are. So we’re. So these are thirty billionths of a meter thin.
And then we collect them on tape. So basically we have miles of tape that’re collecting these sections of this brain. And then we spool them up. Jeff’s lab cuts them up and then put them on silicon wafers, and then we just have a catalog of this animal’s brain. So every piece of their brain cut into thirty-nanometer-thin slices and then put on to these wafers.
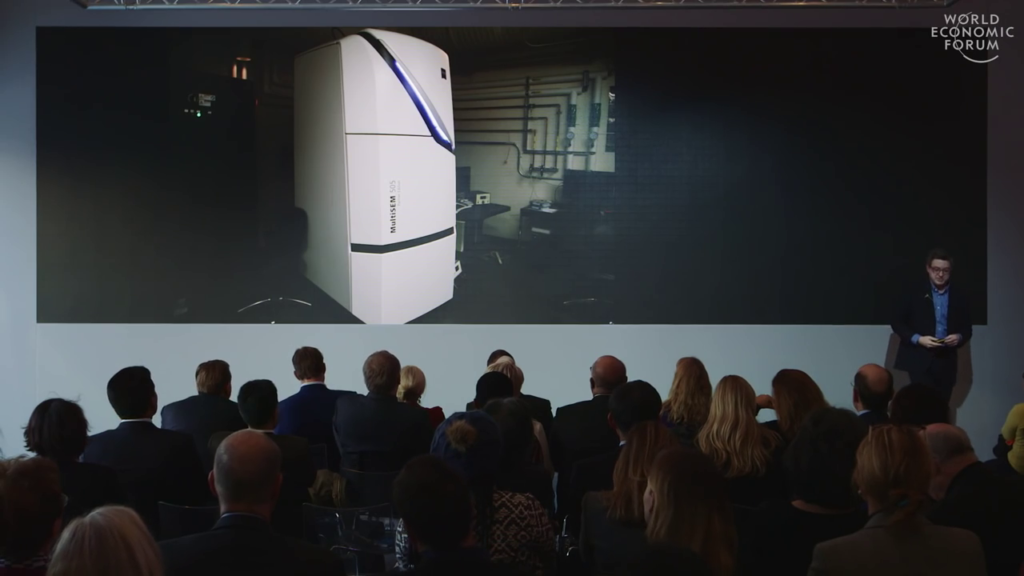
And then we image of them in this, which was at the time when it was built the world’s fastest electron microscope, which then images at four nanometer resolution and produces about two petabytes of data for a cubic millimeter of brain.
And this is what the images look like. And then they can be reconstructed. So we take all of these images and you can see we can identify each little sort of thing here. So what you’re seeing in these cross-sections are individual wires going from one nerve cell to another nerve cell in the brain. And then by using computer vision techniques, we can basically reconstruct all of these pieces.
So then we take all that data, almost two petabytes of data, and of all places it then goes to 1 Summer Street, above the Macy’s in Downtown Crossing in Boston. It turns out Harvard rents a data center space there. I actually just went to visit them recently. And the final resting place of this animal’s brain then, at Harvard, is this. This is a two-petabytes storage array. So this is a bunch of hard drives. We’re storing what remains of this animal’s brain in digital form there. And then from there IARPA wants us to deliver the brain up to the cloud. So this is a forty gigabit-per-second Brocade switch, the Internet 2 infrastructure. This is like the fast, fast Internet. And we upload that animal’s bring to the cloud.
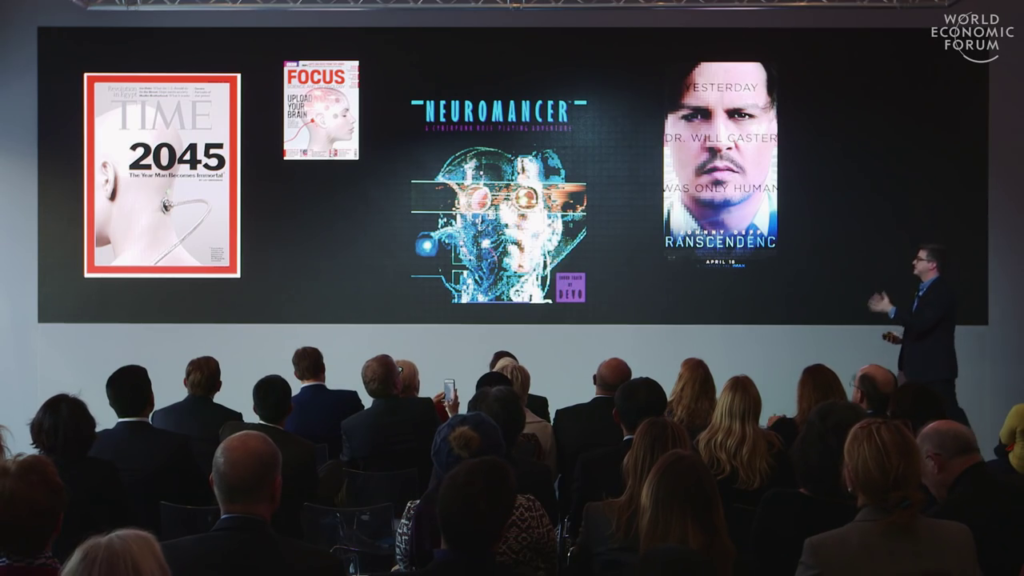
You know, this idea of bringing uploading has sort of captured a little bit of the sort of popular imagination. So you know, Time, and Focus and…magazines have started to latch onto this. What if we could upload our brain? Maybe that’s the path to immortality. Back in the 80s, William Gibson wrote a book called Neuromancer that sort of explored these themes of brain uploading. And there’ve been more recent works of art, film, that have explored this idea of like uploading the brain. And what I can tell you is that way before humans upload their brain, it’s going to be rats that get their brains up into the cloud first.
This is interesting to hear because it then drags in— Some people are taking this idea so seriously that here’s an example of a woman who recently was dying, had a terminal disease, and she decided that what she wanted to do was to preserve her brain in the hopes that people like us would figure out how to later upload her brain and reconstitute it. And using similar techniques to preserve her brains is what we used to preserve our rat’s brain.
Now, if you’re excited about the idea of uploading your brain, I have good news, I have bad news, and I have neutral news. So, the good news is there’s nothing in principle that stops us from doing this. Now, there are scientists who if you ask them they’ll say that’s crazy, we can’t possibly upload brains, never gonna happen… It could happen. I’m just gonna put that out there. There’s nothing in principle that stops us from being able to digitize a brain.
Now the bad news is we have no idea how to do that yet. And it’s going to be a long time before we figure that out. And it’s not even clear that we’re collecting all of the data we need from the brain to do that. But these are the first steps. This is what the first steps look like towards understanding enough to be able to take a brain and put it into digital form.
Now, I also promised you neutral news. And the neutral news is well before we get anywhere close to thinking about uploading a brain, many other things are going to happen first that’re going to have huge impacts on our world.
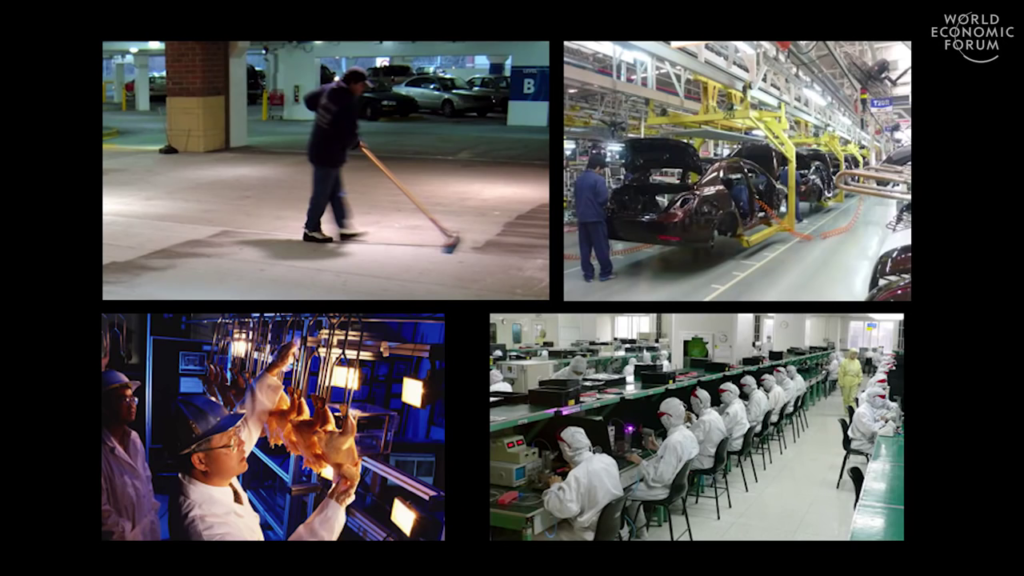
So, this notion of the Fourth Industrial Revolution that’s been very prominent at this meeting, you know, if we can capture more of what makes brains smart, and adaptable, and able to learn, there’s a huge fraction of employment that’s just not gonna stick around. So if we look at jobs like cleaning, factory inspection…you know, lots of different kinds of factory automation jobs that just basically involve the ability to see the world and interpret it correctly and the ability to use your hands to enact something in the world, those jobs are gradually gonna erode and go away as we start to build robots.
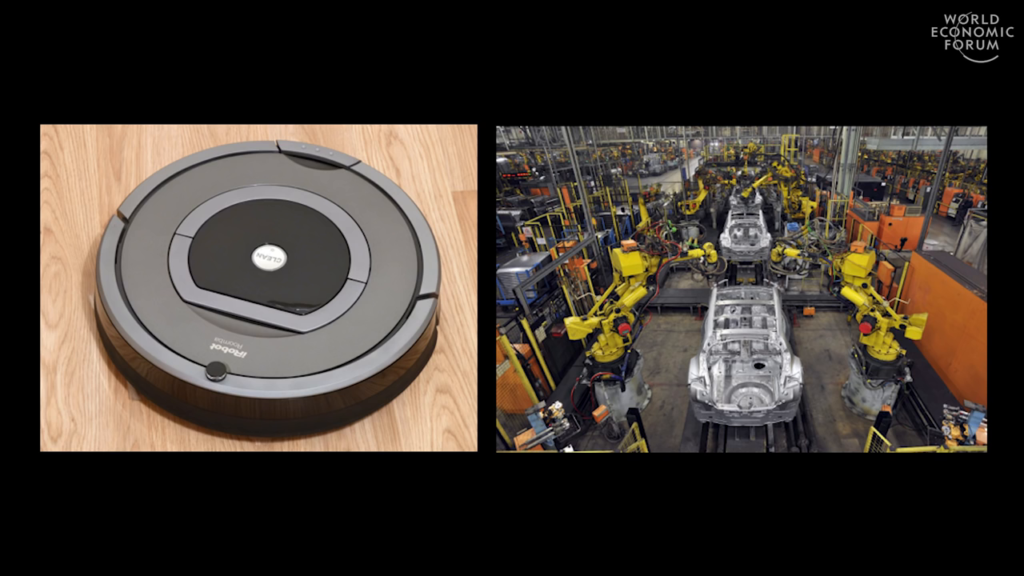
And we’re already seeing this. So you know, things like the Roomba for cleaning, and industrial robots. You might think of these as sort of the insect brains of automation. There’s not a lot of smarts here, but there doesn’t need to be a lot of smarts.
And then already we’re starting to see much more sophisticated robots, even since that 2015 video I showed you of all those robots falling over. Already the robots are getting a lot better. And we’re starting to get more flexible robots that are made to work with humans. So, as we start to learn what we’re missing in our machine learning technologies, we’re gonna see a big shift in how employment works.
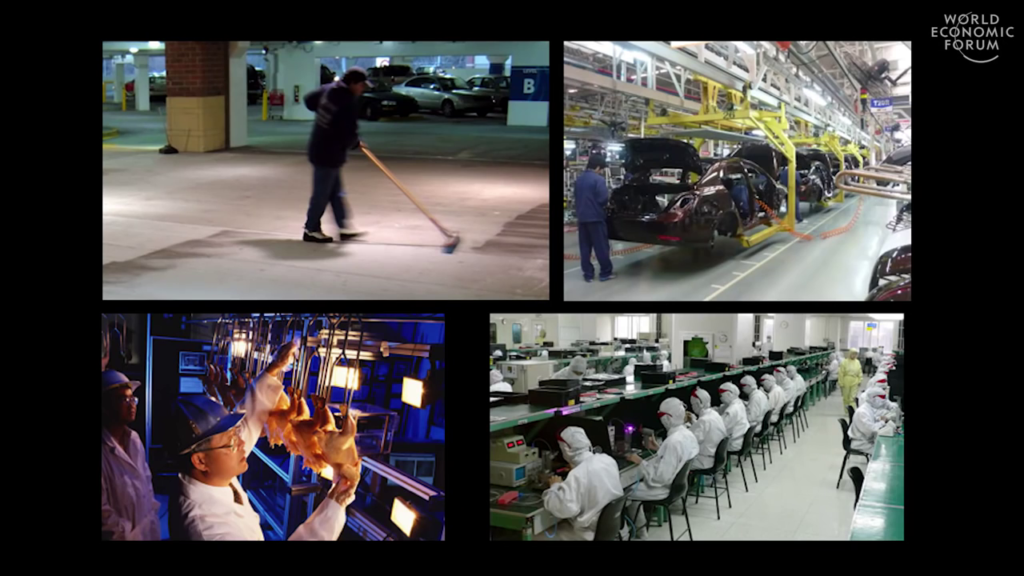
And you know, one of the areas that’s super hot right now is self-driving cars. I would submit that the brain power of a rat, properly applied, is sufficient to drive a car. I’m not saying that people who drive cars are rats…please… But you know, a rat has a lot going on in it’s brain. There’s no reason the car needs to chase cheese. Like, if we understand how this works we can start to tackle these problems.
The urban driving problem is quite difficult and I think it’s gonna be a long time before we solve it. But highway driving is perhaps closer and within reach. And people are already starting to look at trucks, having self-driving trucks deliver goods in a more efficient way.
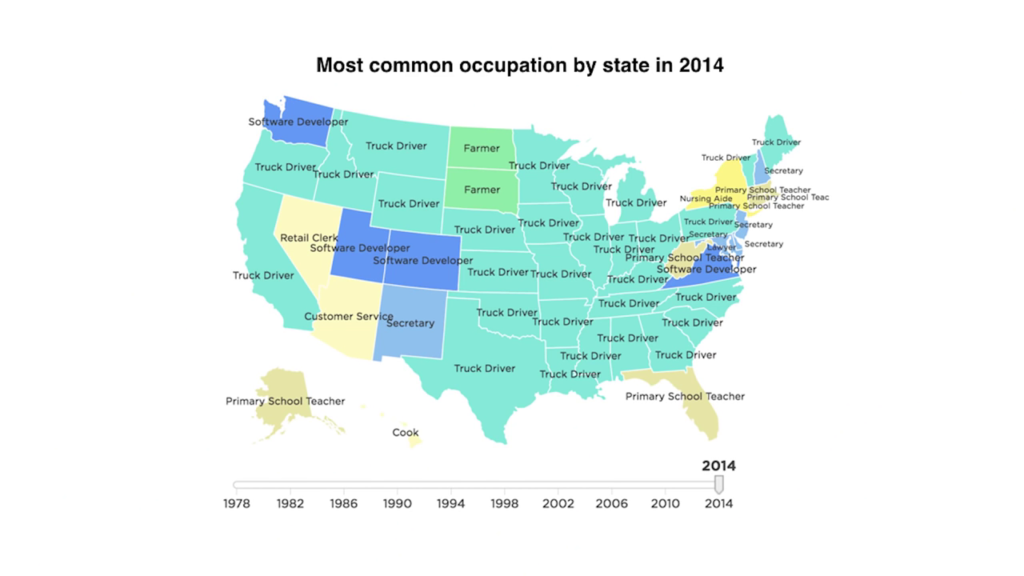
Unfortunately if you look at a map— And sorry this is a very US-centric view, because I’m from the US. But if you look at the most common occupation by state in the United States, quite a few states have “truck driver” as the most common occupation. So as we start building systems that can replicate what our brains can do, we’re gonna have to find something for those brains to do.
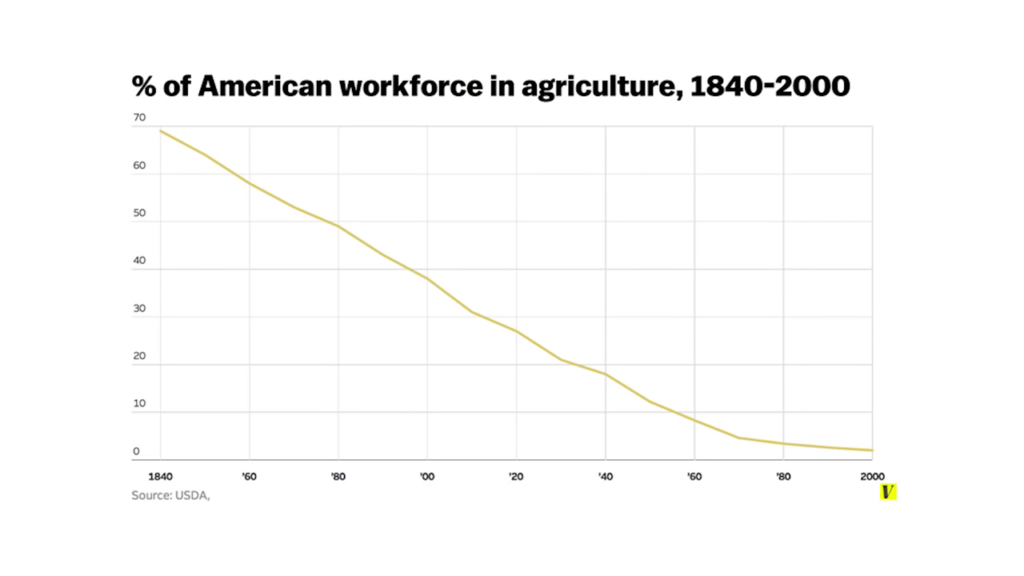
The good news is we’ve done this before. So if you look at a plot of the percentage of the American workforce that’s engaged in agriculture, back in the 1840s was about 70%, and we’ve basically taken that down to almost zero. This time maybe will be different but you know, we have to think about how these technologies as they increase are going to affect things.
And I think one of the reasons why I’m excited to be here at the World Economic Forum is because I think we need to start dialogues with many different kinds of stakeholders, people with many different kinds of expertise. And already in this project, we’re engaging neuroscience, we’re engaging physics, we’re engaging computer science, high-performance computing. But we also need to start engaging law. We need to start engaging business leaders. We need to start engaging policy, and ethics. And I think that the challenges that lie ahead—the opportunities are enormous that this technology enables. But we also have to think very seriously about those consequences. So, thank you for your time.