David Cox: So my name is David Cox, and I’m a professor of biology and computer science at Harvard. And I’m gonna tell you today about an exciting new frontier at the intersection of neuroscience and computer science. And part of what’s exciting about this is that neuroscience and computer science are to the fastest-moving fields that we have today. And for many of you when you think about neuroscience, the first thing that comes to mind might be medicine, and health. But I’m gonna argue, I’m gonna try and convince you that actually neuroscience is much bigger than that, and that the stakes are much larger.
So, science is about understanding the world around us. But it’s also about understanding where we fit into that world. And it’s human nature to look at ourselves and try and understand how we fit in. And more than just how we fit in, how we’re special. And there are many things that we could think about being special that are different about humans from the rest of the world. And we might even be tempted to think that we’re some sort of pinnacle of evolution. But it turns out that biology teaches us otherwise. We’re just one out of millions of species on this planet, each of which is exquisitely adapted to its niche.
We’re not the most numerous species. We’re not the largest. We’re not the fastest, or the strongest. We’re not the longest-lived. We’re not the most resilient. So what, if anything, makes us special?
Arguably, the thing that makes us unique is our complexity. But not complexity in some generic sense. Nature is rife with complexity. What makes us special is the complexity of our brains. We more than any other species can learn, and adapt, and shape our environments. Pass on culture. And we’ve spread to every corner of the planet, and even beyond it. Every work of art, every edifice of our civilization, is born of activity in our brains and born the complexity of our minds.
And meanwhile, we’re slaves to that complexity. If that complexity strays even just a little bit we can collapse underneath it and have mental disorders, and disease, and at the same time all of the great things that our complexity is able to produce also produces all the bad things that are facing us today. So, I would argue that understanding the brain is tantamount to understanding who we are. And I think we should all be interested in neuroscience. I may be biased.
And we’ve been thinking about it for a long time. So what is it about the brain? What is it about this complexity? How does it work? And interestingly, when we look out into the world, oftentimes we look at it through the lens of our own technology of our day. So, in the 17th century Descartes, a great philosopher and mathematician, thought about the brain in terms of the technology of the day, which was hydraulic technology. So he believed that the seat of the soul was the pituitary gland, and that fluids animated our body much like a hydraulic system would.
Fast-forward to the 19th century, Sigmund Freud used the analogy of the technology of his day, the steam engine. Talked about pressure being released and built up. Talking about the mental states of our minds being driven by the engines of our conscious and subconscious.
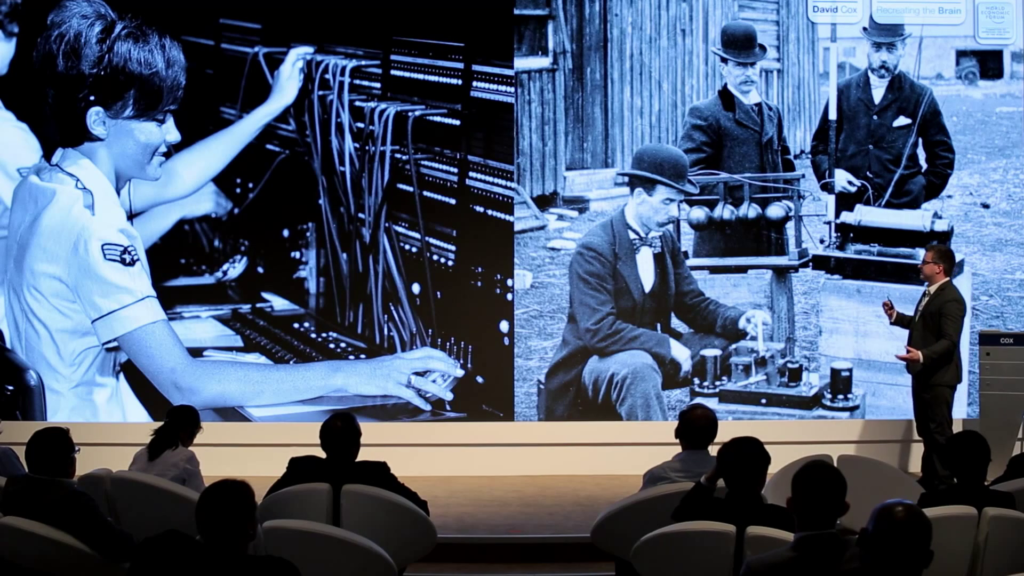
And we fast-forward to the 20th century, the era of radio and electronics. And all of a sudden we start talking about our mental processes these ways. We talk about wavelengths, and crossed wires, and channels.
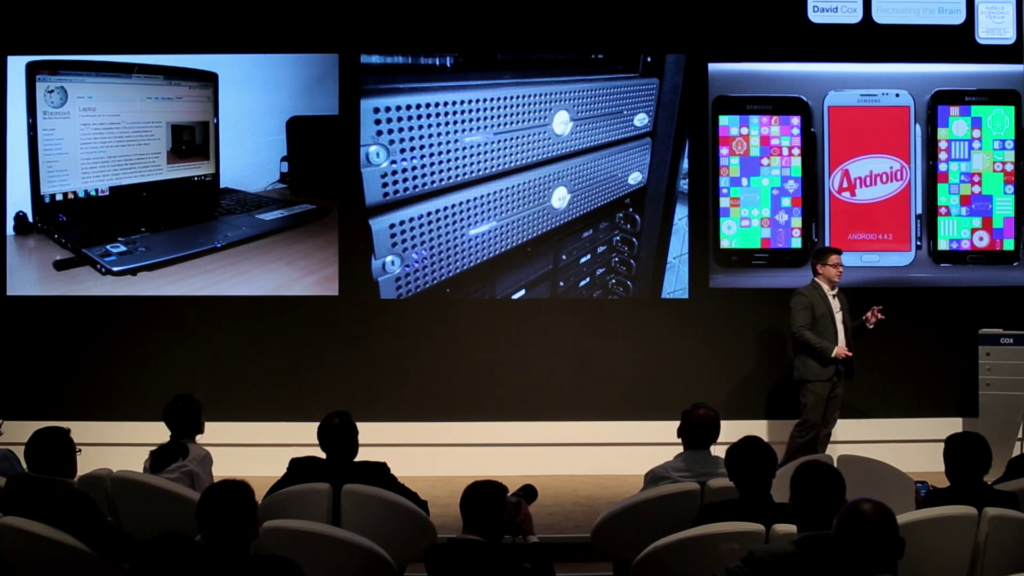
And now today, we have computers. So, increasingly neuroscientists talk about circuits. And we talk about brains passing information, processing information. We talk about networks. As our technology advances, so too do our metaphors. And it’s very easy to be led astray by our metaphors. There are many ways in which our brains are not like the computers we have on our desks or in our pockets. But what’s different about computers is that this metaphor is actually more than just a metaphor. Computer science gives us the formal tools to evaluate a computational system, a system that processes information. So, even when we’re faced with something that has a different implementation, we can separate out what’s computed—an algorithm, from how we compute it—an implementation. And this gives us tremendous power to reason about computational systems, including ourselves.
So why is this important? Well, first of all health today, mental health, is in many ways the last frontier of neuroscience. Increasingly, we’re able to treat many of the diseases and disorders that afflict humanity, but mental disorders, diseases, are in many ways the sort of last frontier. And part of the reason for that is that our tools that we use to treat them are relatively crude. So most of these pills—this is Prozac—are small molecules that target molecular systems, receptors, that act throughout the brain and in fact throughout the body. Prozac actually also works on the heart. Because they have such diffuse effects, it’s very hard to target their action, and it’s very hard to prevent off-target effects. In many ways it would be like going to your IT department with your computer to have them fix it and all they could do is to change the silicon properties of the computer chips inside. They might be able to fix the problem some of the time, but that’s not really the right level of analysis, the right approach to fixing that problem. Instead, you really need to understand the software of the system. And if we could understand the software of the brain, then complex disorders like schizophrenia, and obsessive compulsive disorder, and depression, which aren’t caused by any sort of overt obvious damage to the brain but are probably more like miswirings and problems in the software. And increasingly, we’re starting to get to the point where we do understand at a computational level some of the codes that the brain uses that we can then interface with.
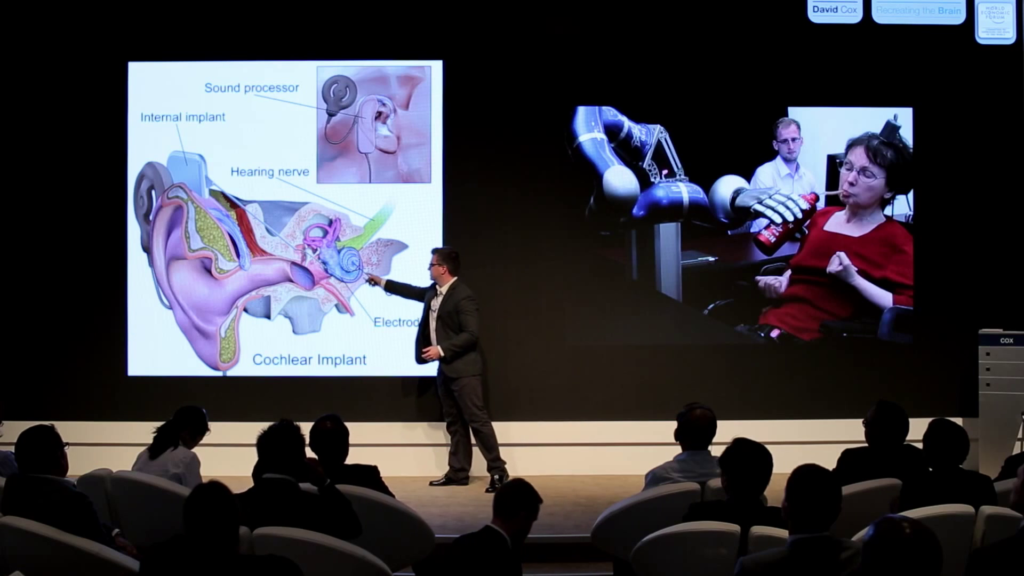
So on the left here we have a cochlear implant, which is one of the earliest sort of bionic implants. It’s a series of electrodes that are inserted into the cochlea of the ear, and you can restore hearing in some cases. So this is a direct interface to our nervous system.
On the right, we have BrainGate. So this is an exciting new technology but also at a very crude sort of infancy stage. Here this woman is quadriplegic, and that thing on her head is actually an electrode array that’s inserting electrodes into her brain, and then those electrodes are reading activity from her motor cortex and then using it to move an arm. So increasingly, we can interface with the brain, if we understand how it works.
Now, there’s an even bolder and broader set of things we can do if we can understand at a computational level how the brain works. So, if we could take those codes, if we really understood how the brain works, we should be able to build it. And the famous physicist Richard Feynman once said, “That which I cannot build, I do not understand.” So, that’s really the mantra of what my lab does. And through this lens we’re basically looking and asking can we reverse engineer the brain? Can we study the brain’s wiring and circuitry so that we can build computer systems that work the same way.
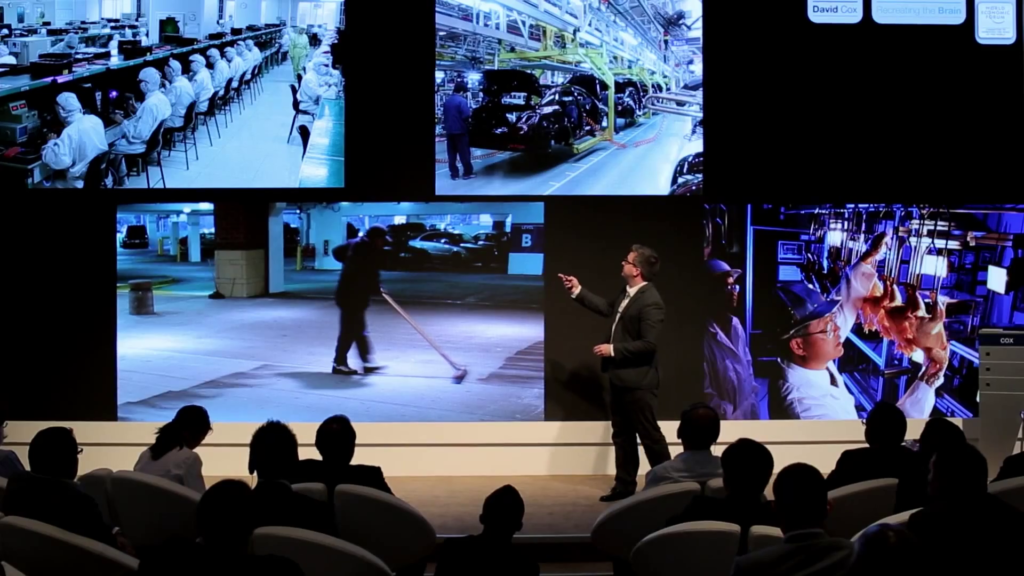
So, the consequences this might not be immediately obvious. So let’s just take a moment to think about all the different jobs in the world. So, here we have some factories making cars, making iPhones. We have people sweeping the street. We have people looking at poultry. A surprising fraction of the world’s jobs mostly require a working visual system, so we can see and understand what we’re seeing, and a working motor system, so we can take hands and we can move them and we can manipulate our environment.
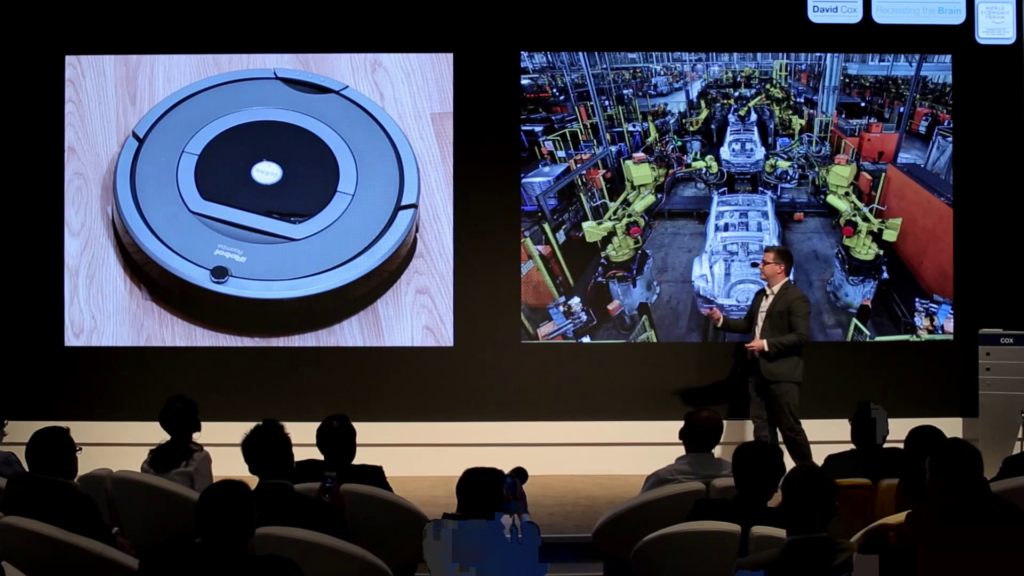
But at the point at which we can recreate these abilities in computers and in robots, a lot’s going to change. So, here are some very crude robots that are sort of the advance guard in this new revolution. We have the iRobot Roomba, which is basically replacing somebody sweeping the floor. It has a very simple brain in it, perhaps more like an insect than like a person. We also have these industrial robots which have been around for quite a while. But what these require is a highly-controlled environment where the robot needs to move—it needs to have the thing be where it needs to be at the moment it needs it to be there. And it’s all a very highly-choreographed, very highly-controlled system.
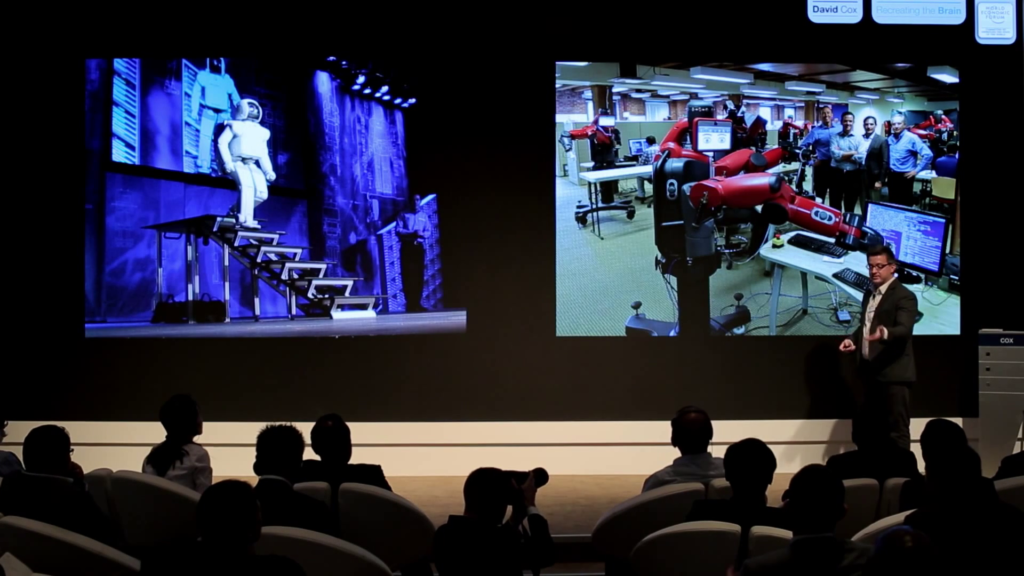
But increasingly we’re finding robots now that are gonna break that mold. So already we have this advance guard of Asimo, which is a bipedal walking robot made by Honda. It doesn’t have a purpose per se other than to be a showcase for robotics. But people are already starting to think about using robots like this for domestic servant kind of roles. We also have this robot Baxter which is from Boston, a startup coming out of MIT. And what Baxter is is it’s robot with two hands that can be trained alongside a human to perform tasks. And increasingly beyond the industrial robots that I showed you in the car factory, this system can adapt to different conditions, it has some rudimentary vision. So, as we imagine to have an understanding of the brain to be able to build more and more complex abilities into our computers, then we’re gonna see a renaissance in robotics, and that’s really going to change just about everything about our economy.
There are also jobs that aren’t jobs currently. So there a lot of things we’d like to do, that only humans can do, but that we can’t scale up to a scale that we need. So this is an example that’s literally close to home for me. The Boston Marathon bombers planted a bomb, they were caught by many many cameras. So it turns out now nearly every storefront, every shop has a camera in it, people were taking pictures of the event. They were documented multiple times moving around, dropping the bomb… But interestingly, even after the fact when the authorities collected together all of the images, it wasn’t possible to find out who they were. They had pictures of them, they had pretty much— Right in this spot you can see one picture there. Here’s another picture. And this turns out that this is one of the bombers right here. And then lots and lots of these photos.
But it turns out that face recognition software was not useful, at all, in discovering these bombers, even though we had many pictures of them and we had pictures to match against the technology that we have currently for doing machine vision, for having computers look at images and understand them, wasn’t up to the task. Now, we know that humans can do this task, because the friends of these brothers saw these pictures online and then went and destroyed some evidence. So they were clearly able to do it. But what we weren’t able to do is to deploy at scale the kind of human resources that we’d need. This is just something that computers can do well and humans can’t. So if we can build human abilities into machines, then scalability becomes not an issue anymore.
So, we want to study the brain, we want to reverse engineer it. That’s an awfully big piece to bite off all at once. So, it turns out the human brain has 100 billion neurons in it. And it has 100 trillion connections. So we can’t just understand it all at once. So what we do and what many other labs do is to focus on one subsystem in particular. And for a variety of reasons, I study vision.
Now, obviously vision from the examples I gave you has sort of industrial relevance that’s hard to argue with. But in addition it’s one of our most natural senses. We as primates use our vision all the time. We’re very good at vision. And we frankly take it for granted.
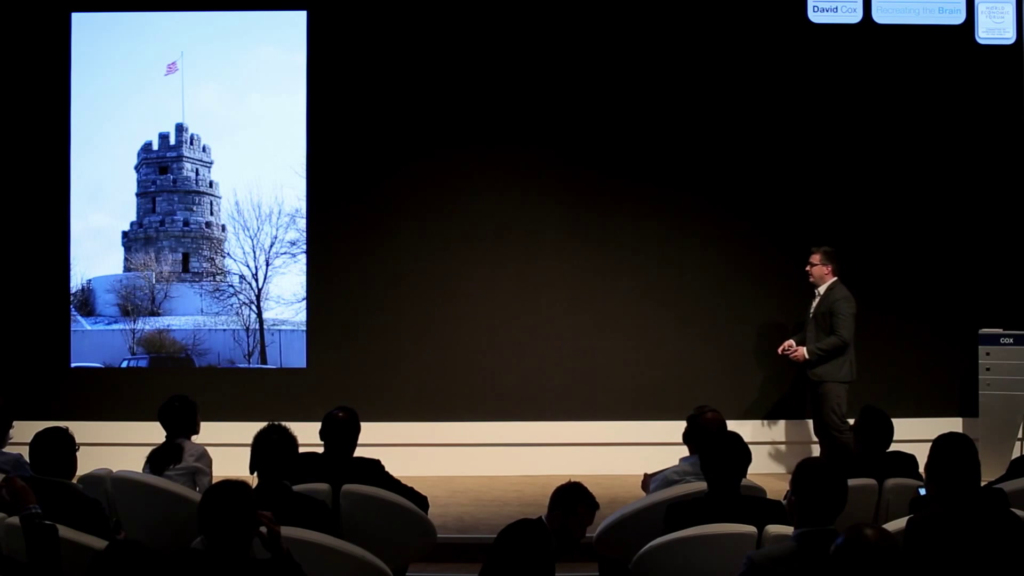
So if we look at an image like this one, even if you haven’t seen this structure—this is close to where I live—instantly without any effort you’re able to read out all kinds of information about the scene. So you can tell that this is a castle, you could tell me which way the wind is blowing, you could probably tell me how cold it was that day.
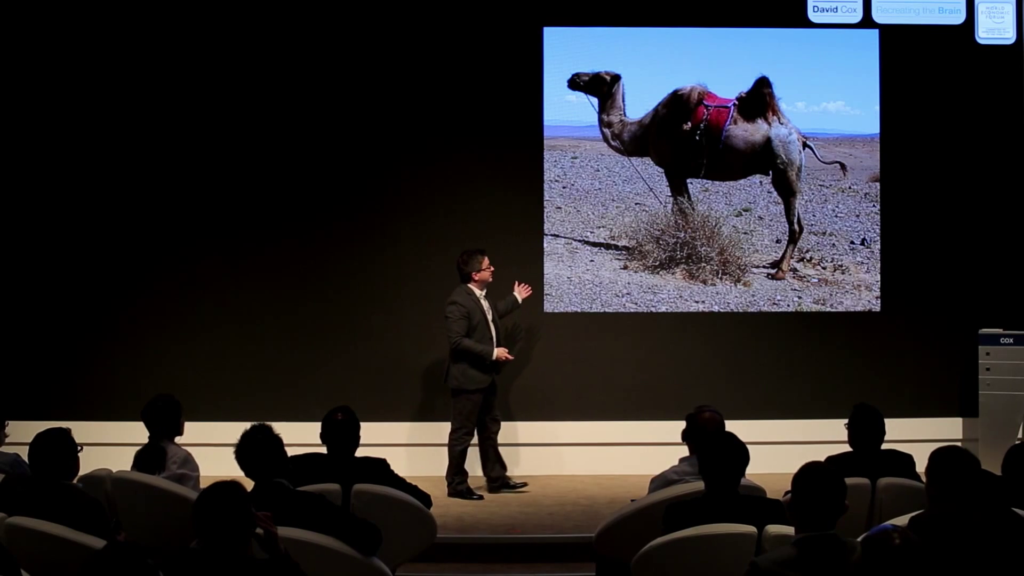
If I take another picture like this camel, even if you haven’t been to the Gobi Desert and you’ve never seen a camel like this before you instantly recognize that this is a camel. You could probably tell me what it would sound like to walk on the ground in the scene.
So, all of that you got instantly from the image and you don’t have to exert any effort. And one of the things that’s frustrating frankly about studying vision is that everyone thinks it’s easy. Because you just look at things and you see them. But the reason it’s easy is because you have the solution to the problem in your head and it evolved over hundreds of millions of years.
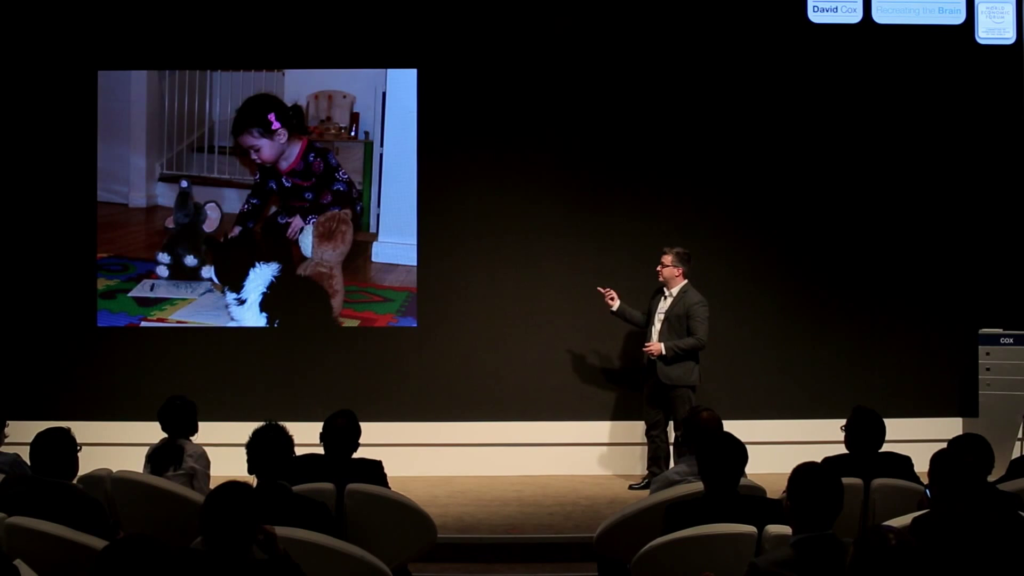
So let me give you some insight on why this is actually so hard for computers to do even if it’s easy for humans to do. So, for one thing here’s an object in the world. That’s one I care about quite a bit; this my daughter. This is presumably the first picture you’ve ever seen of my daughter.
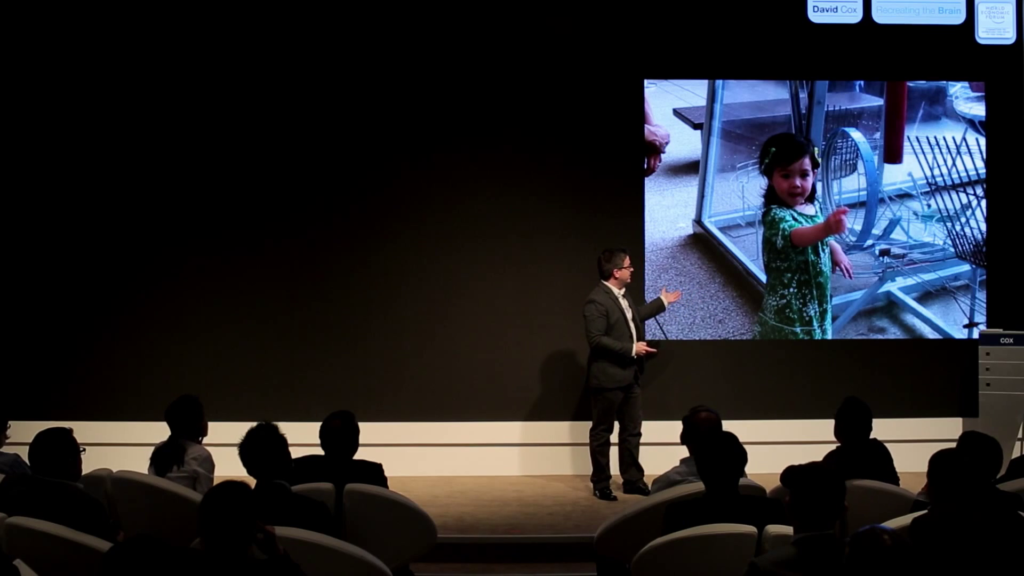
But if I see another picture in a slightly different pose, different lighting, everyone can instantly recognize that this is the same person, this is the same thing in the world. But at the pixel level these images have almost nothing to do with each other. The colors are different. The arrangement of pixels is different. Computers have a very hard time telling those two things are the same thing. And we can also deal with incredibly rich and complicated occlusions, and different views and lighting, so we can instantly recognize that. And it’s frustrating at some level to try and build computer vision systems, systems that can do what we can do, because we take it so much for granted and it’s actually such a hard problem.
So, any given object in the world can cast infinitely different images on your retina. And actually it turns out the converse is true as well. So, any given image in the world can correspond to infinitely many different objects in the world. So has anyone figured out what’s going on in this image? Who says magnets? No, no magnets today.
So this is actually an illusion. And actually many of these illusions are playing on this tricky piece about vision. So, any given object can cast infinitely many different images, because we can change your view and lighting. But any given image could actually correspond to infinitely many different objects. And this particular illusion was constructed to take advantage of that. I mean one interpretation of me looking out of at this audience is that I’m standing inside a sphere and you’re all just painted on that sphere in this particular arrangement. It’s not a good interpretation of the world, but it’s a valid one. There’s actually no proof that that’s not the answer. And this is what we call in science an ill-posed problem. We have a three-dimensional world outside, and we’re measuring it with a two-dimensional structure. Our retinas are a two-dimensional structure, so we have to make inferences. We have to be guessing about what’s in the world, and our visual system is very good at guessing the right thing. It gets it right more often than wrong, and that’s why visual illusions are so compelling, is because they violate those usually very good assumptions.
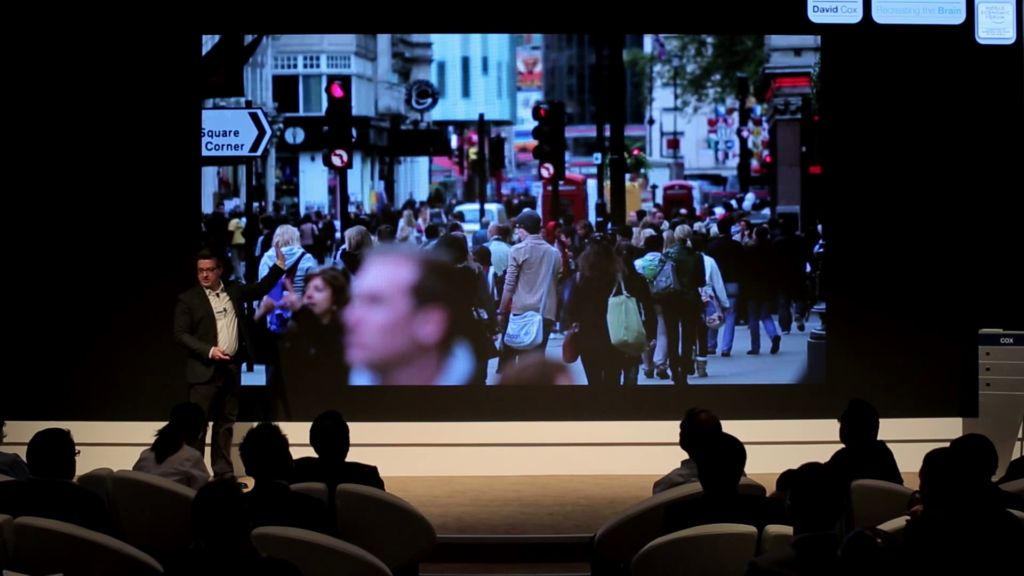
The other thing about vision is we’re constantly dealing with incredibly complex and ambiguous information. So here we have a street scene. And I think all of you could probably make an estimate of how many people roughly are in this image. And I think we’d all agree that there are people on the other side of the street as well, right. So there’s people in the foreground, we can see them somewhat clearly. There’s also people in the background.
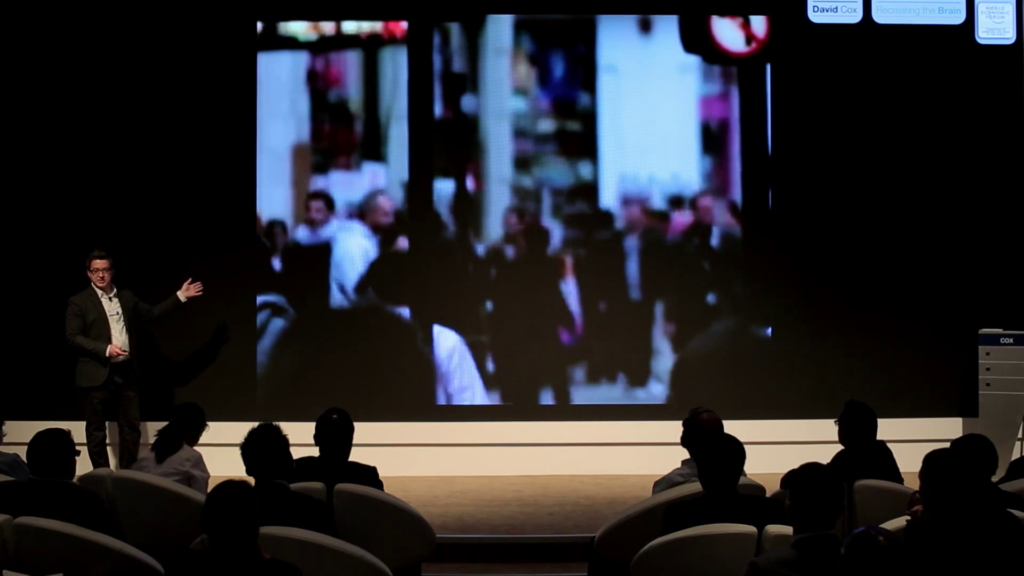
If we zoom in on part of that background, this is what you were actually looking at. This is exactly the same information just blown up a little bit.
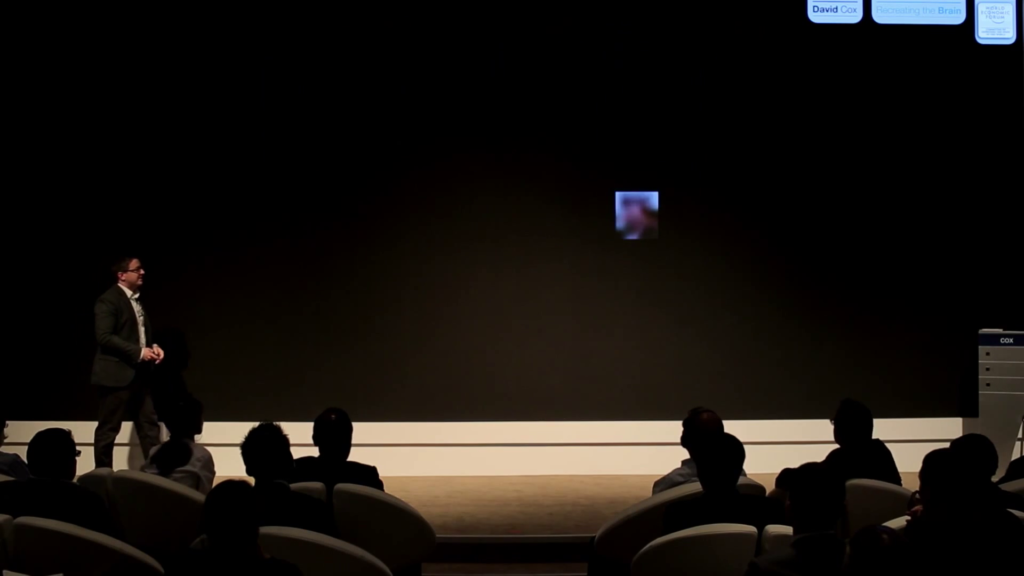
And if cover we this up, you were certainly able to recognize that there were people on the other side street, but you didn’t actually have any information to prove that, or to give you that impression. The information you used to know that there were people on the other side of the street was the context. You were able to integrate a model of knowing about how street scenes work, knowing how people—where they should be, where the heads would be, and you were able to infer a lot of things and perhaps even to the level of almost hallucinating the impression that there were these faces even though you couldn’t see them. There wasn’t actually any real information. So, these are amazing abilities that we don’t yet know how to build into computers.
So what do we know, though, about biology? So, if we take an image in the world, the photons are projected onto the retina, which is a two-dimensional layer of tissue on the back of the eye that transducers the photons into an electrical signal that goes across the optic nerve to the brain. Now the brain is a massively-parallel computer made up of 100 billion elements in humans. And each neuron, so each computational element, is actually a computer unto itself. So it takes inputs in, and it puts outputs out, over some hundred trillion connections between these neurons.
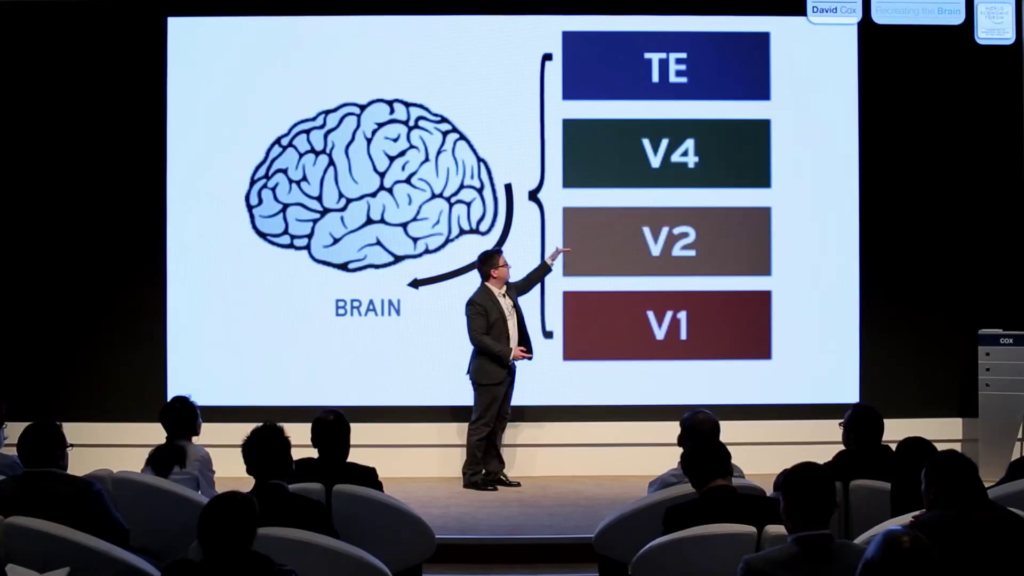
Now, it’s not just diffusely organized, there’s actually a very interesting structure to the visual system. It’s arranged hierarchically. So, information comes in at the back of the brain into an area called V1, and then successively information is sent to waystations where it’s processed and transformed. And these areas are called V1 for Visual Area 1, V2, V4 (don’t worry about where V3 went), and then there’s an area called TE, which is the temporal cortex. And there are actually quite a few more visual areas. It turns out that in vision there’s a segregation between our processing of what something is and our processing of where it is and how fast it’s moving and things like that. So some of the other “V” numbers correspond to that other stream of processing.
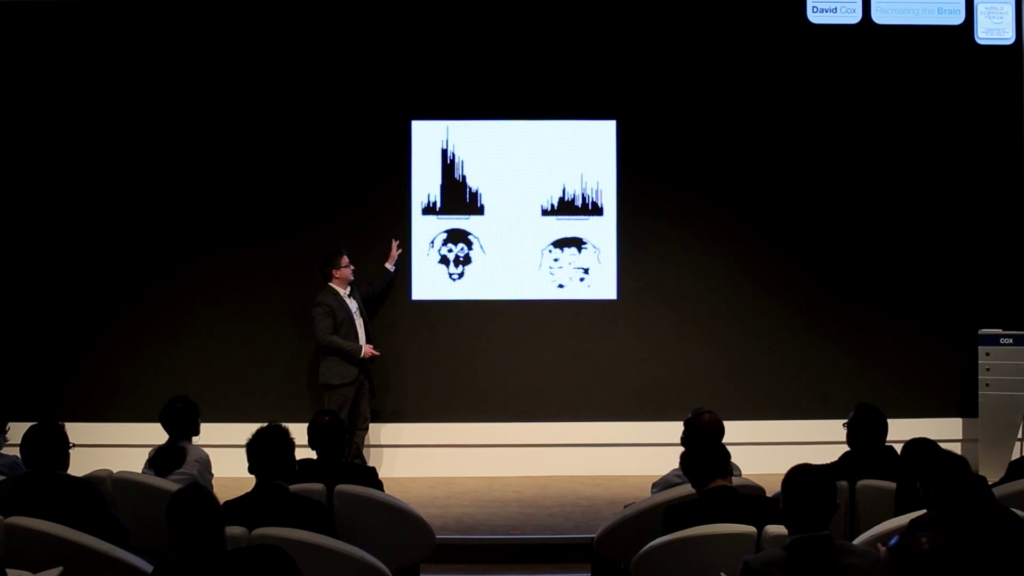
So, it’s interesting what happens. If you record from the neurons in these areas and measure their activity you find that neurons in area V1 are primarily concerned with small, simple structures like little edges. And then if we go up to the highest levels in area TE, also called Area IT, we find really interesting neurons. So here’s a figure from the 1980s from Robert Desimone. And what he did was he showed a monkey with an electrode in its brain, in this area, images of a face and an images of scrambled faces. So they have comparable complexity, visually, roughly speaking, but this one forms together to form a face and that one does not.
And what you see above is the firing of the neuron in the brain. So, you don’t need to worry too much about what this means other than up means more firing, and that way [indicates to the right] means forward in time. And this little bracket shows you when the stimulus was up. So without thinking too hard about it, you can clearly see that this neuron seems to like faces. It fires when you see faces. And this is actually quite magical when you have an electrode recording from a neuron and you’re showing stimuli and figuring out what the cell fires in response to. It’s probably a little bit more complex than just saying that this is a face neuron? But at the same time it’s reasonable to say that this neuron represents the face.
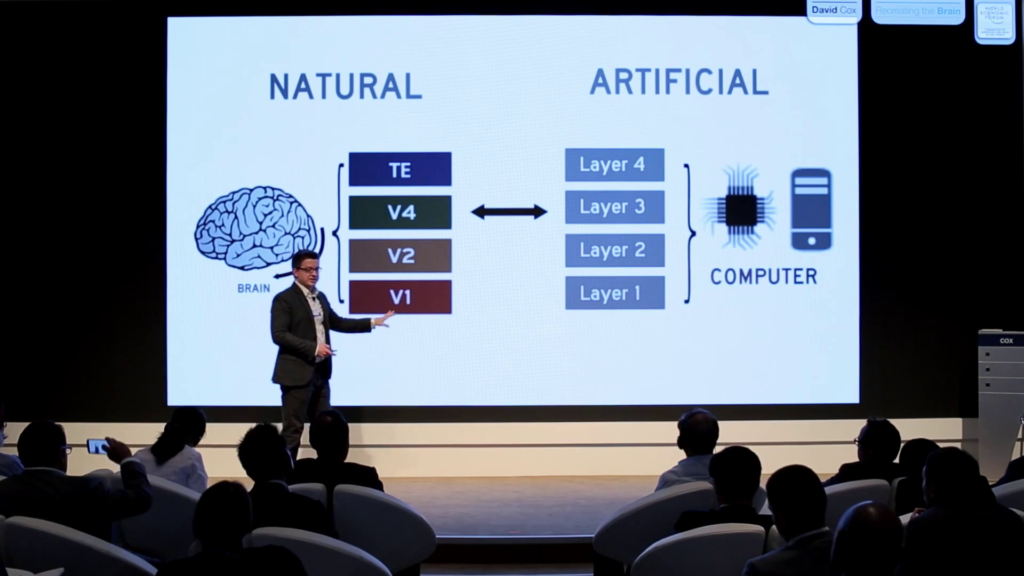
So then, we take all this information and then what my lab and other labs do is try to take inspiration from the natural system and what we can glean what we know about the natural system, and then build an artificial system that shares the same structure and shares aspects of the same processing. And where the natural brain is made up of billions of neurons, the artificial system is built up of artificial neurons that are basically functions. So what we need to do is study the system, figure out how to build versions like that. And then we can deploy these in a variety of contexts. My lab uses these for face recognition, face detection. We all use them for robot navigation and a variety of other different tasks.
So the problem is that the processing power of the brain is actually quite a bit more than the processing power of a computer. So it’s at least petaflops of computational power in the brain, which is remarkable also considering that it only dissipates somewhere between fifteen and twenty watts. So, it’s using about as much power as your laptop and yet it’s as powerful computationally as some of the most powerful supercomputers in the world. So that’s an interesting fact in and of itself.
Eventually we’ll figure out how to get that power efficiency, but in the meantime what we do is we build up large clusters. We use lots of computers to try and mimic the power or the abilities of a brain, and we’ll worry about the power later. We’ll figure out— And this is again one of the great things about computer science, is you can divorce the algorithm from the implantation. We can figure out how to implement it efficiently later.
And you may have heard stories about how there was a group associated with IBM that claimed that they had assembled enough computational power to simulate the brain of a cat. This was big news about five years ago. And it’s a bit of a curious claim, but it’s an illustrative one. So it’s sort of like saying you took aluminum and bolts and put them together, and you got an airplane. I don’t particularly want to fly on an airplane if somebody just told me “I’ve assembled enough aluminum and enough bolts to build an airplane.” I’d actually like to see that plane fly.
So, in the case of this cat, if it’s not chasing mice and catching mice, our job sort of isn’t done. And this is really the hard part. So you’ll find people claiming that they’ve built these supercomputers and we can finally simulate brains. The question is what does that brain do? And does that brain actually do the important things and the interesting things that brains can do, or does it just have sort of a virtual seizure. And there’s actually a huge European Union project, a multi-billion euro project, aimed at simulating a huge brain but not necessarily with a whole lot of emphasis on what the brain’s going to do. And there’s differences of opinion about whether that’s a good idea.
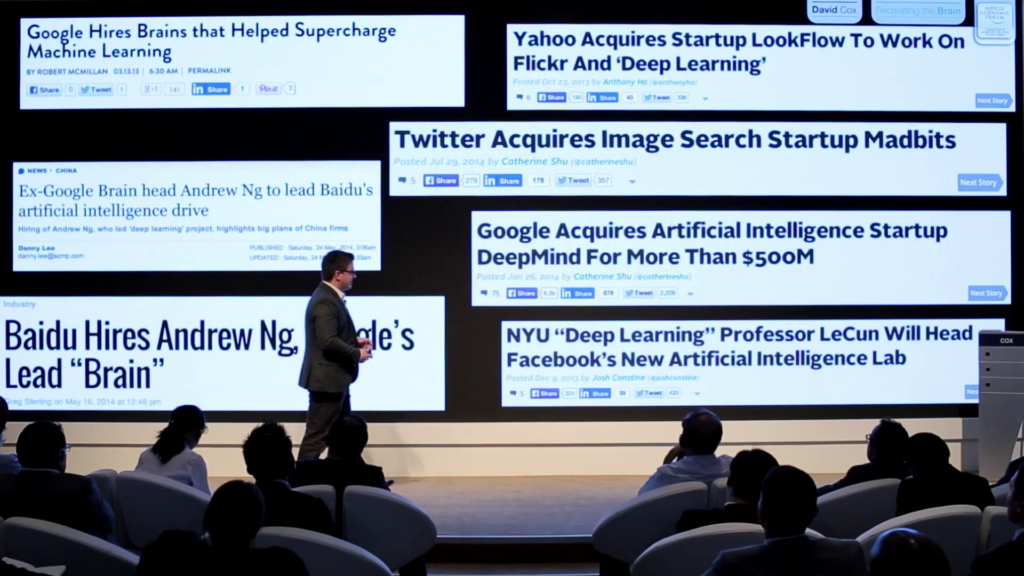
So, this is actually an incredibly ripe time to be in this area. It turns out we’ve been studying these artificial brain-like systems, they’re called artificial neural networks, for a very long time. In fact in the 40s, the first neural network ideas sort of were born, and in the 80s they became a big thing, and then in the 90s they hadn’t quite delivered yet so the whole thing collapsed and there’s something called the AI winter. But today is actually a really sweet time to be in this business, because the systems have gotten pretty good. And you might’ve heard stories like these.
So Google just bought a company called DeepMind for half a billion dollars, and that was entirely based on this technology of building brain-inspired computational systems. And meanwhile Google, and Baidu, and Twitter, and Facebook are basically hiring up huge a huge fraction of the field. Actually, Mark Zuckerberg showed up this past year at one of our field’s major conferences and basically hired everyone in sight. So there are people at Google now who are claiming, sort of back of the envelope, that perhaps 10% of the best people in the field now work for these companies. So it’s an unprecedented privatization of an entire academic field. And at some level that’s at least an indication that some of the smart money at least thinks that there’s some some gas here.
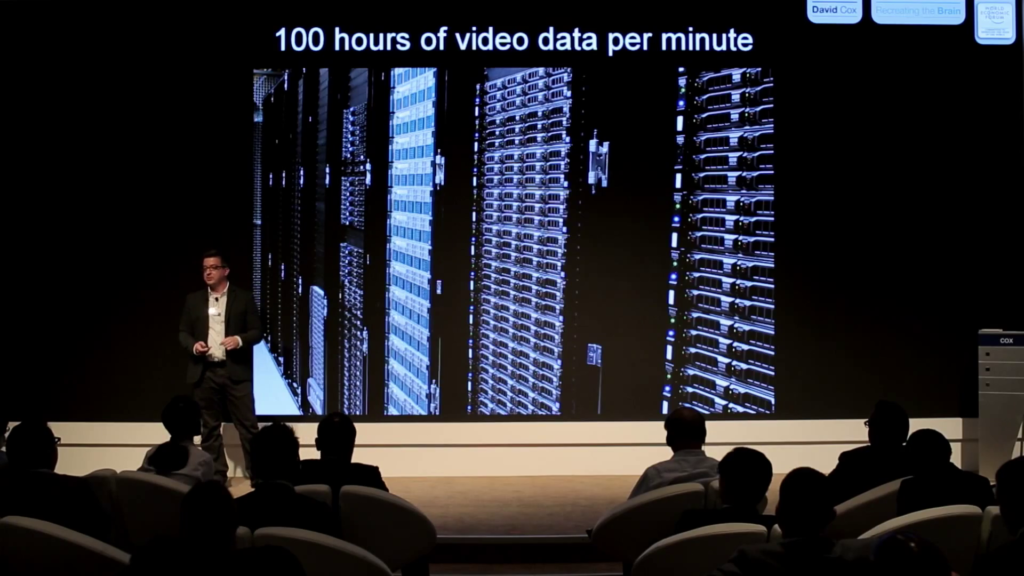
But the interesting thing about it is that this wasn’t really driven by some conceptual advance that happened. It’s much more driven by computational power and the availability of big data. So Google and YouTube alone collect hundreds of hours of video per minute. So that’s just a huge, huge amount of data. And they have the computational resources, they have the server farms, to run it. And in many ways the systems that are now available that Google’s getting so excited about buying and that are winning a lot of these academic benchmarks and challenges over in the academic field, what’s changed isn’t so much that we’ve understood something new about the brain—a lot of our insights were from the 80s. But what’s changed is now we have huge amounts of data.
But at the same time, a lot of the tasks that we’d like to solve, like the Boston Marathon bombing, like that complex street scene, we still aren’t able to do. We aren’t able to do them just with lots and lots of data and just with lots and lots of compute power. We need more information. We need more clues about how the system is organized.
So fortunately, there’s sort of two huge tidal waves that are on a collision course with one another. So on hand, we have all this data, and we have an unprecedented amount of compute power, and we have some real traction where we’re trying to get useful applications coming out of these computer algorithms. But on the other hand, neuroscience is going through an absolute revolution in new tools and techniques. And my lab is a bit unusual in that we try and actually do both. So in addition to building computer algorithms of the sort that Google’s interested in, we also want to go into the brain and look for clues about what we should build next and get data that we can use to constrain the algorithms that we build.
So this is roughly speaking how we reverse engineer a brain. So imagine if you had a competing product that one of your competing companies produced and you didn’t know how it worked but you really wanted to know how it worked, you might buy the product, open it up— There are laws against that in some places but you might do it anyway. You open it up, you put some oscilloscope probes in, and you try to figure out how it works. You reverse engineer the system. And roughly speaking we can do the exact same thing with nature. It just so happens that instead of being a competing product it’s actually usually a warm-blooded furry creature. Or a human.
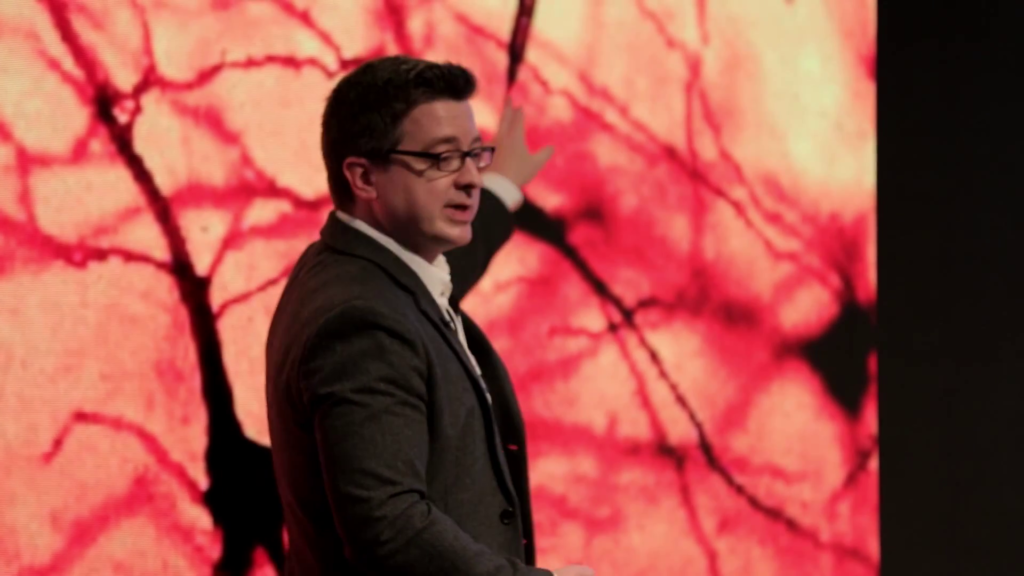
So what we have here are some of the— This is pretty much the earliest technology for reverse engineering the brain. This is a tungsten microelectrode. So this is basically a wire that you can hook up to an amplifier. These are two neurons, and this gets down to about twenty microns, or twenty thousandths of a millimeter at the very tip. And then traditionally what you do is you go and you park an electrode next to a neuron, and you listen to it. You literally put the amplifier to a speaker, and you can listen to the cell. And the way cells communicate with each other is by something called action potentials, or spikes. And they’re little popping noises. So you can actually hear, as you stimulate the cell or you show an image, you can hear a little tak tak tak tak tak of the cell firing, and that’s what this lets you do.
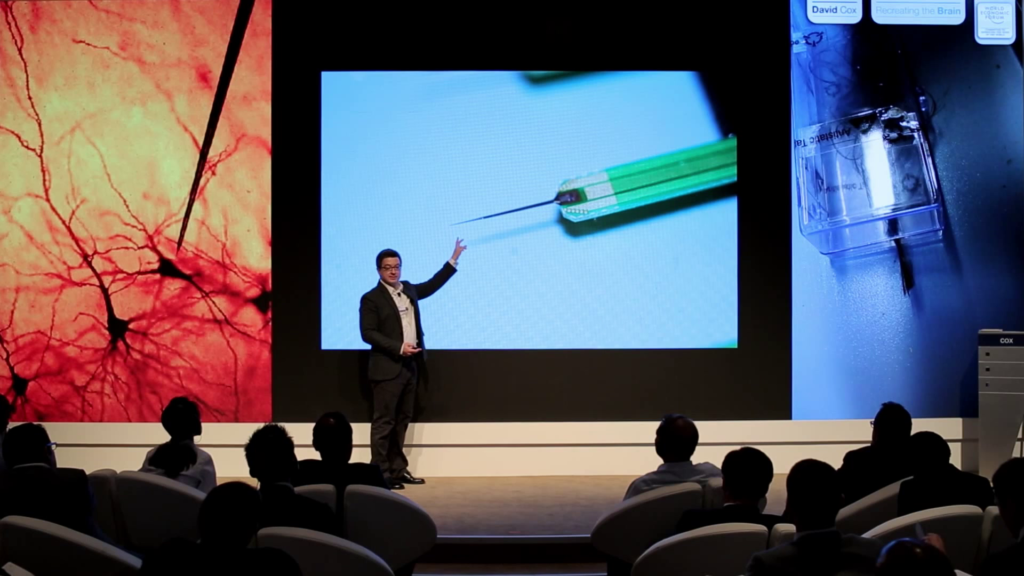
Now, what’s exciting is that through all kinds of innovations in other industries, we now increasingly have access to much better versions of this technology. So this is a kind of electrode array that we use in my laboratory. This is a silicon micromachined electrode array. So it’s got an array. You can see these little dots…are iridium electrode recording pads, so it sort of like one of those except now we can have dozens or hundreds of them. And then this basically sticks into the brain and we can wiretap a much larger number.
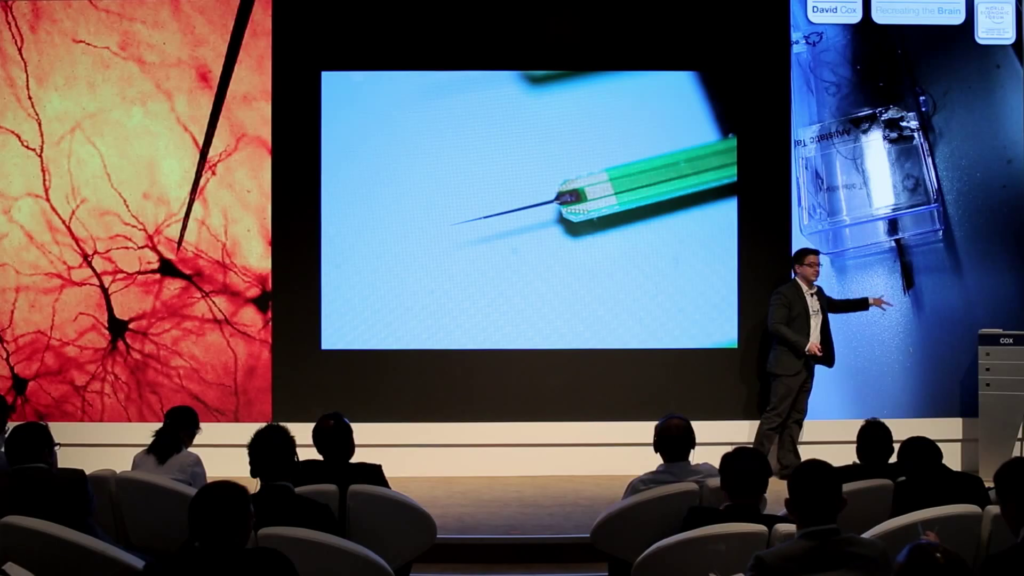
And then this is something new that we’re developing, or starting to use my lab. These are carbon micro wires. So these are each five microns in diameter, or about a twentieth of the width of a human hair. We can get huge numbers of these now into brains, we can snake them in. And then because they’re so flexible, they’re actually almost impossible to see with the naked eye because they’re so small. They can kind of float in the brain. the brain’s always pulsing because there’s blood flowing through it, but these guys can sort of float in the brain and then we can get isolations for a very long period of time.
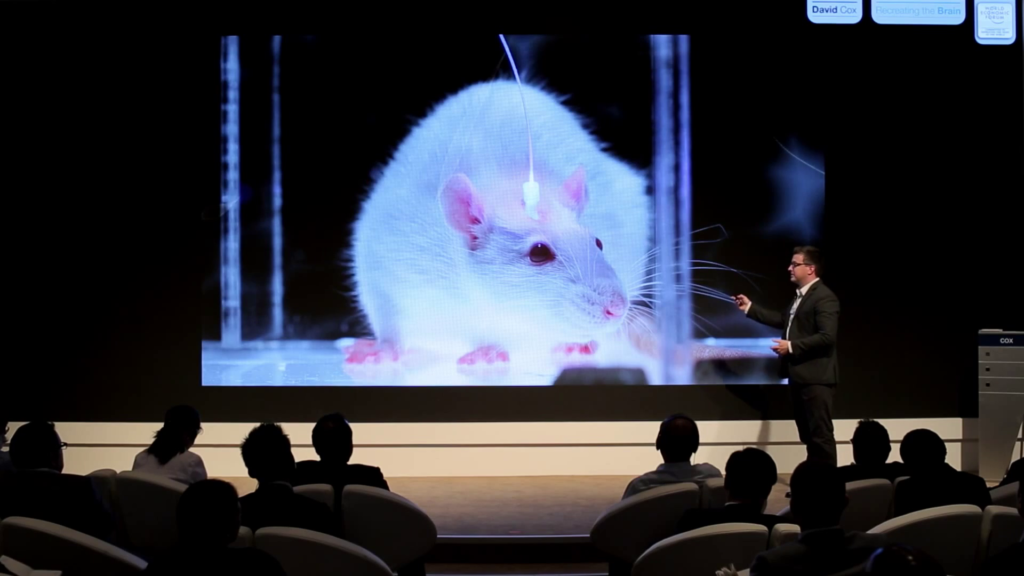
Now, these are the old-school technologies, frankly. That was the updated version of old technologies. But there’s actually quite a number of new, exciting technologies that’re also available. So this picture I just learned was taken by from Feng Zhang who just gave the Betazone presentation. I stole it without knowing it was his, and…anyway, thanks Feng.
So this is an example of optogenetics. So what this is, is particularly to researchers Karl Deisseroth and Ed Boyden at Stanford and MIT respectively, developed a way to introduce ion channels, proteins from other species, and in some cases engineered versions of those proteins from other species, into neurons. And then what this lets you do is it lets you shine light on the cells, and then you can either turn them on or turn them off. So it’s a little bit like installing an on/off switch in neurons. And because these are targeted with genetic technologies, you can target specific kinds of cells. There’s different cell types in the brain. So we can give certain cells a kick, we can turn off certain cells, we can start to manipulate the circuits. So again this is for reverse engineering the brain. These are the kinds of things you want to be able to do. You want to be able to selectively probe different parts of the circuit, and see what happens.
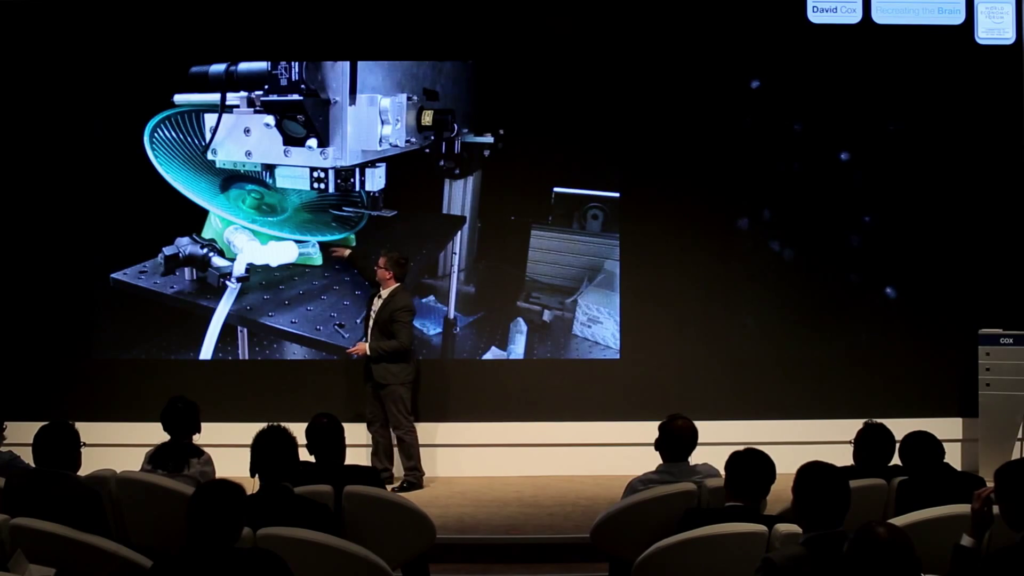
In addition we also have new optical technologies for recording the activity. So I showed you the old-school thing, which was putting an electrode in and measuring the electrical potentials near the cell. But there’s actually quite a few new technologies. So this is a picture of an instrument in my lab. It’s called a two-photon excitation microscope. And what we do…so the rat roughly speaking would go right here. And there’s this little wheel he can run on. And then this is a powerful laser that we shine into his brain.
And the reason shining a laser into the brain works in this case and lets us see activity is because we’ve also introduced a genetically-encoded calcium indicator. So, when cells fire calcium rushes into the cell, and then these genetically-encoded fluorophores will light up when the cell’s active.
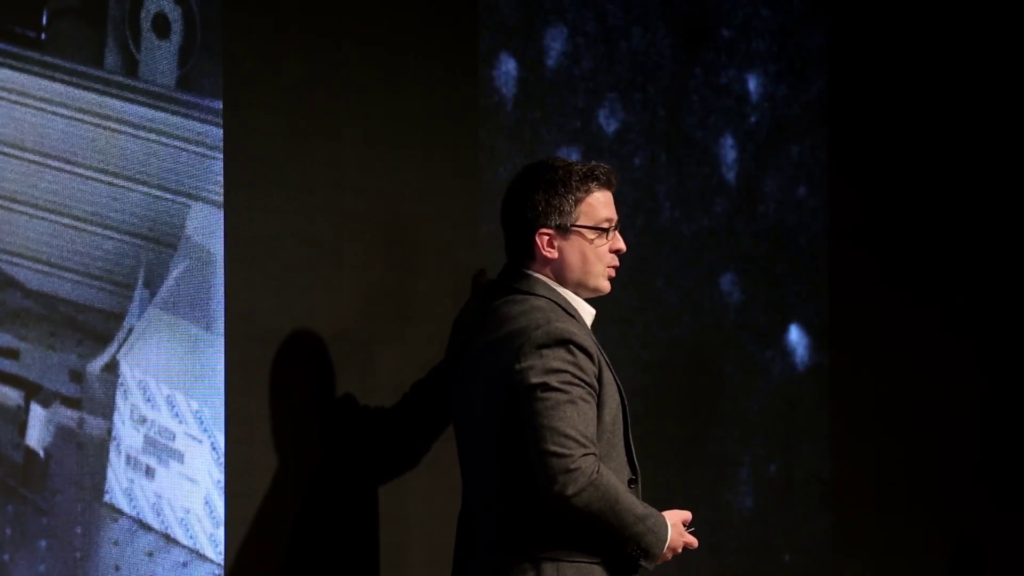
So if you look here you just saw there was a cell that’s sort of glowing. You’re watching right now a series of cells—each one of these round bodies is a cell—in the rat’s visual cortex. And as it lights up and gets dimmer, you’re watching the activity of the cell. So when the cell fires it gets brighter, and we can use this technology to record from hundreds of cells. And critically we can record from the same cells over long periods of time. So you can imagine learning not just how the brain works sort of in its final steady state, but you can also start to study how the brain changes over time. And this is the kind of thing that when we’re building machine learning technologies we really like to see them learning in action.
And then there are other exciting technology. So this is something that’s happening just down the hall from me. So this is Bobby Kasthuri and his advisor Jeff Lichtman. And what we see here is an electron micrograph of a brain. So, what we can do is we can take the brain that I just showed you, where we were watching the activity, we can take the brain out— So this is one thing that’s not great to do in humans but you can do it in rats. And we take the brain out, and we’ve imaged those cells so we know what their activity was like. But then we can actually slice with a very fine knife the brain, and we can look at the very fine structures of the tissue. So this is the example one image, but it’s actually part of a large volume of tissue that’s imaged this way. And the reason you need to use an electron microscope is because these features are actually too small to image with light. So the wavelength of light would actually be something like this. So it’s just, light is too big to interact with how small these things are.
But with an electron microscope, we can slice, and reconstruct, and then we can trace the wiring. So we can literally get the wiring diagram from the very same cells that we were just imaging to get their activity. And this is an incredibly powerful technique that Jeff Lichtman has largely pioneered, and that we’re joining forces to use.
And here’s an example of a single cell’s dendrite, which is the input process. And then all of the other processes connecting onto it. So I told you there were 100 trillion connections. These are the connections to just one of those neurons, in one place. And you can see this incredible complexity of different kinds of stuff that’re connected.
And really this technology is game-changing because it means that we can know everything about how the brain is hooked up. We can segregate out different kinds of processes—axons, which are the outputs; dendrites which are the inputs; also a number of different things like glial cells that are supporting cells; there’s unidentified stuff which I’m fascinated by, but uh…[shrugs]…there you go. So, it’s really an amazing time to be thinking about neuroscience and thinking about how this all fits together.
So the other thing I should mention, a lot of people want to see this work being done in humans, and I think this is actually a mistake. So I mentioned that we’re doing this in rats and, there really is a huge advantage to that. So, imagine you were an alien coming to Earth, and you didn’t know anything about what cars were. You saw these things moving around, but you weren’t quite sure what they were. A sensible thing to do would be to get a car and take it apart and try to figure out how it works.
Now, you could choose my car. So I took a picture of the inside of my car. This is a 2007 Prius. It’s a pretty good car. It’s a complicated car. It’s got a very complicated powertrain, it’s got a motor and an engine. It’s got thirteen computers on board, computer-controlled fuel injection. It’s a great car. It’s a marvel of engineering. But if I were trying to study cars, it might not actually be the right car for me to start with.
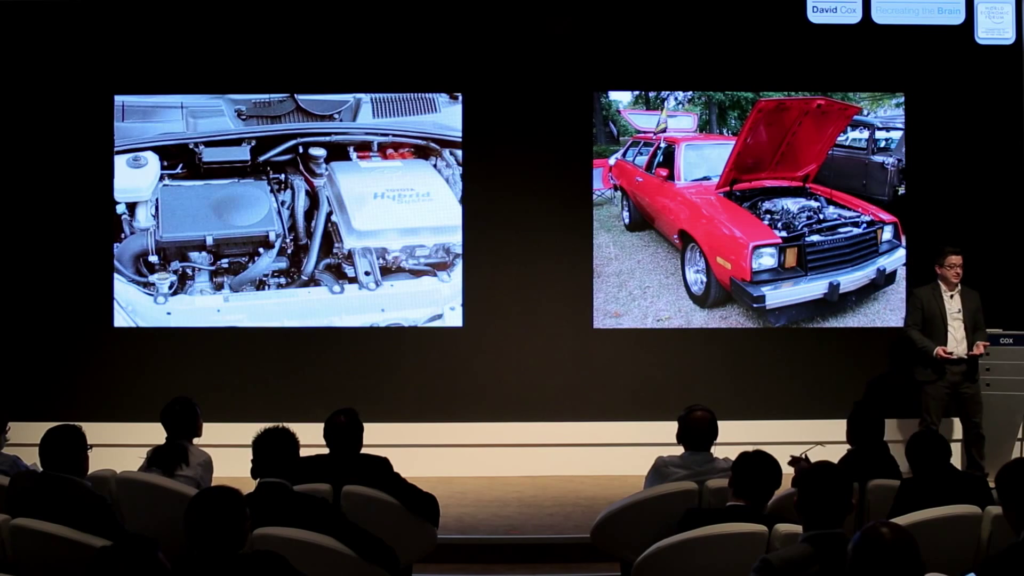
I might prefer to start with the car I learned to drive on, which is a 1980 Ford Pinto. That’s a terrible car. It’s not a good car at all. But it’s got a carburetor, it’s got big parts, it’s got spark plugs. Just because it’s a less-good system…it might be less good at what it does, it’s actually a better system to start with. So this is sort of the training wheels for understanding. And what I’m going to argue is that you know, there’s a drive to study in humans because we’re humans and we need to study the neuroscience of humans. But really we’re not at that stage yet. We’re not quite there yet.
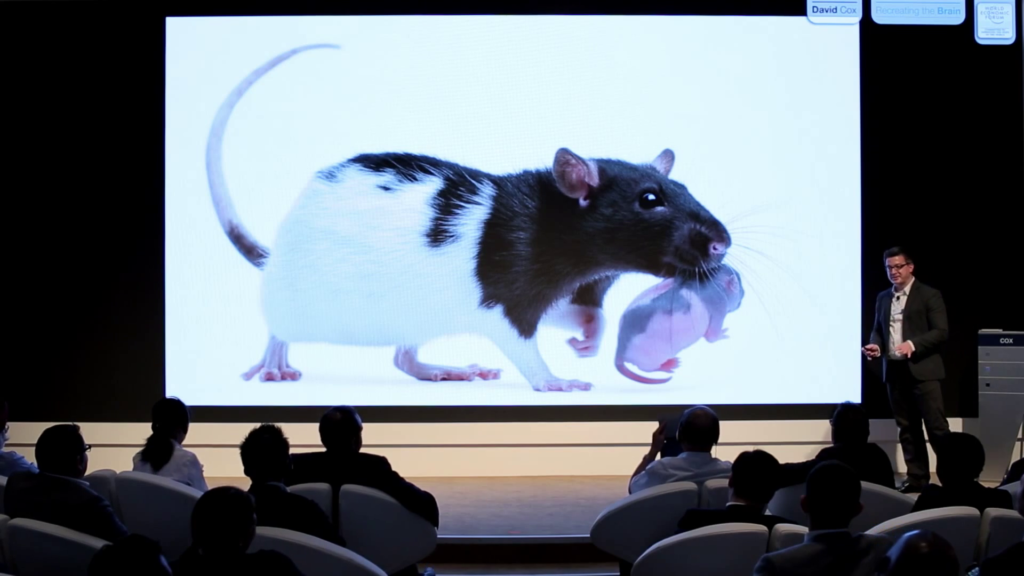
So what we’re going to do instead is we’re gonna find the Ford Pinto of nature, which is the rat. And actually, calling a rat a Ford Pinto is totally not fair, because they don’t explode, and they’re actually quite wonderful at what they do. Nearly every organism on Earth is wonderful at what it does, and if it weren’t wonderful what it does, it would be replaced by some other creature that could do what it needed to do better. But it’s true that their brains are much simpler. Again this idea that this complexity is what makes us different? Like, now we need to dial that back. We need to look at something that’s simpler. And in sheer numbers, the numbers of neurons are much smaller. Where the brain is sort of two pounds of stuff in our head, a rat’s brain is about this big. So when we do things like connectomics, or we do imaging, we can actually start to make some traction.
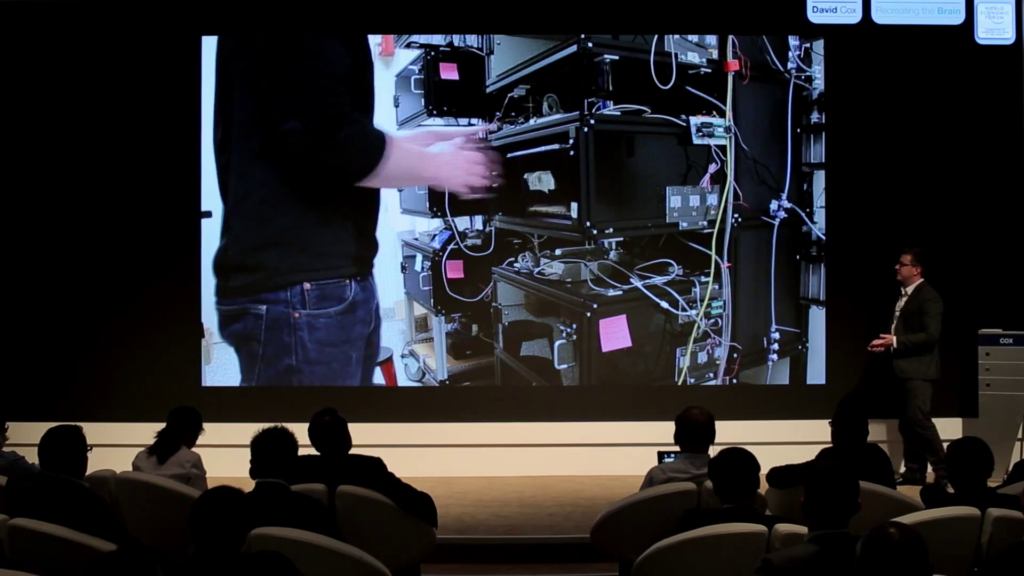
And this is just to show you what my lab looks like. So, because we’re studying neuroscience, which is really the biology of behavior, we’ve built all these rigs to control the behavior. So basically what I’m telling you is I have an army of trained rats that live in my lab. And these are the rigs we train them in. So it’s basically a series of high-throughput boxes. We can take the animals, we can put them in. They basically play little video games. And then we teach them to do stuff so that when then we go and measure their activity in their brain, or we measure changes in the activity of their brain, we can do that with respect to an actual thing that the animal’s doing.
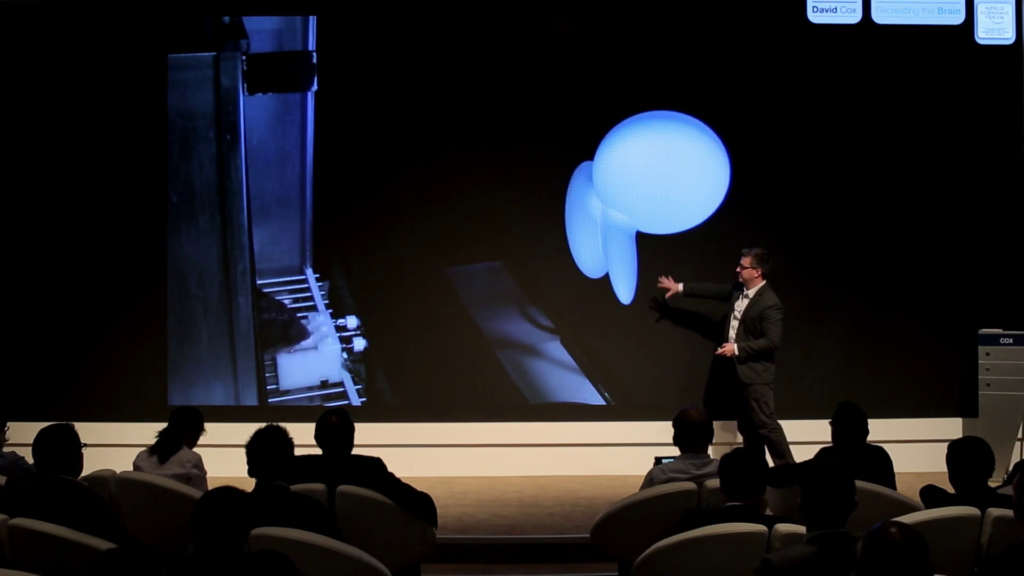
And this is just to give you a sense of what this looks like. So this is a monitor where we’re showing the animal different objects, if you’re interested in object recognition. This is a rat down here. So you can see there he is with his whiskers, and he’s licking. And this is basically PlayStation 4 for rats. This is about as good as it gets.
So, he’s just touching these little capacitive sensors and they put out little bits of juice. And what this lets us do is to have very fine control over the behavior, in a very high-throughput way, and we can mix this with all these technologies and bundle this all up to try and build—he’s adorable, isn’t he?—build up an understanding of how his brain works. [the screen in the training rig flashes several times] He just got one wrong.
So, this is an amazing time to put all this stuff together. And we’re actually starting to assemble a team— I mean, this is an enormous undertaking. So the connectomics alone, if we were to take a millimeter cubed of tissue and slice it up and try and imagine it, that’s one and a half petabytes of data. So an enormous quantity of data to record from all those neurons, an enormous quantity of data, an enormous undertaking, bringing all together all the machine learning expertise that we need to bring together. So what we’re doing now is we’re assembling a team across Harvard and MIT and a few other institutions, basically to do a very serious take on this reverse engineering of the brain, partly driven by interest now from the government. So the Intelligence Advanced Research Projects Administration, which is basically the intelligence version of DARPA, which you may have heard of, is now putting out a challenge for groups like ours to assemble and really take seriously this idea that we can take all of these technologies which are right on the cusp, go to the very frontier of what we’re able to do with them, put all that information together, and really make a front-on push.
This is not an easy task for us to undertake. It’s going to take money. It’s going to take academic cooperation. It’s going to take private corporation. We’re increasingly working with Google now, because Google’s one of the only entities in the world that can deal with this much data time, and we’re collaborating with them now. And we’re going to have to bring that all together to make this push. But the sense that this really is the sort of, the key, the crux of our humanity even if we’re studying it in rodents, really makes it sort of the one of the greatest challenges of our time, sort of to go to the frontier and see if we can figure out how these brain systems work. And then figure out if we can build them ourselves. So with that, I will close. Thank you.