Anne L. Washington So, my colleagues today are going to go against everything I’m talking about, that we’re not talking enough about data science. But we just heard two amazing talks that ground us in a lot of different ways.
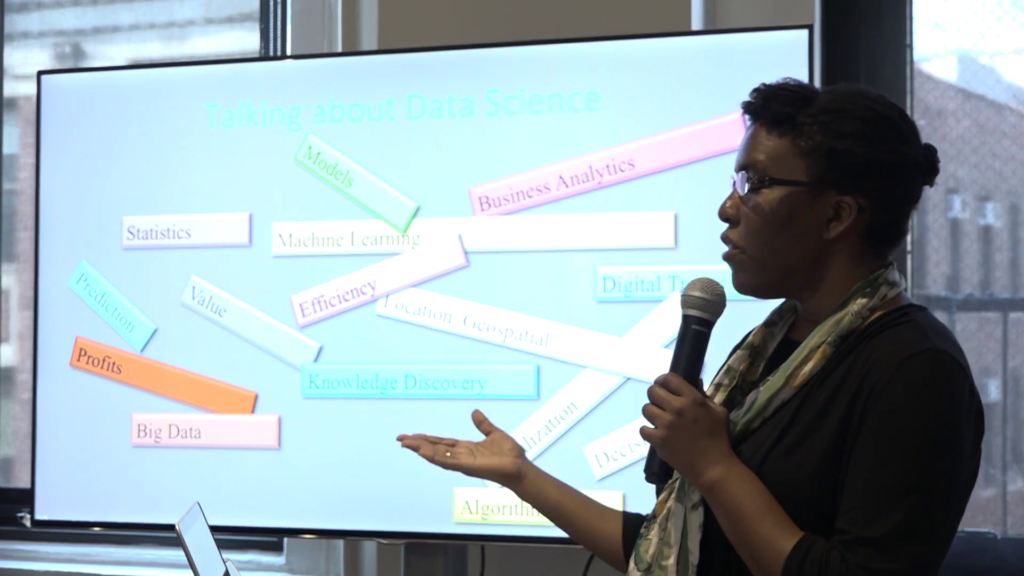
So, people are talking about data science. And usually it’s out there, it’s a big buzzword. Most people approach it from this point of view of computation and computer science, or they’re thinking perhaps of statistics. Now, as a computer scientist who works in policy I’m very aware that these conversations are kind of privileged and technical. Because if we go even farther, maybe we’ll add a few more words but nobody knows what these things are.
And it’s being used in highly important, practical times, in public policy, and I was realizing there is no way for the technical experts to have conversations with policymakers in a way that they could actually understand each other. So during this year that I have been off I’ve been thinking about how to teach both people who are trained in technical parts of data science, and also policymakers, how we could have a common language. And then that way we could have these conversations so we could talk together. And that the point for these data science conversations is to get a level above all the technical jargon and dig into deeply about what is our reasoning for our claims? How can we explain ourselves? And so that way people will know both what the evidence is and then what is supporting.
So let’s get back to thinking about what data science was in the very beginning. Data science was a thing for science. If you imagine what happened for a big sky survey for astronomers, there’s something clicking every moment, taking pictures of the sky. And they teamed together and needed to efficiently understand what they were looking at. So what’s interesting about this example for data science is science. People were all trained similarly, so they came from a disciplinary similarity. They were STEM-aware; they already were aware about science, technology, engineering, and math—a homogeneous group. And so they were just trying to be efficient in what they were doing. It was a pretty straightforward thing.
Well, now data science moved into marketing. And marketing you know, you’re trying to figure out which clients are valuable—very important. And you’re trying to figure out which which customers are which, and how you can make money off of them. But again it’s still a single group. We know the famous case where Target was trying to figure out who was going to have a baby next, and they disrupted some people’s expectations of ethics in that process. And this is what happens when people can’t justify what is happening within the technology. There’s a kind of “ugh factor” that happens when people have to be at the result of it. But still, this is marketing. It was uncomfortable but basically there was no direct harm done.
When you start talking about data science in the public sector, we’re in a different level. So if it’s a marketing problem and I don’t get a coupon, I do get a coupon…like so what, right? But this is different. There always is going to be some constituent in public policy who votes who may or may not have gotten treatment or something that they think is important. The consequences are much bigger, and the politicians and people who work in the public sector have to really think about how they’re going to balance these interests together.
At the same time, in the public sector there’s less money, there’s a great need. And data science brings incredible efficiencies. There’s just no doubt that it brings a lot of benefits into large organizations. But they have to balance that.
So the benefits of data efficiency are just tremendous, right. You can improve your operations. You can figure out your processes. You can predict future or past trends. We know all these things. It can do a lot at once, and that looks really good.
But there are some things that it does that we have to think about differently. Once you have efficiency, you’re creating a clear path towards a specific target. Computers do this beautifully. And computer scientists like me do it beautifully. Like you know, a dog on a bone. Like “let me optimize this,” right. And we’re going to go straight after our target.
What we have to think about are the risks of efficiency, and we saw this beautifully in what the previous speakers were saying. You need to know where you’re going on that road and where you’re not going. You have to think about what you’re optimizing for, and what you’re not optimizing for. There will be second and third place winners as you move through these ideas. And so it’s important to start to think about all of these different things that are going on when we’re talking about data science and public policy.
And that has started to happen. This is the court case— We just heard in Ravi’s talk about criminal justice systems, and my talk touches on that in a little bit. There was the case against Loomis, who had this issue that these scores were used against him and— Well, they were used in his case and he was unable to know exactly what they meant in terms of his case.
At the same time, in the summer of 2016 the US Congress was considering whether these types of scores should be used in federal prison, and there was a lot of talk about that. And in comes ProPublica. So, ProPublica came into this debate and did a very important article talking about whether these scores were fair or not. And fair in all the beautiful ways Suchana already demonstrated to you. There are lots of ways to think about fairness.
Now, what it was that was at issue for ProPublica was fairly simple, and this gets back also to what Ravi was talking about. Who needs services? And how do you understand that? So let’s just take a hypothetical, because you’re thinking about public safety. So let’s say that Mr. Elliot who lives in EliteTown gets arrested. Now, Mr. Smith who lives in the Sad City also gets arrested.
Now, they’re going to look at these scores and try to figure out who might need more services. Now, it turns out EliteTown has a lot of social services and it’s really taken care of. So the risk score for Mr. Elliott might be very low. Whereas Sad City doesn’t have a lot going on, and there might be a greater need for Mr. Smith to have services because they’re not in his community. So he might get adjusted differently for that.
Now, what happens is when the needs assessment gets used as a risk assessment, and now suddenly Mr. Smith in Sad City is seen as a higher risk. Now, in the case of the ProPublica article, what they found was indeed Mr. Elliott who lived in EliteTown— (Those aren’t their real names.) Mr. Elliot who lived in EliteTown actually had a five-year record in a different state. And Mr. Smith never committed another crime. But Mr. Smith had the higher risk score. So you start to see that once you start breaking down the reasoning of how these things are built out, you can understand it in some different ways.
What was fascinating about this argument about COMPAS scores between NorthPointe and ProPublica is that ProPublica said, “Ding!” Northpointe said, “Dong!” And they went back and forth. What was fascinating is that data scientists chose to write about it. This is just a few of the articles that I’ve been reading this year. A new one comes out about every six weeks. And they are having an argument about how do we understand the same data set when we look at it differently? What’s important to us? What are our arguments? All around that, but they are using the exact same data. And this got me to think about how we can start having these conversations between each other, looking at the reasoning that I read in the COMPAS articles.
So, one intervention for this is to imagine reasoning about the populations that are at risk. Who might win? Efficiency will always tell you who will win. In the ProPublica case it was very clear—everybody agreed—the algorithm found violent offenders who are likely to reoffend. That was no doubt. But who might lose? This is where ProPublica argued that there were certain populations that would lose, and it was this idea of understanding who was less likely to survive from that.
So part of this is thinking about reasoning about priorities, right. The primary priority of Northpointe— They were hired, as we have heard from several of my colleagues. They were hired to build a system that did one thing. And they were like, “We did it. We did the one thing.” There’s no doubt that’s true. But there are secondary priorities that have to be taken care of. And those priorities can be a variety of things. One might be the law, for instance. Or public policy. Or regulation. And the reason I say that is that a lot of these tools are sold for multiple industries, and therefore they might really not know what are the subtleties of regulation in a particular industry.
It’s also important to consider reasoning about impact. Most efficiency algorithms are going to go straight to an immediate gain in efficiency for a particular operation. People are saving money. Everyone’s happy. There’s some bottom line argument that’s pretty immediate. When we’re talking about risks overall, the conversation that needs to happen is about long-term impacts or social impacts, and understanding this balance between different groups.
So as I create this curriculum development that could be used in other places, the point of this is to begin to start a conversation about data science, and a conversation where everyone can participate and it’s not just people who know certain skill sets. Really to understand who can win, who’s at risk, how do we think about the long-term impacts, and have this as an integrated experience so that way we can all come to better solutions for data science that will impact both our society and our governing. Thank you.
Further Reference
How We Analyzed the COMPAS Recidivism Algorithm, ProPublica
Papers cited in presentation slide at ~8:00:
- False Positives, False Negatives, and False Analyses: A Rejoinder to Machine Bias: There’s Software Used across the Country to Predict Future Criminals. And It’s Biased against Blacks, Flores (2016)
- Inherent Trade-Offs in the Fair Determination of Risk Scores, Kleinberg (2016)
- Fair prediction with disparate impact: A study of bias in recidivism prediction instruments, Chouldechova (2017)
- A Convex Framework for Fair Regression, Berk (2017)
- Identifying Significant Predictive Bias in Classifiers, Zhang (2016)