Dan Taber: So on behalf of my group I’m extremely excited to be presenting our product, which is known as AI Blindspot. I actually wanted to start by just reflecting a little bit. Looking back on March, we started this program in the middle of March with an initial two weeks. And right after that, there was an amazing flurry of activity, where major tech companies were making all these bold declarations of major…statements that they were making of what they were doing to address AI ethics.
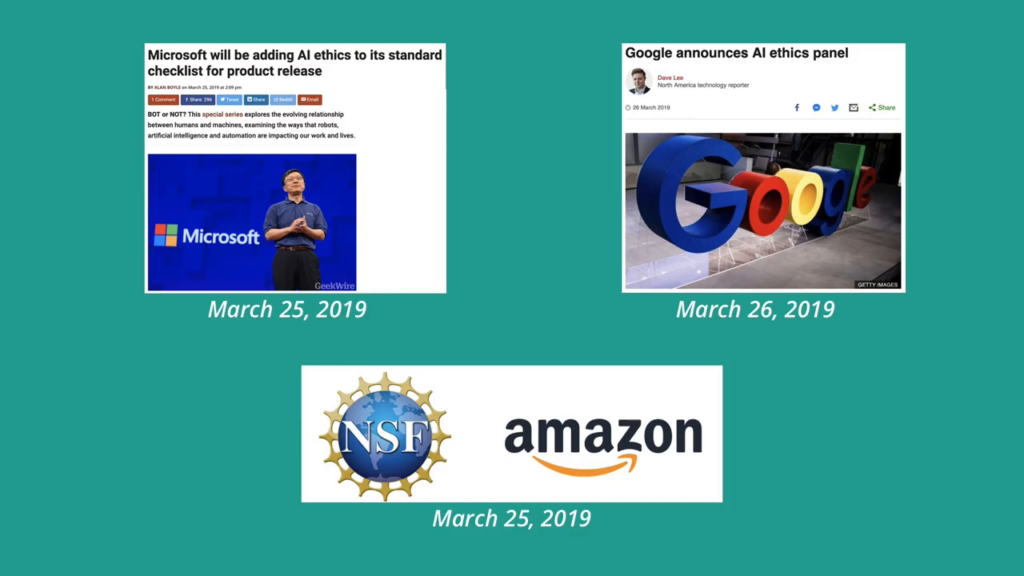
In the span of two days, you had Microsoft announcing they were going to add an ethics checklist to their product release. You had Google announcing their now-infamous ethics panel. You had Amazon announcing a partnership with National Science Foundation to study AI fairness.
But, as I was watching these headlines come out, you got the sense that this is what was really going on:
Because right about the same time, I was starting to have conversations with several tech companies, with people who were involved in ethics initiatives, where even ones who were really trying to do their best to implement changes to study bias in AI systems, and…frankly a lot of them just didn’t know what they were doing, as they openly admitted. Because there were just no structures or processes for assessing bias in AI systems. There were a lot of tools that had come out in the past year, including those that came out of Assembly, like the Data Nutrition Label and others like Model Cards for Model Reporting from Google. But you need both schools and you need structures and processes. And the structures and processes are what we decided to address with AI Blindspot.
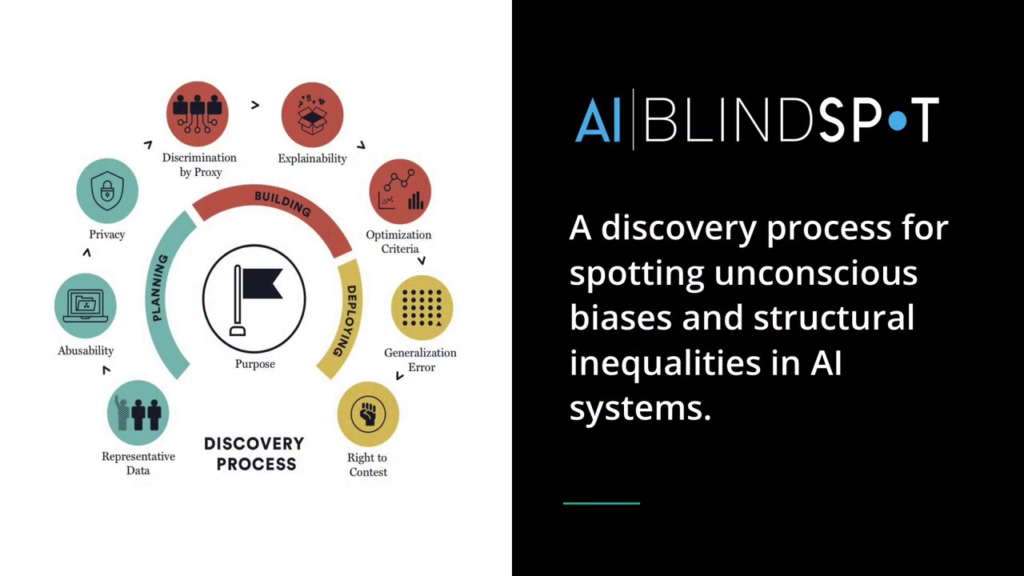
AI Blindspot is a discovery process for spotting unconscious biases and structural inequalities in AI systems. There are a total of nine blindspots—and when I say blindspot I’m referring to oversights that can happen in a team’s natural day-to-day operations during the course of planning, building, and deploying AI systems.
There are a total of nine blindspots you see in this diagram on the left. It all starts with purpose right in the middle, because everything in AI systems should always come back to a purpose and what it’s being designed for. And then if you start at the lower left and go clockwise around, the other blindspots are representative data, abusability, privacy, discrimination by proxy, explainability, optimization criteria, generalization error, and right to contest. And again, these are all cases where oversights can lead to bias in AI systems that in most cases are gonna harm vulnerable populations due to unintended consequences.
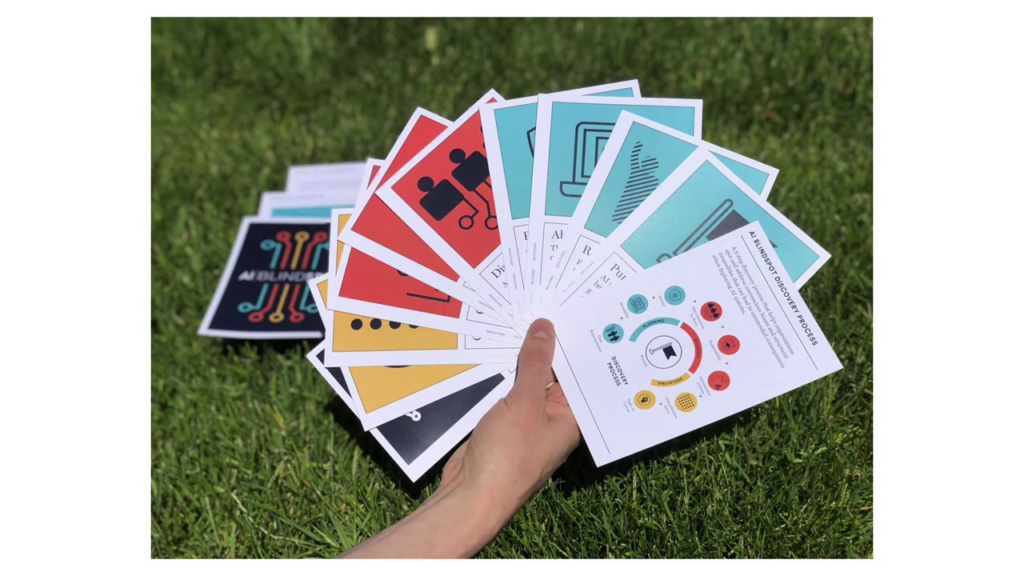
But AI Blindspot, it’s not just a fancy diagram with lots of nice colors. We wanted to turn it into an actual tool that teams could use. So we created these blindspot cards. And we created these because we wanted to design something that was a little bit more accessible. There are also a lot of impact assessment tools that are coming out, and I can say from personal experience that they’re pretty cold and technical. We wanted to create something that was a little bit more light and accessible, that teams would be a little bit less intimidated by.
By the way, this photograph is courtesy of our professional photographer Jeff and our professional hand model Ania.
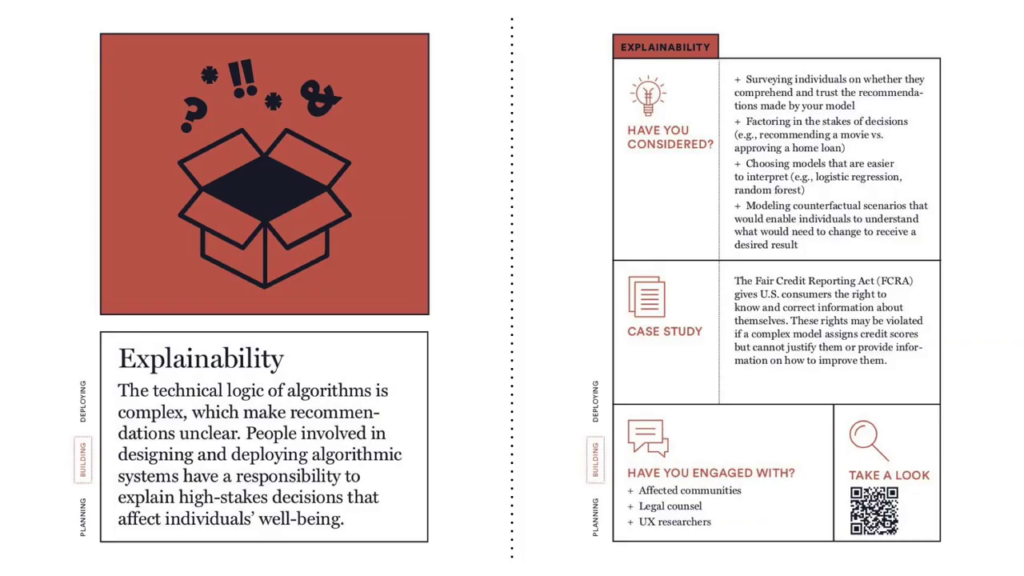
I’ll walk you through the layout of the cards. The left represents the front side of the card, and the right represent the back side. So it starts in the front with a description of what this blindspot is, doing our best to phrase it in non-technical language so we can reach different audiences.
And then on the back side, we have a “have you considered?” section that talks about some of the steps you can take to address this blindspot. So in the case of explainability examples could include surveying individuals—users—on whether they actually trust recommendations made by your AI system; considering different types of models that are maybe more explainable than others; factoring in the stakes of the decision—are you just recommending a movie to somebody or are you deciding whether somebody’s going to get a home loan or not? And then potentially modeling counterfactual scenarios that enable people to see what would have to change in order to achieve a more desired outcome.
And then we provide a case study to give a real-world example of where this blindspot arises and potentially—or in many cases has harmed vulnerable populations due to oversights the companies made.
And then there’s the “have you engaged with?” section that highlights specific people or organizations that you may want to consult with due to their expertise, either within your own company or organization, or outside.
And then there’s a “take a look” section that provides a QR code that’ll take you to different resources that’ll help you address this blindspot.
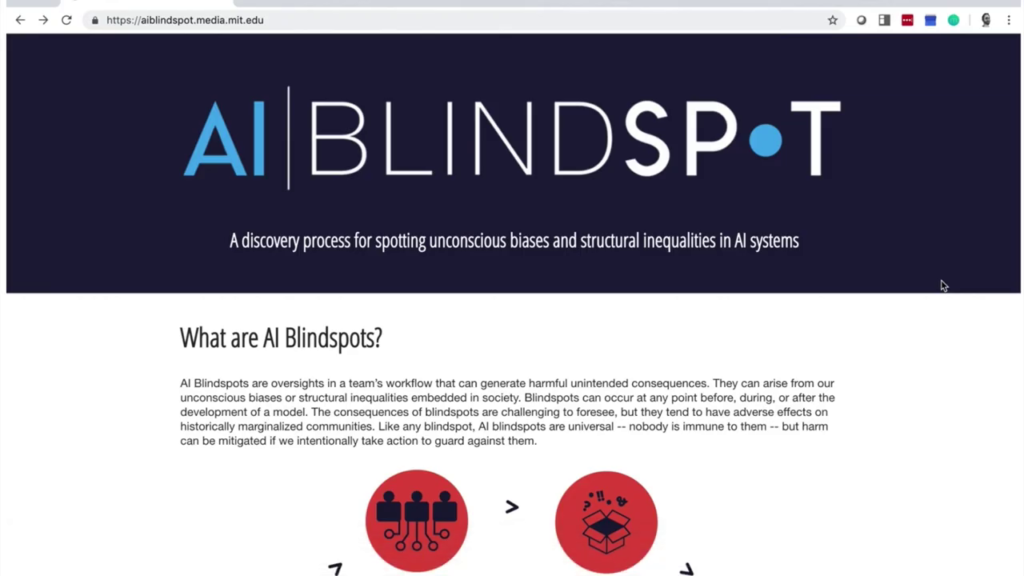
And then this shows our web site that… It’s amazing actually that this video I recorded this morning, it’s now out of date because Jeff keeps making so many changes to the web site. But it shows the cards, shows Ania’s hands, and then enables users to just explore the different blindspot cards. And if you click on one, like explainability here, it’ll show you the same content of the card, and it’ll show you the actual resources that’re behind that QR code. You can take the link to different places to learn more about this blindspot or how to address it.
And then a “what is missing?” button, where you can provide suggestions. It’s a good thing we added this, because we’ve actually gotten feedback already. We got our first feedback from somebody at the University of Washington who I think mostly had good things to say, fortunately.
So with that we want to give an example of a case study of how this could be applied in the real world. this is a semi-fictional case study. It may or may not have been informed by an actual incident that happened at a major tech company I may have mentioned earlier in the presentation.
But, so hypothetically let’s say there’s a tech company that has a lot of internal data on their historical hiring practices. And so they want to use AI to identify candidates for software engineering jobs. So they go their data science team and they say, “Okay, we want you to build a model that’ll help us screen through resumes so we can fill these software engineering jobs.”
So the data science team does that. They build a model, they deploy it. But then they realize that they’re just getting white men being recommended. So what happened there? And more specifically how could AI Blindspot have prevented this? So I’m going to give examples of one card from each of the three stages, the planning, building, and deploying stage.
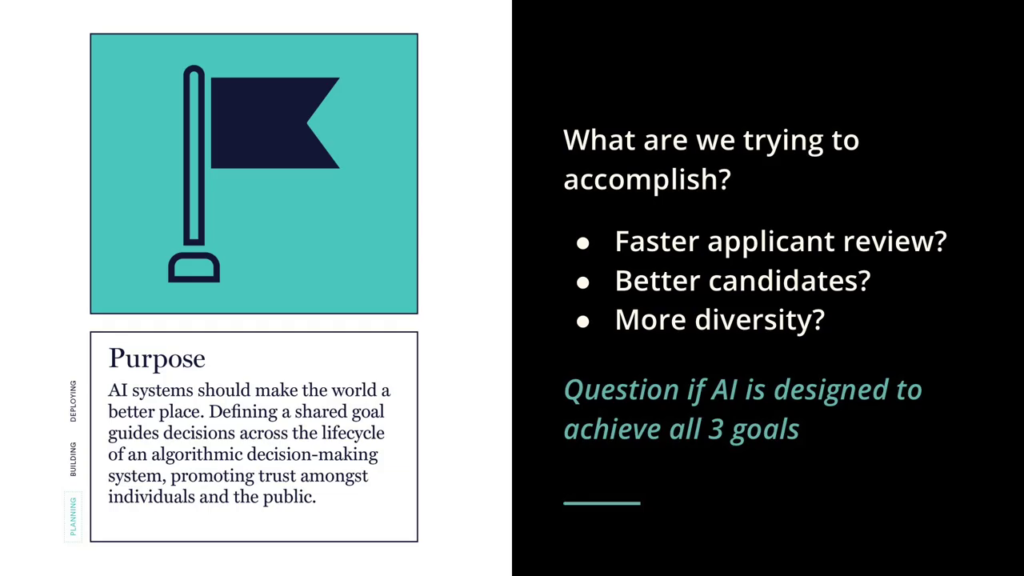
Again, it all starts with purpose and really asking yourself what are we trying to accomplish here? This would involve talking to the team about why do you want to use AI? Like are you just trying to get through resumes faster? Or are you trying to identify better candidates? Or are you trying to increase diversity? And then really asking yourself is AI really designed to achieve all those three goals.
If you just want to get through resumes as fast as possible then AI may be able to help you with that. But if you want to identify better candidates, you would have to question your historical hiring practices. And certainly if you want to increase diversity AI may not be the right tool for that so we would encourage seems to really question if AI is even suited to their purpose. But in this case let’s say that the team says okay, it’s number two. We really want the best candidates, and we really think AI can do that.
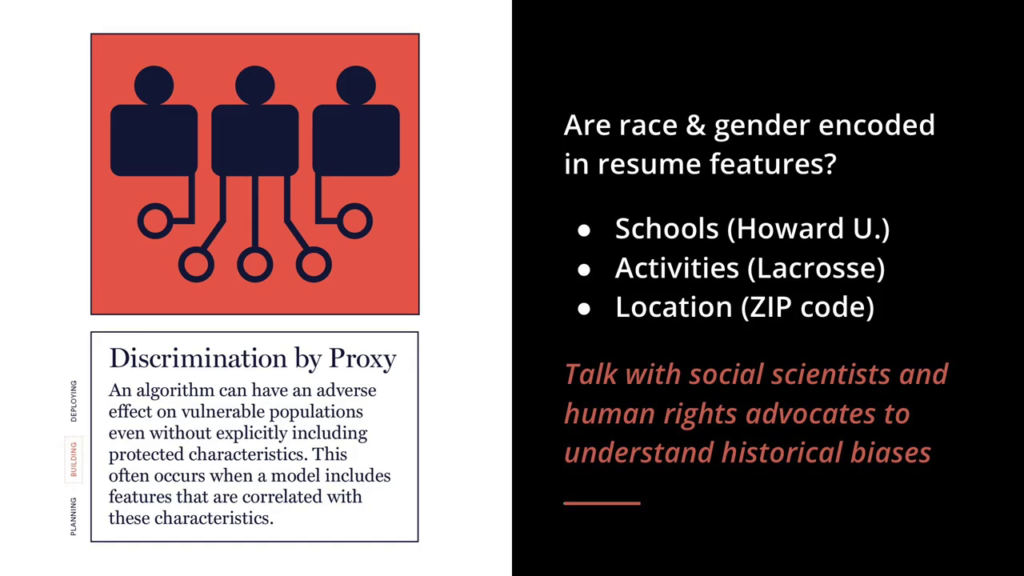
So we move on to the building stage and then address the issue of discrimination by proxy. That refers to situations where you may not include features like race or gender or other protected classes in your model, but you may have other features that are so highly correlated with race or gender, such as historically black colleges or all-women’s colleges, or sports lacrosse that white men are more drawn to. And features that are so correlated that it ultimately leads to discrimination. And we would encourage the team to consult with social scientists or human rights advocates who are just more knowledgeable about historical biases and can help you identify certain features that may be problematic that could lead to discrimination.
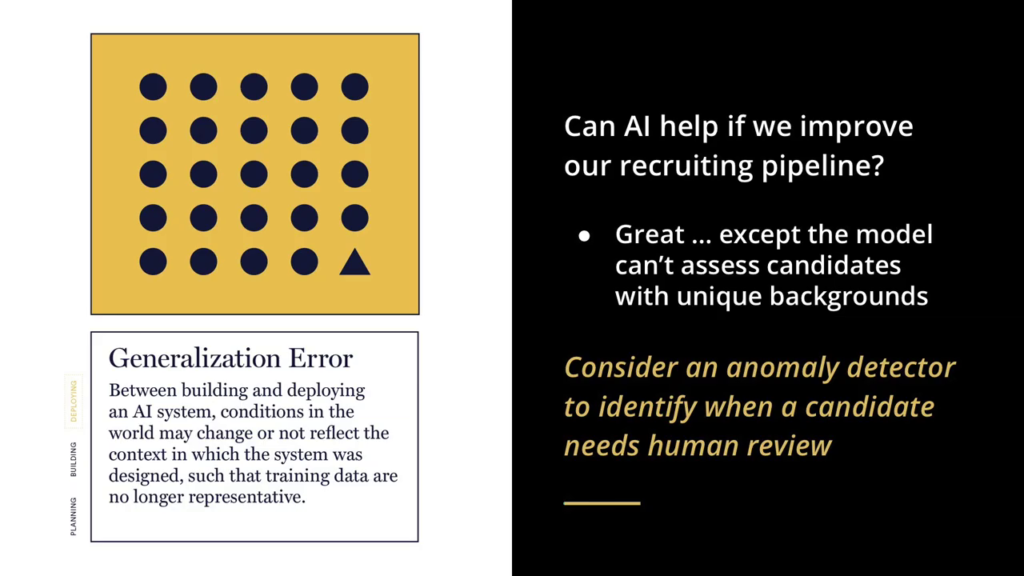
So let’s say the team has done that and now we move on to the deploying stage. And in this case I’m even going to give the company the benefit of the doubt and say that they actually want to increase diversity and they realize that AI can’t do that, so they realize they have to go back and fix their recruiting pipeline first by getting more diverse candidate pools. And then maybe they think okay now AI can help us increase diversity.
But that’s not actually the case because that brings up the issue of generalization error, where if you have a history of not recruiting diverse candidates and now you do recruit diverse candidates, the model that was built on historical data is not going to be set up to evaluate new candidates with different backgrounds. So you’d have to consider something like maybe an anomaly detector that enables you to identify circumstances like candidates that have more unique backgrounds, where AI’s just not suited to or where you do need a human to review.
These are just suggestions but this gives you some ideas of ways that teams could work through the planning, building, and deployment of AI systems to identify their blindspots and brainstorm how to address them.
So with that said what’s next for us as a team? We have a lot of ideas of potential use cases for this, some of which we got from peers in our cohort. I can see a lot of potential uses in different settings such as a product manager leading design sprint, and seeing potential use of the blindspot cards to help through the design thinking process.
It could be a new Director of Data Science at the start up where there aren’t really structures or processes for how data scientists go about their job, and blindspot cards could potentially help guide data scientists work.
On the other hand it could be a city task force that’s responsible for auditing AI systems but has a less technical background, and similarly needs some sort of guidance on what blindspots to be looking for.
An there could be other potential uses as well. So our plan for next steps is to engage with users, doing user studies to figure out what the best audiences were, and then hopefully getting testimonials from organizations where the blindspot cards have been helpful in helping them assess bias in their AI systems.
And then in the grand scheme hoping that this could be a part of some certification process through an organization like IEEE, possibly setting up some standards where say if an organization has processes like AI Blindspot combined with tools like the Data Nutrition Label, they could certify themselves as using AI responsibly. That’s kind of our long-term goals that won’t happen anytime soon, but we see a lot of potential for what this could do.
But we wanted to close with the joker card. That’s one of the blindspot cards. All of you, by the way, should pick up a set of blindspot cards. We have them at our table outside. But the joker card kind represents the idea of the unknown unknowns. You know, we’ve identified these nine blind spots but there are other blindspots too that we probably didn’t think of. We’ve identified potential use cases but there may be other ones that we haven’t thought of yet that maybe some of you in the audience think up. So, definitely come talk to us if you have ideas for where and how this could be applied, because we really see potential to kind of help those organizations I was talking about at the beginning that really want to evaluate their systems for bias as best they can and just don’t know how to do it. So with that, I thank you very much.