The human brain is the next topic of discussion. The brain is much like a computer, but it is a computer that works by very different principles from say, the computer that runs your cell phone. That computer, which was designed by people, has very separate hardware and software stacks. They’re designed to be as independent as possible, so that the phone can run any software.
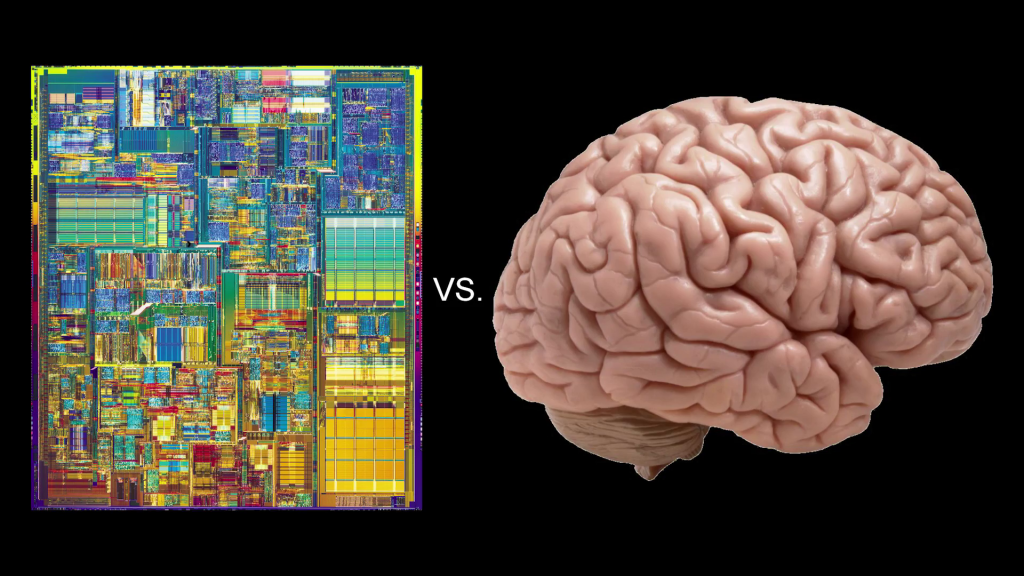
The human brain works by the opposite principle. The hardware and the software are intimately interlinked. Now, there are about, we estimate based on animal studies, five hundred distinct brain modules in the human brain, and these are wired together in a complicated, interconnected network.
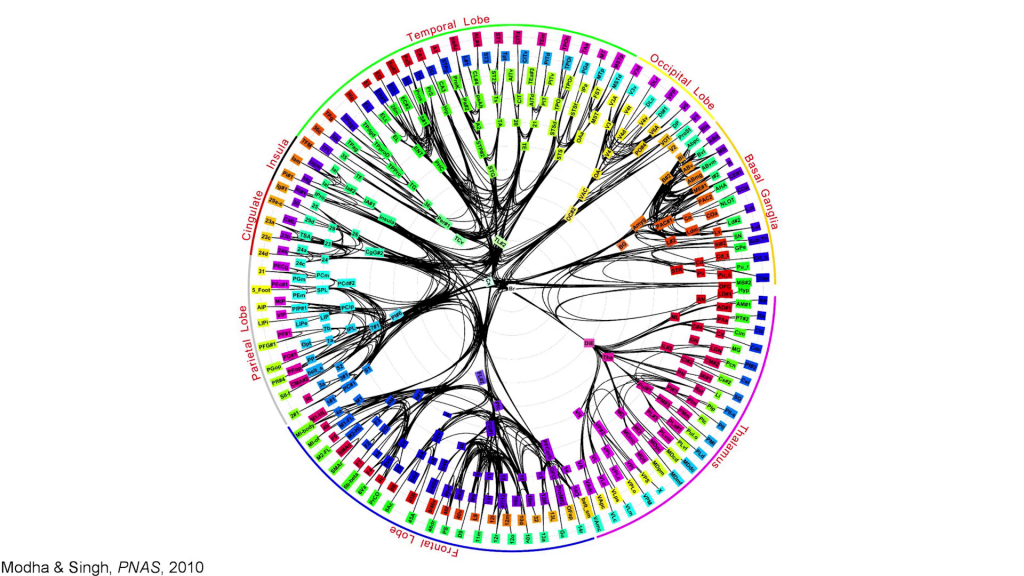
And on a short time scale, the network is fairly fixed, and your fleeting thoughts all arise by interactions of information through this network. Each individual brain area is itself fairly complicated. If we look at the visual cortex of an animal, we find that there is a map of visual space in the visual cortex, and on top of that there are say ten or twenty other visually-relevant dimensions represented in that same brain area. So if we have five hundred brain areas each representing twenty dimensions, we have ten thousand dimensions represented in the brain.
Now, people have known for over a thousand years that brain function was probably somewhat localized. But until recently we had no way to actually measure the brain in living humans. So most of our conceptions about brain function and brain anatomy were based mostly on superstition.
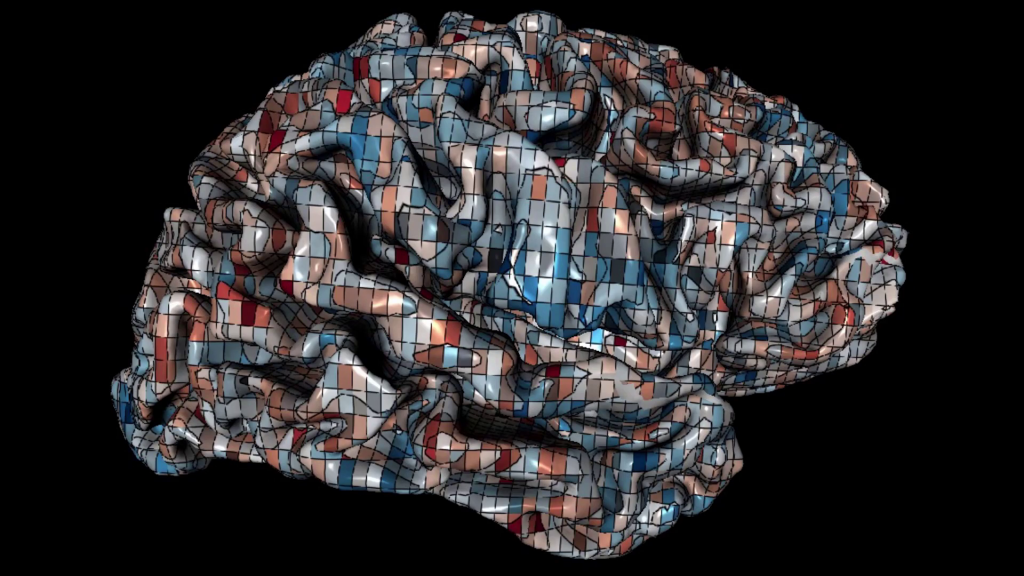
But about twenty years ago there was a new method developed for measuring metabolic activity in the brain that is associated with neural activity, and that’s called MRI or functional MRI. An MRI measures brain activity in small volumetric units about the size of a pea called voxels. And you can measure these metabolic units all over the brain, and you can use this therefore to map brain activity, as shown here.
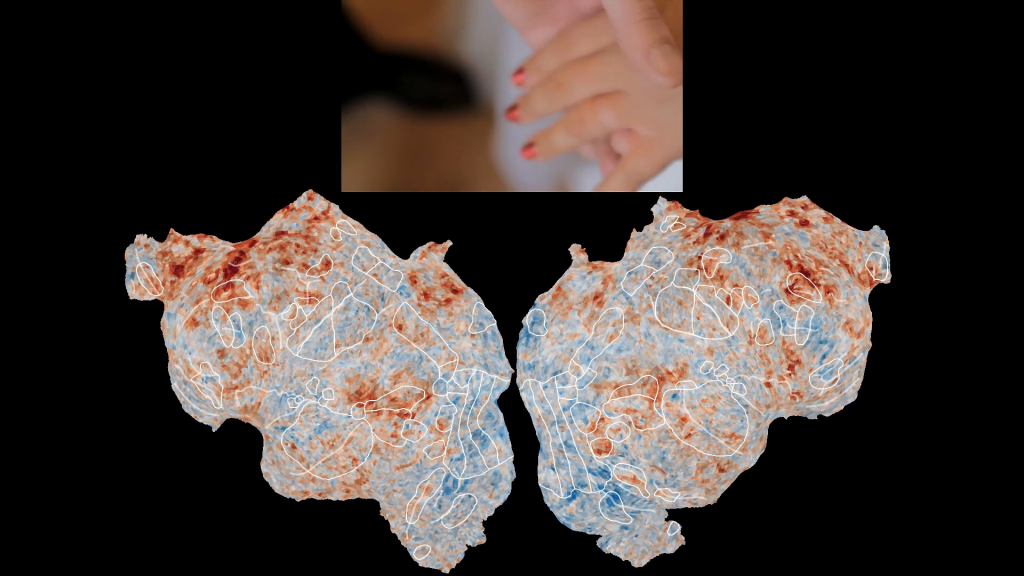
Still from Jack Gallant, “Human Brain Activity Elicited By Natural Movies With Sound”; during this section, Gallant plays a 15-second portion from ~2:00
This is the brain of one human subject watching a movie. We inflate the brain and we flatten it out so you can see the entire cortical surface, and we’re painting brain activity on the cortical surface as this subject watches the movie. And you can see that these patterns are dynamic and complicated and constantly shifting.
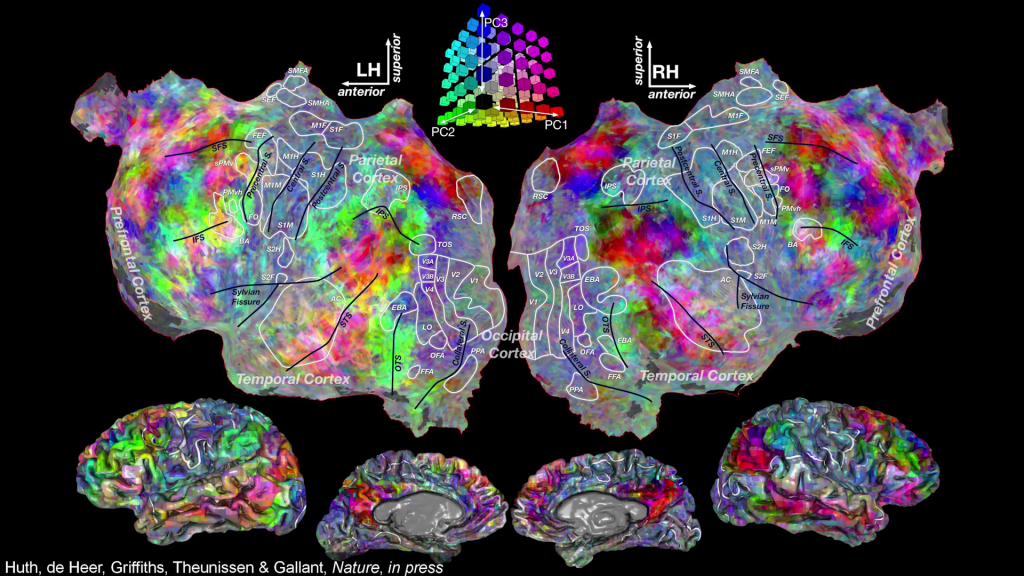
Now, if we do a controlled experiment, for example we have people listen to narrative speech, we can actually pull features out of the speech and we can look to see where those features are represented in the brain. So for example, green on this map shows where semantic information about speech, the meaning of speech, is represented in the brain. And you can see that the meaning of language is represented in wide swaths of the brain.
We can drill down into the semantic model and color each voxel according to its semantic selectivity. So the colors on these maps indicate different kinds of semantic concepts. Animals, vehicles, tools, anything you can think of. Social interactions, they’re represented in language, and you can see that these maps are fairly rich and complicated.
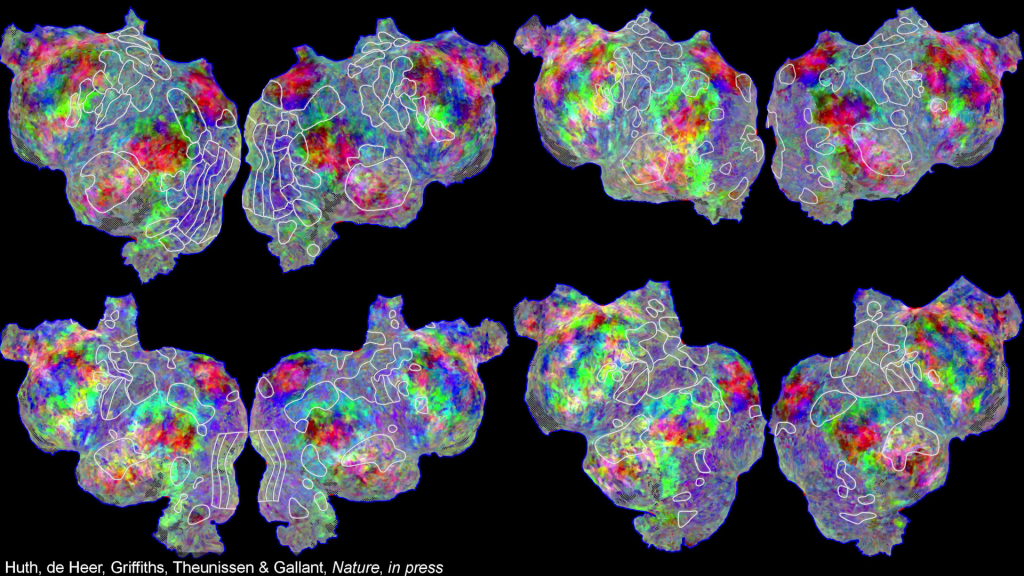
We can do this mapping in individual subjects, so we can recover these semantic maps in each individual subject. You can see that the motifs are somewhat comparable across subjects, but there are also substantial individual differences.
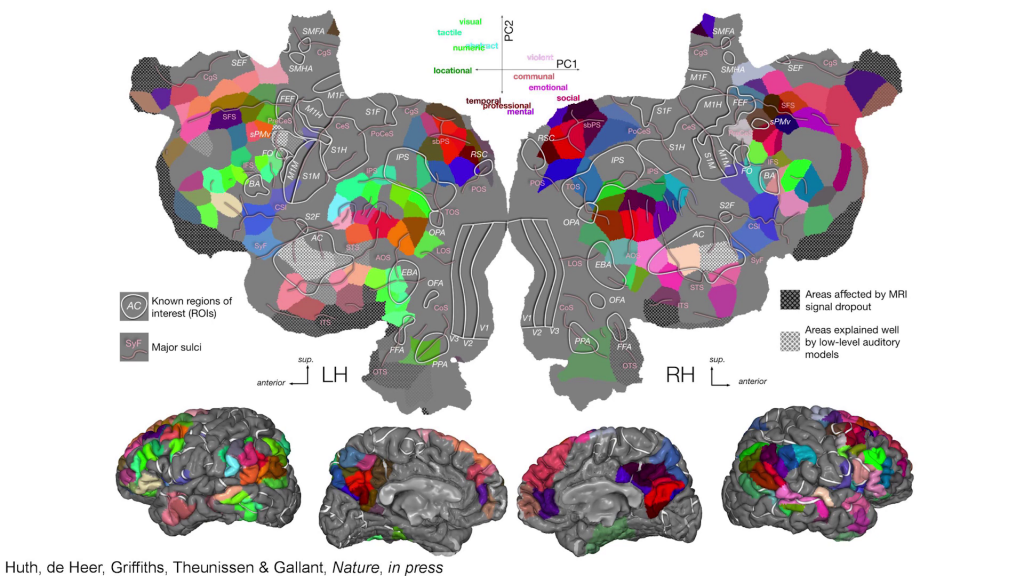
And if we collect data from a group of say, seven or ten subjects, we can use modern machine learning and generative modeling methods to recover an atlas of semantics selectivity. And this atlas shows that there are about two hundred distinct brain areas that’re involved in representing the meaning of language. And these areas are shared across all humans, but their exact position and size differs in different individuals.
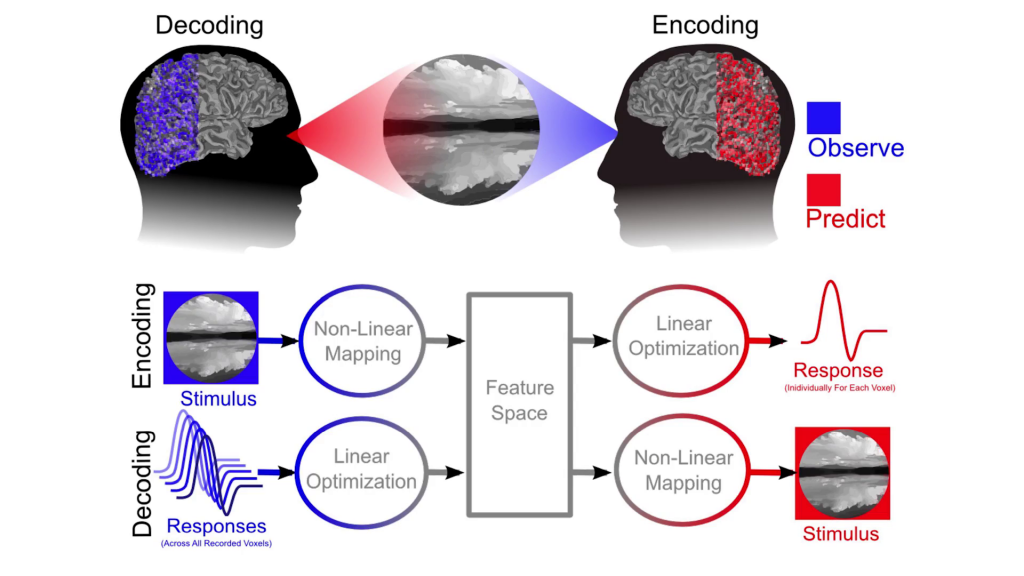
Now, to show you how powerful this atlas approach is, we can do brain decoding. And in brain decoding, we take our model that we’ve developed of the brain (and this can be a model for anything, vision or language) and we reverse it. And instead of going from the stimulus to the brain activity, we go from the brain activity back to the stimulus. And we actually decode the information represented in a given brain area. So we can build one brain decoder that represents or recovers the information in each brain area.
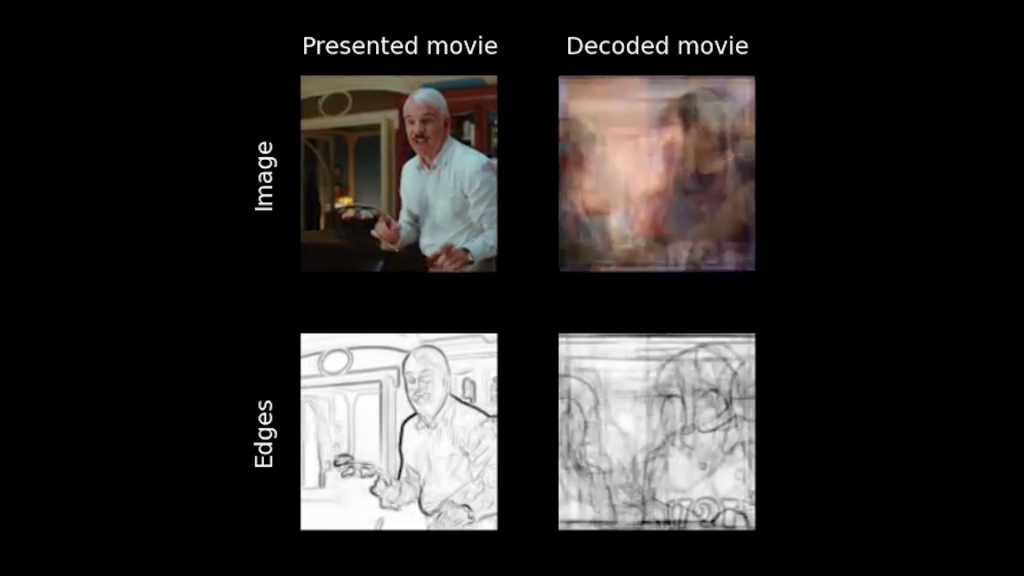
So, this is a decoder built for [the] primary visual cortex. On the upper left is the movie that subjects saw. On the upper right is the decoded result. Primary visual cortex represents the structural elements in movies, like the local edges and textures. And you can see that this decoder does a fairly good job of recovering the structural information, even though we’re decoding one brain area.
This is a decoder that is recovering information from higher level-visual areas that represent the semantic information in movies and scenes, the objects and actions in the movies. And you can see that this decoder also works quite well. It recovers talking, and woman, and man; covers all the semantic information in the movies.
So, at this point it’s still early days for noninvasive measurement of human brain activity. We’re much like the situation was in photography in the early 18th century. The pictures aren’t very good yet. They’re somewhat dim. But as long as we keep investing in neuroscience, we going to develop better methods for measuring the brain. And whenever we can measure the brain better, we can build a better brain decoder.
So, better brain decoders are in our future. These will be used to decode internal speech. They’ll be portable. They’ll be used for brain-aided CAD cam, dream decoding, all kinds of things. And these bring up interesting, I think, neural ethical issues having to do with privacy and use of information. You know, how are you going to protect your brain information, who has rights to use it, what will it be used for, and how do we control that?
Thank you very much for your time.
Further Reference
The Gallant Lab at UC Berkeley