So, a little bit about me. My name’s Allison Parrish. I am an experimental computer poet. Right now, I’m the Digital Creative Writer-in-Residence at Fordham University, where I teach computer programming classes to unsuspecting English undergraduates who just thought they were going to take a Creative Writing course. I’m also an adjunct at NYU’s Interactive Telecommunications Program. For the past couple of years there, I’ve been teaching a course called Reading and Writing Electronic Texts, which is sort of half introduction to Python the programming language, and half an introduction to procedural poetry, conceptual writing, and stuff like that.
Probably my most well-known project is everyword. This is a Twitter bot that tweeted every word in the English language in alphabetical order. It started almost eight years ago. It finished a year ago. We’re almost on the one-year anniversary of everyword’s completion. I started with the letter “A” and then went through “abacus” and all the way up to the final word, which is “étui.” I’m not going to give the presentation today about why it ended with “étui” instead of “zyzzyva” or “zyxt” or whatever. You can come see me talk another time if you want to hear that story.
In its heyday, this Twitter bot had a little over over 100,000 followers. For better or worse, it’s probably the biggest audience that I’ll ever have, and actually 100,000 followers is pretty good for a conceptual writing project, so I feel okay about that. I’ll talk about more about everyword later.
what is computer-generated poetry?
When I say that I’m an experimental computer poet, what I mean is that I write computer programs that write poems. Part of what I want to do in this talk is offer a new framework for thinking about what it means to write computer programs that write poems. Because usually when we think about computer generated poetry, we think of articles like this where any instance of some human task being automated is met by some story that’s like, “I welcome our robotic X overlords” where I replace X with whatever task is being automated by a computer. Most people when they think of computer poetry think that the task of the computer poet is to recreate with as much fidelity as possible poetry that is written by humans. I have no interest in making poetry that looks like it was written by humans. I think that that’s a plainly boring task that nobody should try to attempt.
The thing that I take inspiration from (which is weird wording to use when I’m talking about this quote in particular) is a quote from Jean Lescure, who is a member of the French Oulipo, which is a group of experimental writers based in France. He writes
The really inspired person is never inspired, but always inspired…
[This] sentence implied the revolutionary conception of the objectivity of literature, and from that time forward opened the latter to all possible modes of manipulation. In short, like mathematics, literature could be explored.
Jean Lescure, Brief History of the Oulipo
That little sentence, that little phrase there at the end really stuck with me, “literature could be explored.” This is an amazing, rad idea. I love my job. “Experimental Computer Poet” is a great job title to have. It’s an awesome thing to be able to put on your business cards. But if I had a career do-over, I would definitely want to be an explorer, like a space explorer. In particular, maybe an exo-climatologist; those guys are awesome. They study the atmosphere of planets in other solar systems, just by looking at spectrograms of the atmosphere data. That would be rad.
This metaphor of exploring literature really appeals to me, and I’ve made it my goal as a computer poet not to imitate existing poetry but to find new ways for poetry to exist. So what I’m going to do in this talk is take this metaphor of exploring literature to its logical conclusion. So the question is, if my goal as a poet is to explore literature, what does that mean? What space, exactly, am I exploring? How do I know if I’ve found something new in that space? What does that exploration look like? What are the tools? What’s the texture of that?
A lot of my computer poetry takes the form of Twitter bots, and I’ll be talking a lot about that later. So another question is, why are bots more suited to this task than any other form that that poetry could take?
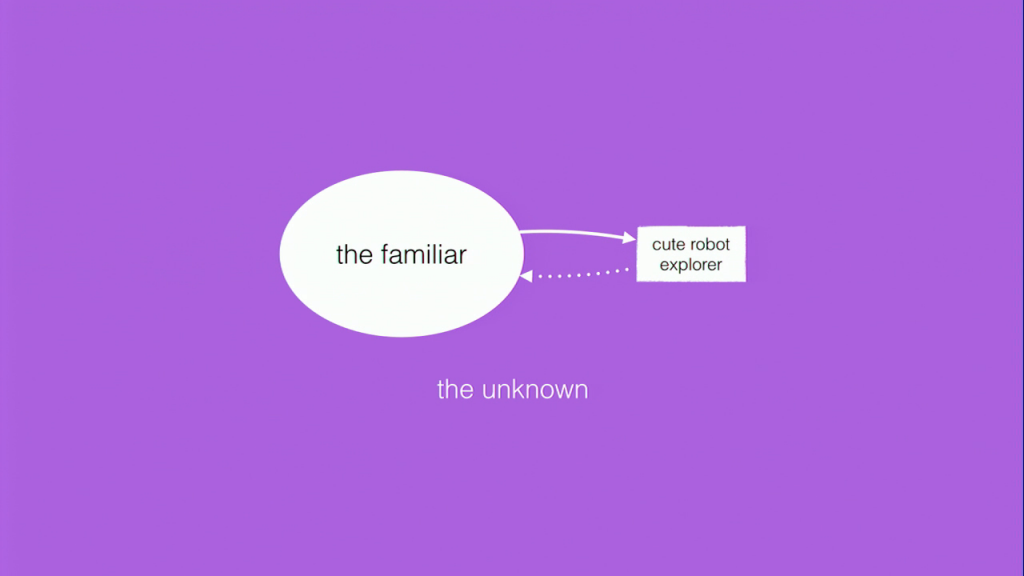
In pursuit of an answer to that question, this is my model of exploration. It’s a very simple way to think about exploration. We humans are there inside the bubble labeled “the familiar,” surrounded by this lovely purple miasma called “the unknown.” Surrounded on all sides by this inhospitable place where we can’t go because we can’t survive there; it’s a place that’s inhospitable to human survival. So in order to find out more about the unknown, we send out cute little robot explorers The little rectangle there goes out into the unknown and then collects telemetry for us. The dotted line there, coming back, is dotted because sometimes the robots don’t come back. Sometimes we send them out and the only thing that we get back from them is signals, like radio telemetry.
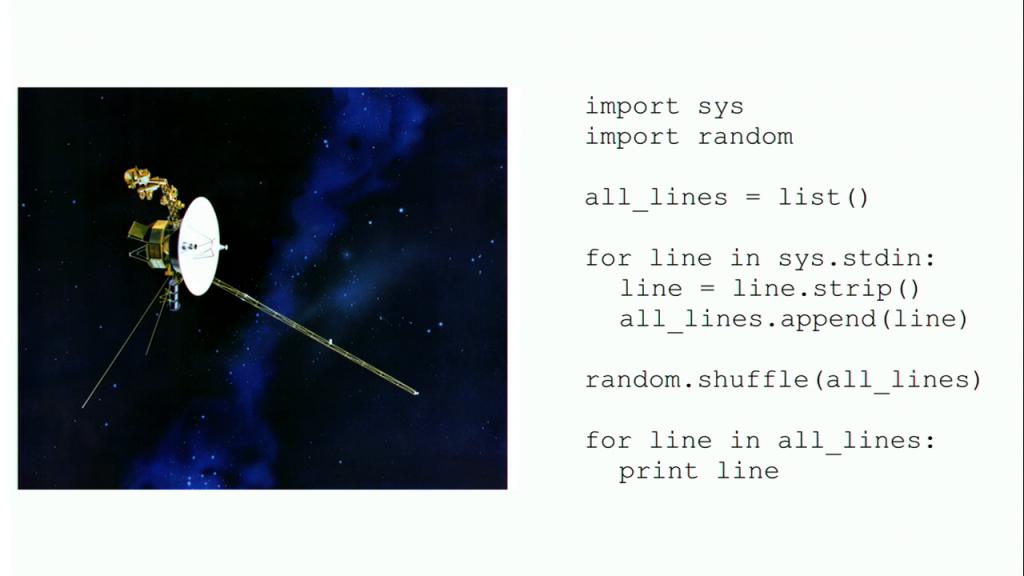
The idea of exploration for me implies traversal. You can only explore what’s unknown, and what’s unknown is by definition inhospitable. So we need special gear, we need special things to take us into the unknown realm. In extreme cases like space exploration, we have to send robots to do the dirty work. There on the left is Voyager 2, which is my favorite space probe. (Yes, I have a favorite space probe. I’m not a nerd, you are.) On the right-hand side is what I’m considering a very simple literal robot, and I’m using the word “literal” here in its most literal sense, to refer to words and letters. It’s a robot that deals with words and letters.
In this case it’s the source code for a tiny little Python program that reads in all of the lines from a given text, puts them into a data structure, and then spits them back out in random order. Very very simple program, but I think that this program is basically a way of exploring in the same way that Voyager 2 (in a very smaller scale, obviously) goes out into the universe and explores.
exploring (semantic) space with (literal) robots because humans abhor nonsense (and need help finding a path through it)
Here’s kind of what I see myself doing as a poet. I’m exploring space—except not outer space, semantic space—with robots, but not physical robots, literal robots. The unknown territory my robots explore is nonsense, basically. What is out there beyond the kinds of language that we know? My robots are exploring whatever parts of language that people usually find inhospitable.
So what do I mean exactly by “semantic space?” This term has a technical meaning that varies across disciplines, and because I’m a poet not a scientist, I’m going to take a very loose, ecumenical approach to defining it.
To have a space, we need some dimensions. Two dimensions would be nice, three would be better. And we need some way to quantify those dimensions, a measurable way of saying that Point A is a different point from Point B. In order to have a semantic space, we have to have some kind of system of relating a point in that space to language: sequences of words, concepts, etc.
I’m going to quickly review some well-known work in linguistics, psychology, and neuroscience that are related to semantic space as a concept. I don’t take any credit for this work, I just think it’s super interesting and it kind of informs the way that I approach poetry in my practice.
color
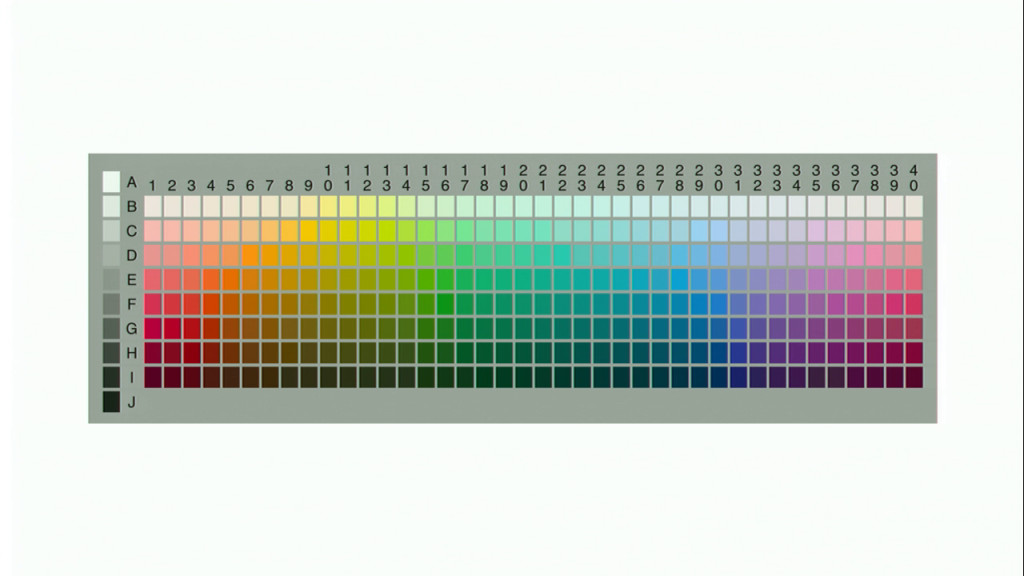
One kind of semantic space that immediately comes to mind is color. This is a chart based on the Munsell color system, which divides color into hue, value, and chroma. This particular chart was used in something called the World Color Survey, which was conducted by linguistics researchers Brent Berlin and Paul Kay. They asked speakers of many many different languages in the world to go through this chart and label every single cell with the word for that color in their language. They did this with many many different languages.
So here we have a very basic semantic space. We have a couple of dimensions, the dimensions of the color space, and we have a way to map words onto those coordinates by asking people, “What is the word that goes with this particular swatch of color?” Of course, this semantic space doesn’t cover all the possible concepts and words in a language. It’s just a smaller semantic space that you can use for a specific purpose, but that’s okay.
So if you’re a native English speaker and you’ve never really thought about this, you might think there’s only really one way to divide this spectrum up into colors. There’s red and there’s green and there’s blue and there’s pink and there’s purple. What’s the big deal?
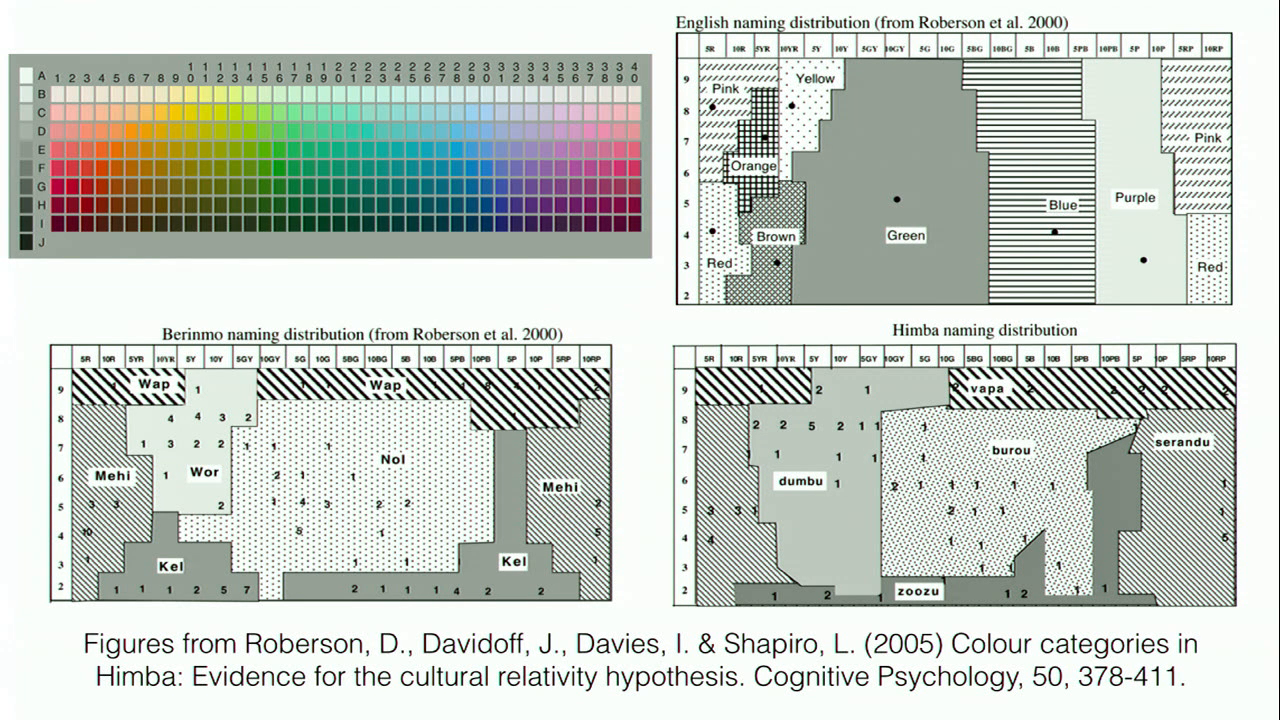
It turns out that across languages, the way that the color space is divided up into words is very very different. In the upper right hand here is the way that English divides up that spectrum. On the bottom are two languages that do it very differently. There’s Berinmo on the [lower left]. [On the lower right] is Himba. Berinmo is spoken in Papua New Guinea, and Himba is spoken in Namibia. So you can see for both of these languages, the spots on the chart are labeled with these contiguous blobs, and that’s the word in that language that corresponds to the colors in the chart. So in English we do it one way, and in these two other languages we do it in a very very different way. I think this is really fascinating, and the thing that occurred to me while I was putting together this talk is I wanted to make a Twitter bot that invented new ways of breaking up the color space, but I had to stop myself from doing that so I could finish writing the talk.
semantic priming
Another kind of semantic space, or at least a way of thinking about semantic distance, is shown through a phenomenon known as “semantic priming.”
[The video described here runs from ~10:35–11:34, or you can run the lexical decision task yourself.]
This is a video that I made of me doing what’s called a “lexical decision task.” This is an experiment that was devised in 1971 by David E. Meyer and Roger Schvaneveldt. The screen shows you a sequence of strings of characters, with a little “plus” symbol in between them, and you have to decide as quickly as possible whether or not the word is an English word or just a string of letters that aren’t an English word. The goal of the test isn’t to determine whether or not you can distinguish English words, it’s to test how quickly you recognize these words. For example, you see “game,” the next thing that comes up is “watch,” followed by “llama.” So the purpose of the task is to see how quickly you can determine whether or not a word is an English word.
The interesting result of this is that it turns out your reaction time for determining whether or not something is a word depends on what word you’ve just seen before. So if you see the word “bear,” you’re going to be very quick to determine that “tiger” is a word, because those two words are closely linked in your mind; the same thing with “lion.” But if I showed you “bear” and then I showed you “seedbed,” it might take a longer amount of time, unless you knew something about bears and seedbeds that I don’t know. Likewise with “bear” and “isomerism.” It would take you a long time to recognize “isomerism” if you’d just seen “bear.” Or “bear” and “frustum” are also two words, potentially. I don’t have experimental data on these words, I was just guessing. The experiment shows that words that are closely related have smaller reaction times. The name of this effect is called “semantic priming.”
Using this data, we can draw maps of semantic space that look like this:
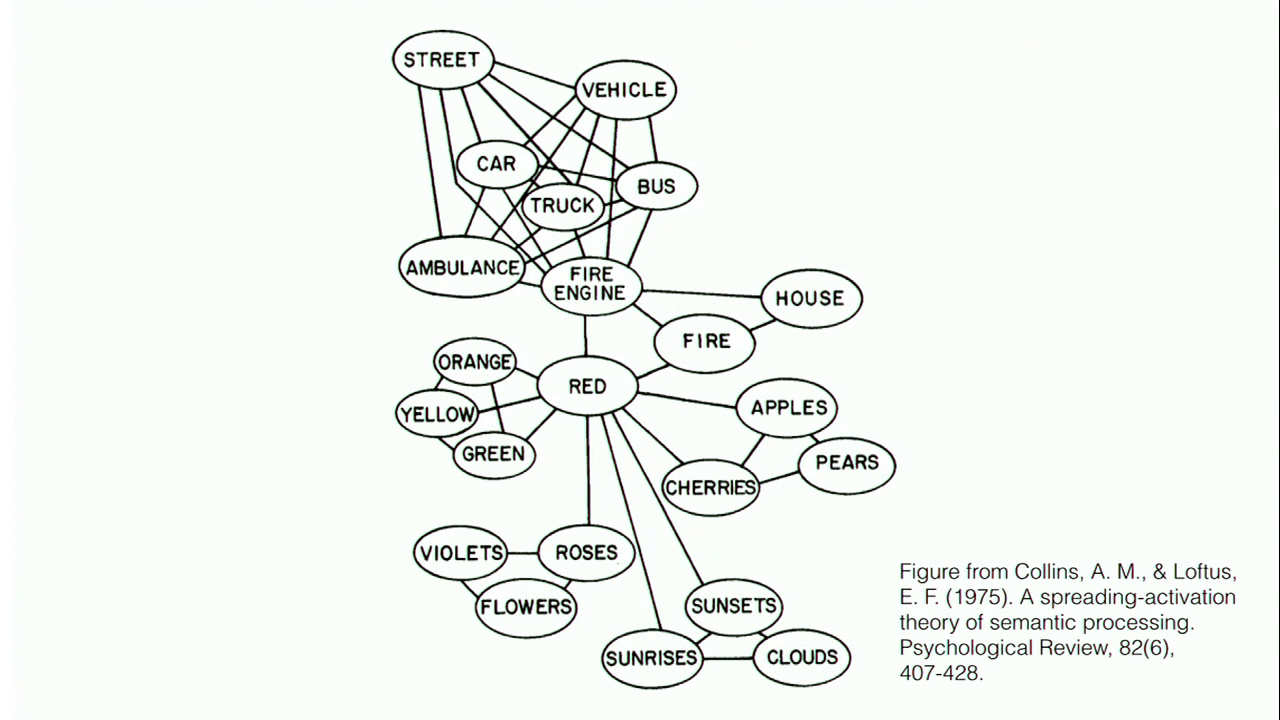
Figure from Collins, A.M., & Loftus, E. F. (1975). “A Spreading-Activation Theory of Semantic Processing,” Psychological Review, 82(6), 407–428
This isn’t a space, this is a graph with nodes and edges, but it’s definitely starting to look like something that we could explore. This is interesting data, and we could start making interesting poetry that used this particular kind of data.
MRI studies
Another thing that’s super exciting and interesting to me— I do not engage in any of these studies. I’m just an interested onlooker and artist who likes to repurpose other peoples’ scientific research for her own purposes. There’s a lot of interesting research into semantic space happening right now based on measuring brain activity with MRI scans.
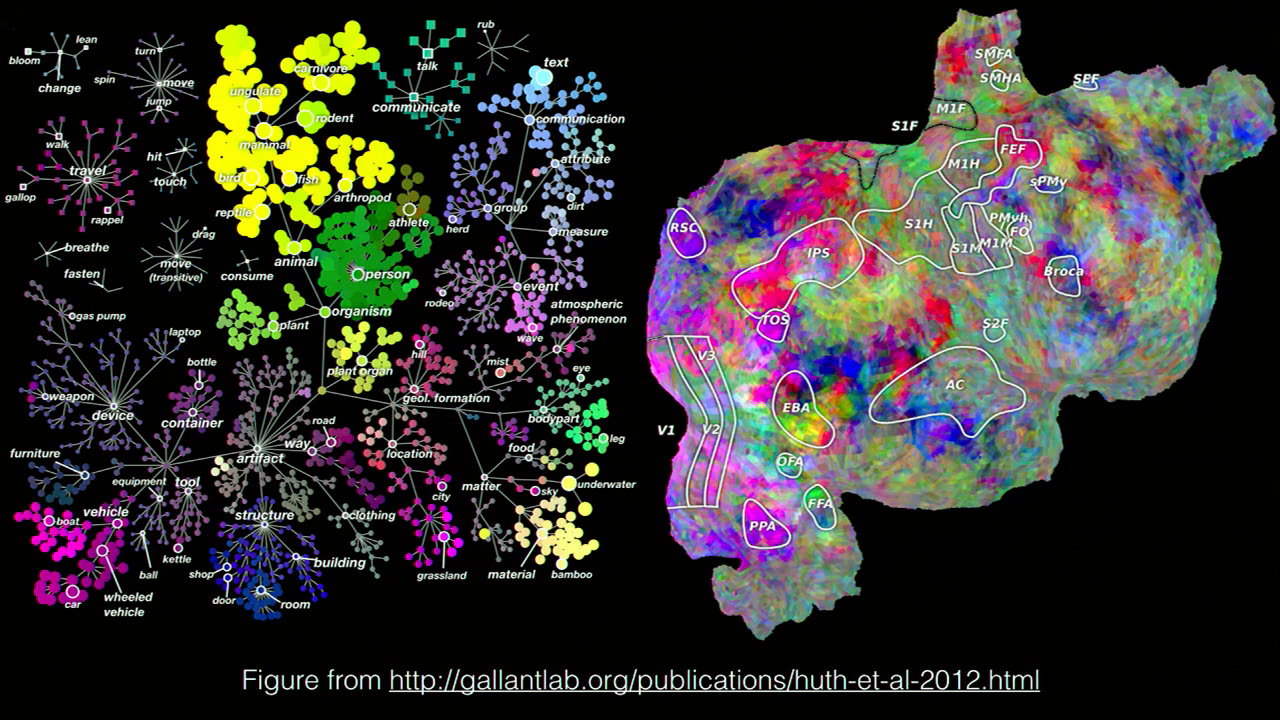
This is a totally amazing and beautiful visualization of a study that caught my attention while I was preparing this talk. Some researching at UC Berkeley have been doing fMRI imaging of people watching movies. The movies have been tagged with the objects that appear in each scene, and then they do an fMRI while the person is watching this movie, then record what object was on the screen at a particular time, and then record which parts of the brain are most active during that part of the movie. Then they associate those words with their position in the WordNet concept hierarchy in order to give a mental map of how concepts activate the brain in certain regions.
Quick aside on WordNet, in case you don’t know. WordNet is an amazing thing. It’s a freely-available database of concepts and categories. It has a hierarchy of “is a” relationships. In other words, if you type word “camembert” into WordNet, it will tell you that camembert is a kind of cheese, and it will tell you that cheese is a kind of food, and food is a kind of solid, solid is a kind of matter, matter is a kind of entity. I think that’s the top of the hierarchy. There might be something even higher than that. It’s really cool. I use it all the time. If I could do a second presentation at Eyeo, it’d probably be titled “WordNet: It’s Awesome and Poets Should Know About It.”
Back to the neuroscience, the panel on the left here shows the WordNet concept hierarchy, and the panel on the right shows the aggregate results of their MRIs. That’s a 2D projection onto the cortex of the brain, in case you don’t recognize that as a brain. The interesting thing is that the colors on the fMRI correspond to the colors in the WordNet hierarchy. So the yellow areas in the brain visualization are activated by the yellow areas in the WordNet hierarchy, which are animals. And the pink areas in the brain are activated by the pink areas in the WordNet hierarchy, which is moving vehicles and stuff like that. Super, super interesting. This is a really cool result, and it shows that there’s— we think of semantic space as being something that’s kind of abstract, but this shows that there might be a real physical basis for it inside of our brains.
n‑gram-based “lexical space”
I’ve been doing some experiments with visualizing something I’m called “n‑gram-based ‘lexical space.’ ” N‑gram is a fancy word for a sequence of words with a fixed length. So if the length of the sequence is 2, it’s sometimes called a bigram. If the length of the sequence is 3, it’s sometimes called a trigram. I’m working with n‑gram data from Google Books, which is freely-available; it’s a really cool data set. It’s essentially a big database that tells you how frequently certain n‑grams occur in Google’s book corpus, which goes back a couple hundred years.
So I have a big CSV file that has entries that look like “about,anything,124451”. This line says that the sequence of words “about” followed by “anything” occurs about 120,000 times in Google Books’ corpus.
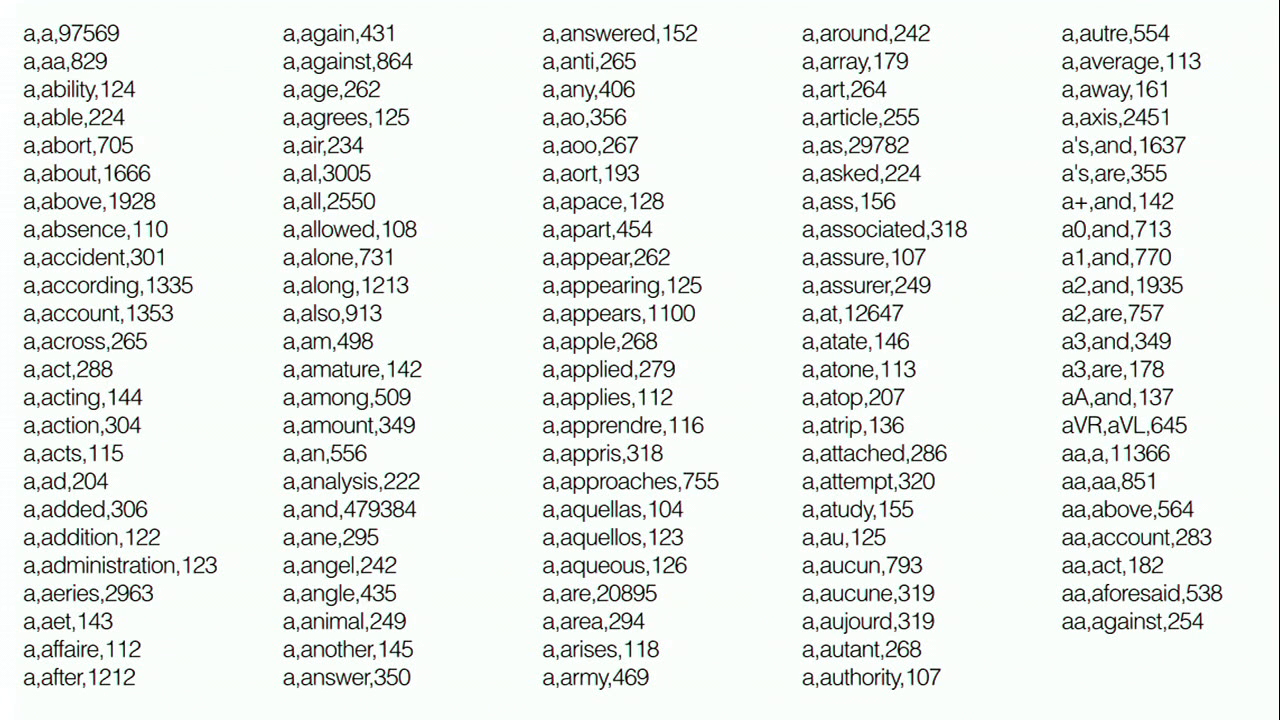
This is a whole bunch of data elements from that big CSV file. For these visualizations, I’m only working with n‑grams where both of the words begin with the letter “a” because that’s a very small, easy to work with subset. The whole data set is extremely huge, like gigabytes and gigabytes, and you have to set up a special server and stuff to work with it, and who really has time for that? So this is just n‑grams where all of the entries start with “a.”
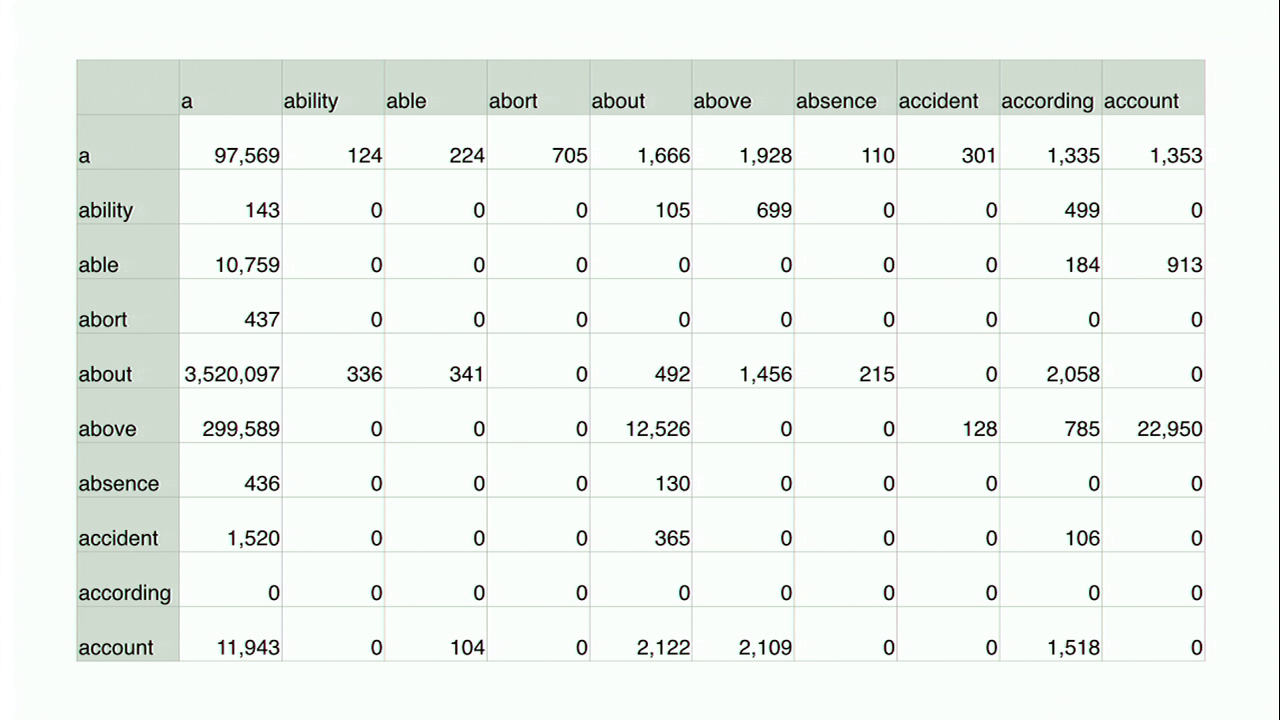
The way that I’ve been thinking about this is, if you took all that n‑gram data and put it into a matrix like this where the first word in the bigram is there on the left-hand side, the second word in the bigram is there on the top, and then the cell at their intersection shows you how many times that particular bigram occurs in the text. So for example, “about a” there on the left-hand side occurs like three and a half million times in the text, whereas “accident according” only occurs a hundred and six times.
So I took this data, put it into a big matrix, and I took all of the n‑grams beginning with the letter “a,” and I did a visualization that sort of represents these as a bigger rectangle based on how common that n‑grams is, and that visualization looks like this:
[Animation runs from 17:37–18:15.]
Which I think is pretty cool.
I made this in processing.py, which is an amazing version of Processing that you can program in Python. I highly recommend checking it out. This is all of the n‑grams visualized. The smaller rectangles are where there are fewer occurrences of that bigram; the larger rectangles are where there are more occurrences. You can see “and an” occurs a lot of times, “and also” occurs very frequently, “as an” occurs frequently. I did the same thing with trigrams, which added a third dimension to the visualization. This again is completely gratuitous, I just thought it looks really cool.
[Animation runs from 18:25–18:47.]
This is an example of what I’m calling “lexical space,” where we’re looking at n‑gram distribution and visualizing it in a 3D environment. This in particular really gets across this idea of exploring semantic space. To me this looks like a scene from some weird space movie or something, like a Minecraft space movie.
“exploring” semantic space?
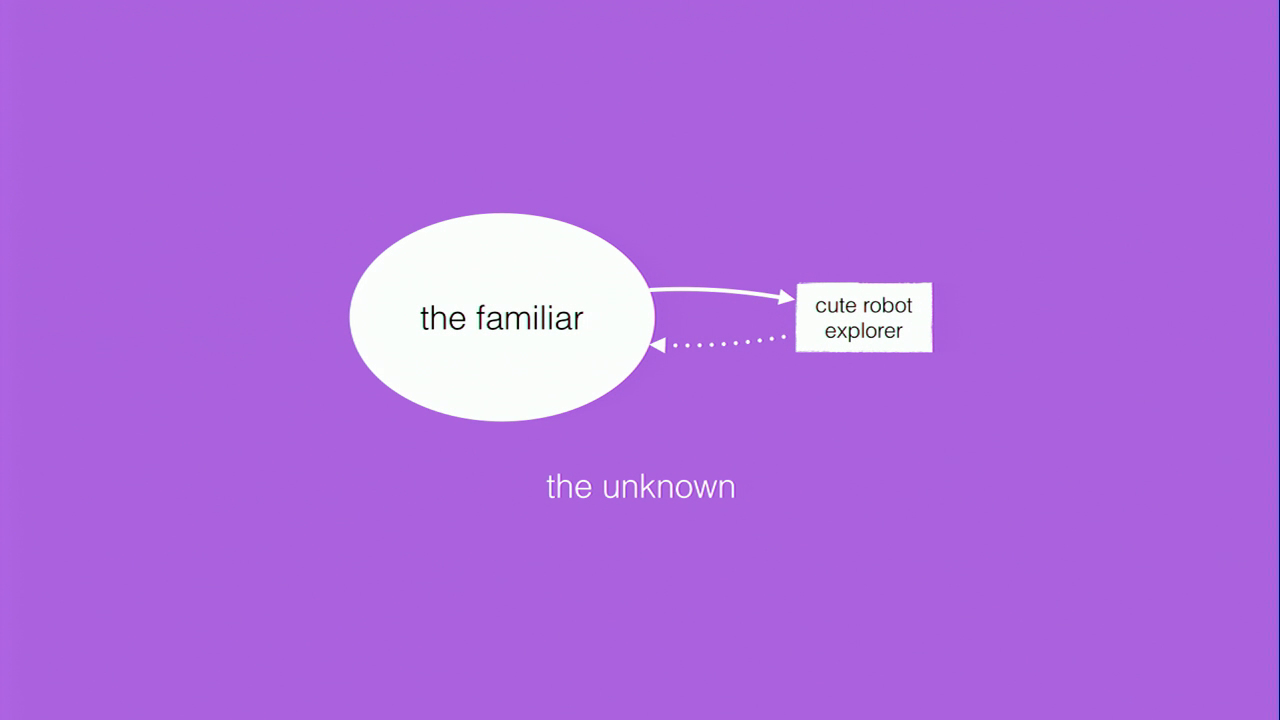
So now I’ve established that we can think about language concepts and words using a spatial metaphor. So what would it mean to explore semantic space? Here we are back with our little conceptual model of what exploration is. There’s stuff that’s familiar inside of the white bubble, and there’s stuff that’s unknown in the purple. When I’m thinking about exploring semantic space, what I’m thinking about is all of these large empty areas in this visualization of n‑gram space.
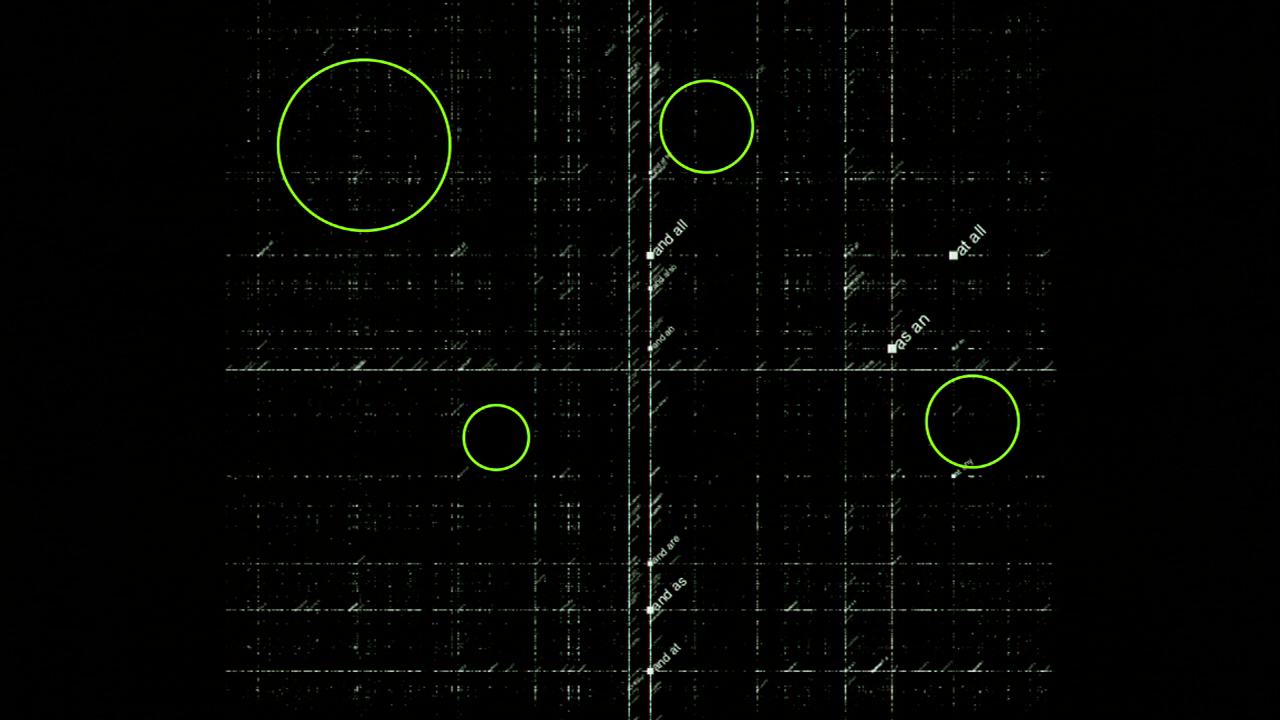
All of the whiter areas are all of the bigrams in English that we know and love. Some of the areas that I’ve circled in green here are areas where those bigrams just don’t occur. These are the unknown parts of language, sequences of words that’ve never been uttered in that sequence before. Here are some randomly-selected bigrams that have zero occurrences in the Google n‑grams corpus. These sequences of words may never have been seen before, except by me when I was preparing this talk.
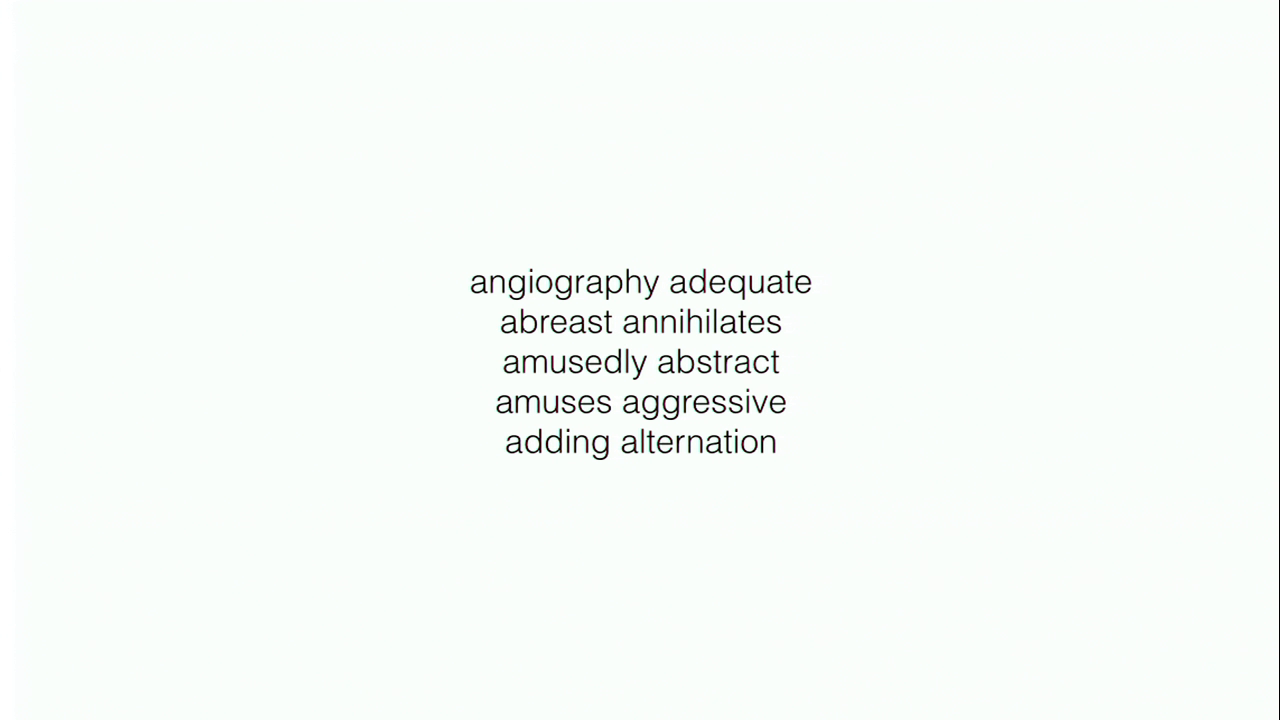
So that’s sort of what I mean when I’m talking about exploration. We’re finding these juxtapositions that’ve never been thought of or explored before just because of how we conventionally think about the distribution of language.
Another example would be to take this map of lexical activation that I was talking about a minute ago. One way to explore this map would be to attach a new node to this graph with random words that we select from a word list, and then find out how does this word connect to the other concepts inside of this graph. How long does it take to get from “ocean” to “bus,” or from “cobbling” to “pears?” This is another kind of exploration.
So basically what I mean is that the exploration of semantic space amounts to the generation of nonsense. By nonsense what I mean is words in unusual sequences, words that sometimes are in potentially uncomfortable, inhospitable sequences. Words that haven’t ever been spoken in that particular order before. The thing about nonsense is that people resist it, the same way that we resist climbing a mountain. We want things to make sense, we want things to be conventional, we want things to follow the rules. For most people, nonsense is frustrating and scary.
Poet and critic Stephen Burt wrote this book called Close Calls with Nonsense, and as a person with an interest in nonsense I was very excited to read this book. But as I started reading it, I realized that I hadn’t really understood the title. It’s Close Calls with Nonsense. This book isn’t about the sensation of nonsense or the vertiginous field of possibilities that nonsense represents, it was about coming close to nonsense but then realizing, with great relief, that what you thought was nonsense was actually sensical all along, you just had to learn how to look at it. And I realized the book that I really wanted to read would be Close Encounters with Nonsense, a book that’s about seeing nonsense close up and embracing it, and feeling what it’s like.
a brief history of unpiloted exploration/generative poetry
Speaking of close encounters, now I want to talk about space exploration. I want to do that cool thing that speakers sometimes do where they weave parallel histories from unrelated fields to tell an amazing story. I actually don’t think this is that amazing a story, but you guys can decide for yourselves.
This is my very vague history of two different kinds of close encounters. Unpiloted atmospheric and space exploration, and the history of procedural poetry. In both cases, explorers are designing devices that help them get back readings from environments that are usually considered inhospitable to human survival, whether that’s outer space or the frontiers of nonsense.
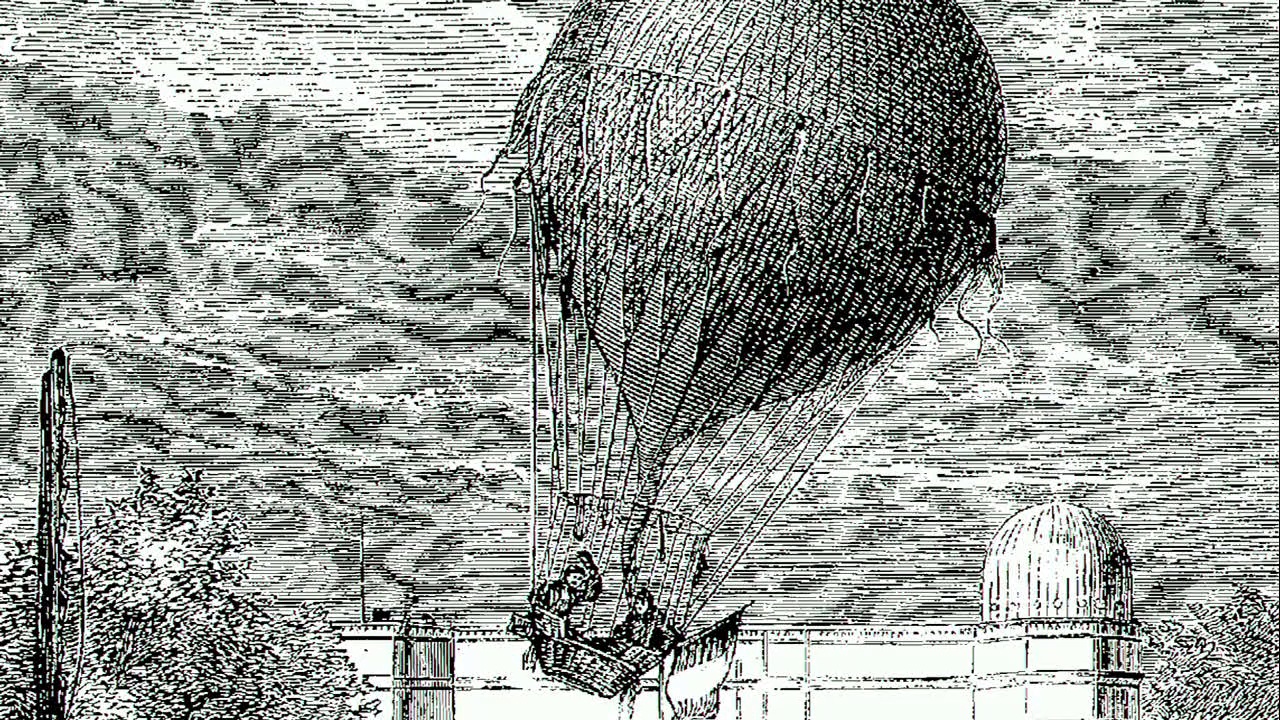
This is one of the first precedents for space travel. This is a delightful illustration of Jacques Alexandre Bixio and Jean Augustin Barral’s hot air balloon flight in 1850. They took a hot air balloon all the way up to 23,000 feet and they measured how temperature, radiation, and air composition changed in response to altitude. The flight was a success but of course it’s not a sustainable success. There’s only so far you can take humans into the atmosphere before you start running into things like the fact that you need oxygen to survive.
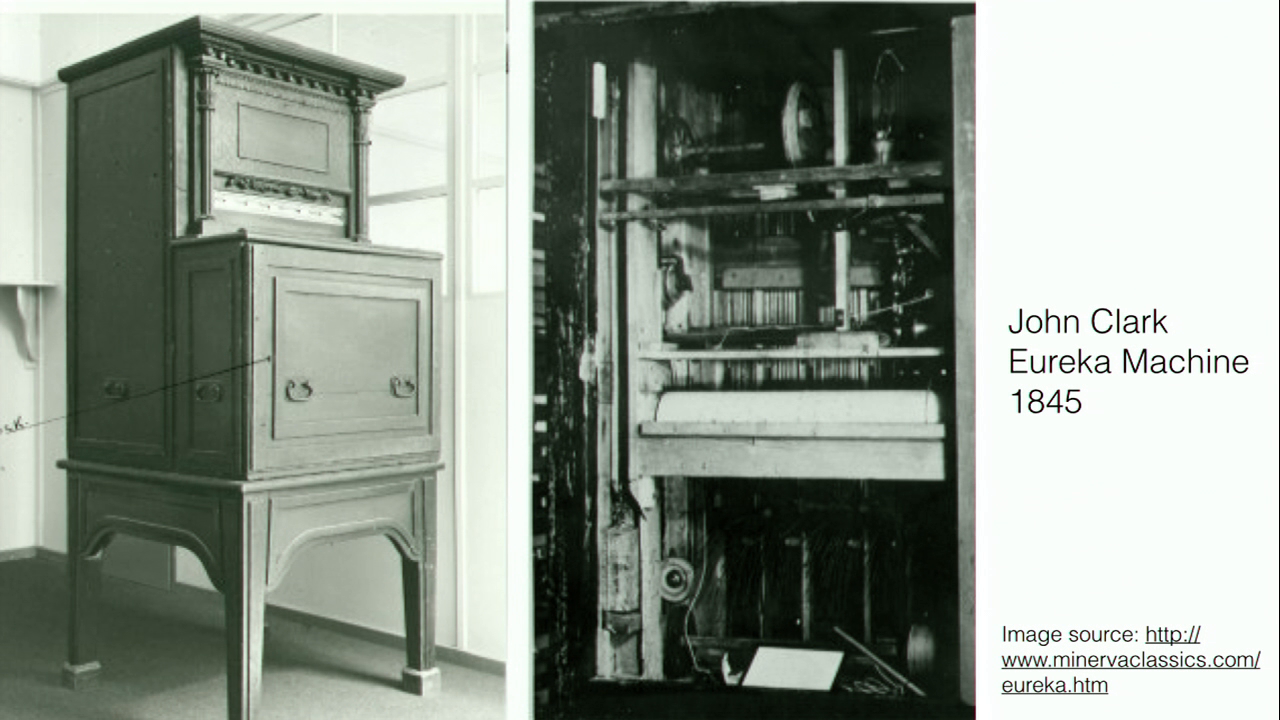
Image: Minerva Systems
In nearby England in 1845 just a few years earlier, John Clark invented the Eureka Machine, which is one of the earliest examples of a procedural poetry device. Specifically, it created Latin hexameter, kind of like this:
BARBARA FROENA DOMI PROMITTUNT FOEDERA MALA
Barbarian bridles at home promise evil covenants
Some people think that the history of procedurally-generated poetry only goes back like fifty years or so, but this is actually a pretty good poetry generator all the way back in 1851. It wasn’t generated with a computer. This device wasn’t a general computer; it was specifically a mechanical device, devised for this task, but it is procedural. It’s rule-based poetry.
ANON (1845), “The Eureka,” Illustrated London News (19 July 1845)
A brief account of the Machine for Composing Hexameter Latin Verses. It states that the machine produces about one line of verse a minute — “during the composition of each line, a cylinder in the interior of the machine performs the National Anthem.”
According to a contemporary account, the machine also, there in the last line: “a cylinder in the interior of the machine perform[ed] the National Anthem” while it’s generating Latin hexameter, which I think is a great touch for any poetry generator. Just put some music on top of it and it’ll be better instantly.
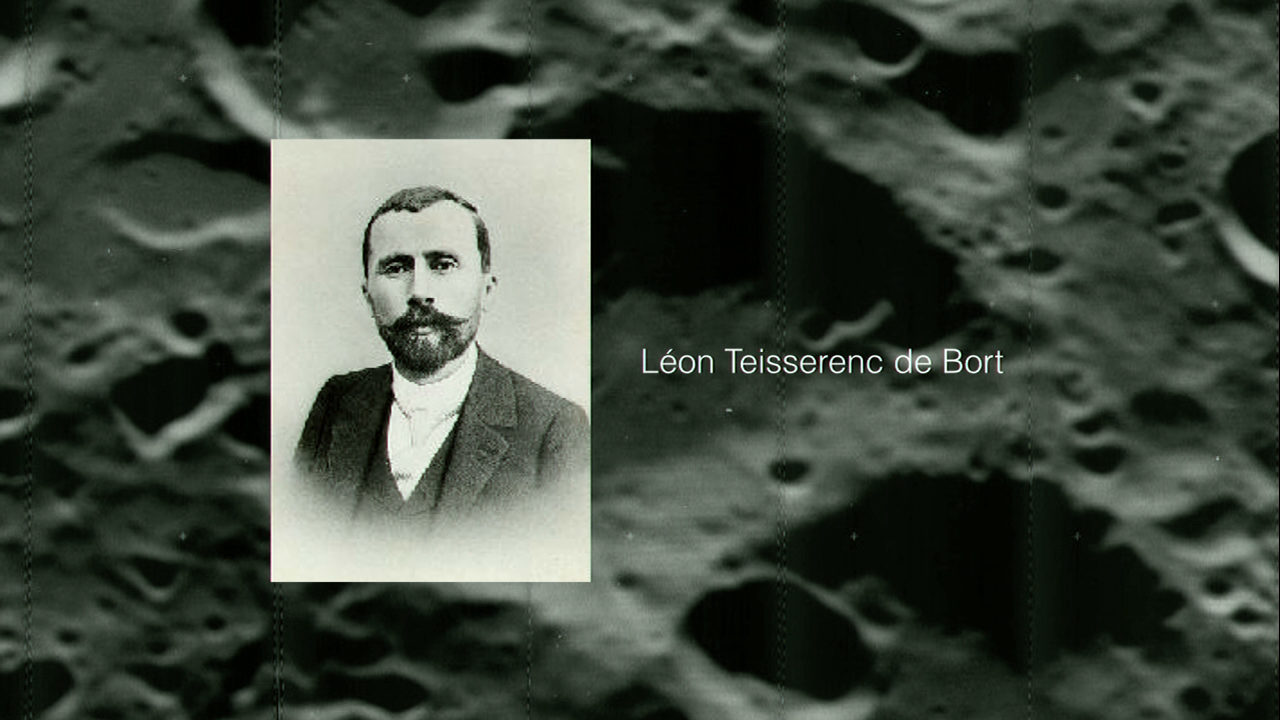
Meanwhile, back in the atmosphere, Léon Teisserenc de Bort had the grand idea of just attaching weather instrumentation to the balloon, without the people on it, which allowed the balloon to go much higher and collect readings from much further into the heights of the atmosphere. The background is the moon crater named after him. I was told to make my slides look pretty and to take advantage of the wide format. He didn’t go to the moon, I just wanted something pretty to go in the background.
So he made these instruments that flew up in to the atmosphere and then after a certain amount of time the instrument would fall down. He attached a little parachute to it, and then he’d go around and collect the instruments. So these weren’t automated, but they were unpiloted, which allowed him access to facts about the universe from places inhospitable to humans.
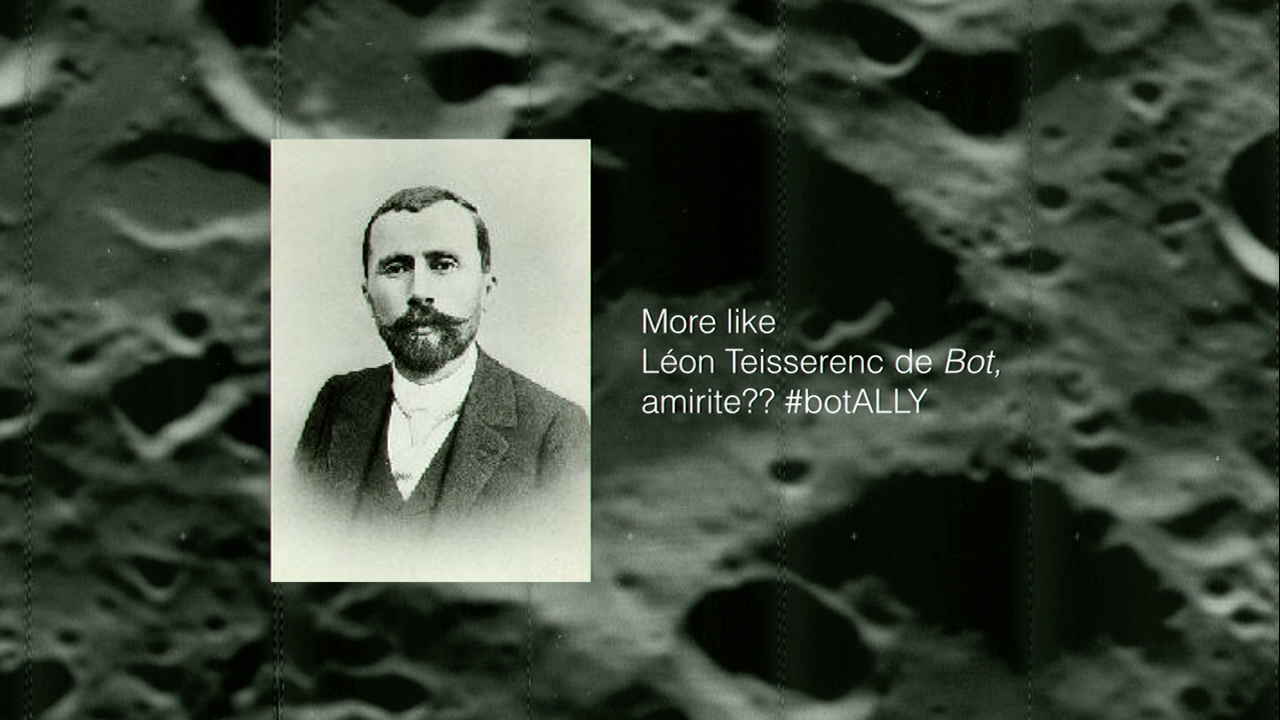
These were our first unpiloted explorations into the unknown. This may make him, in the eyes of some, the first bot-maker. This slide is for Darius [Kazemi].
Starting in the 1920s, meteorologists had the additional brilliant idea of having the weather probes send back a radio signal with their telemetry from higher up in the atmosphere. This was called a radiosonde. It’s a device that takes soundings of remote environments and sends the data back automatically.
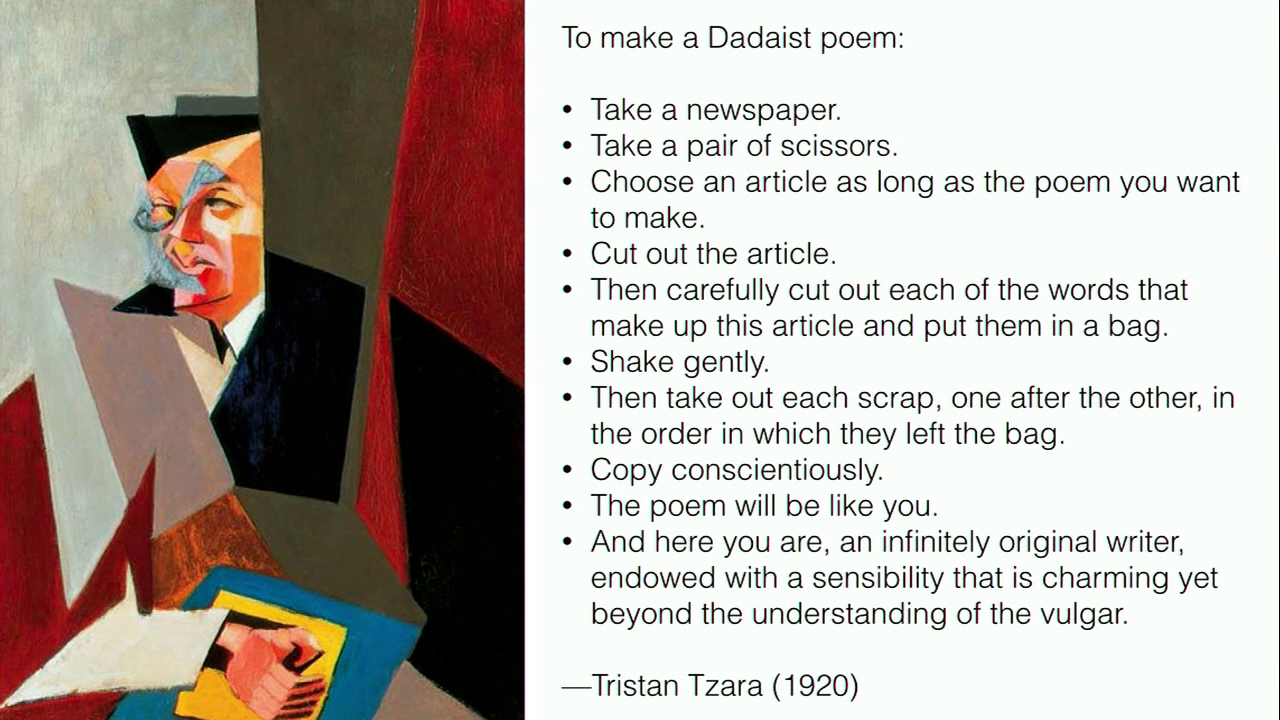
One of the first and most important language soundings was by the Dadaist Tristan Tzara, who wrote this instruction for how to make a poem. Basically cut up a newspaper, randomize the words, copy it back to a sheet of paper, and then you are a poet. This is basically a program for writing poems. And I’m calling this a sounding. It’s a way to venture into nonsense, find out what’s there, and give us results back from that, making a foray into these unknown semantic realms with minimal human intervention. This isn’t automated, of course. You actually have to do this process. But it does seem like a computer program. We’re most of the way to computer-generated poetry here.
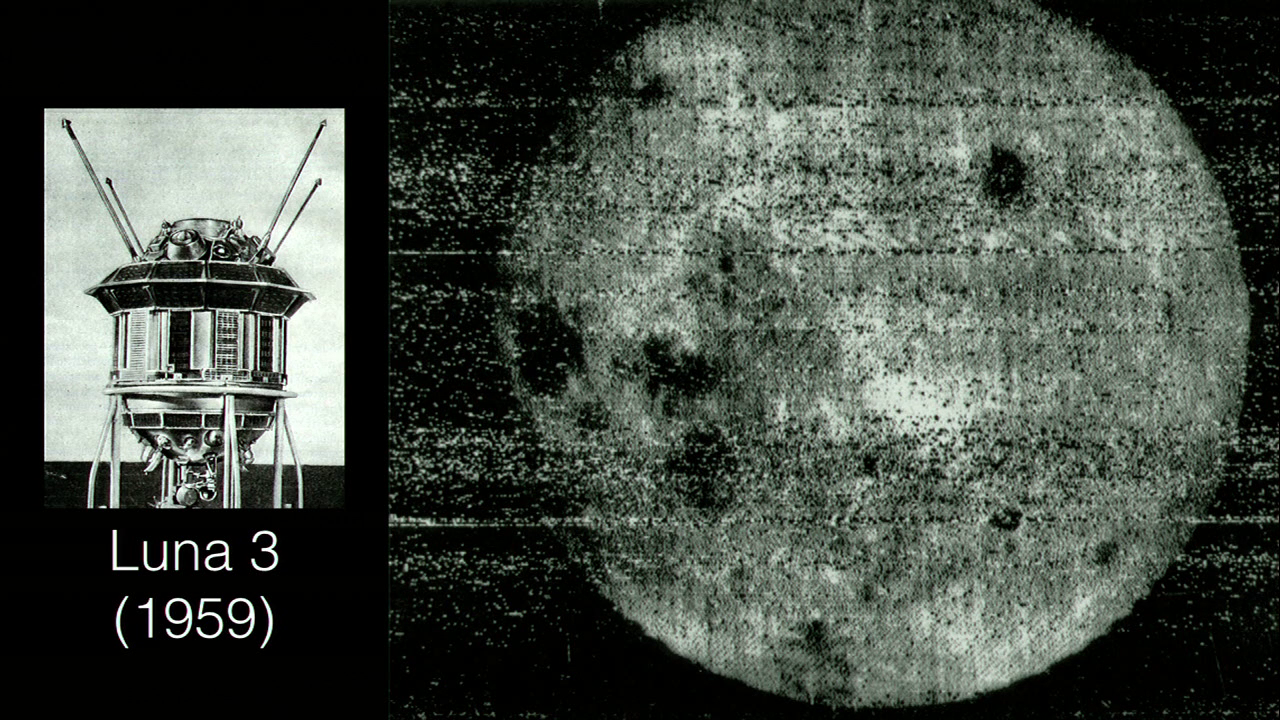
Fast forward a few more decades, and the idea of radio sounding in the atmosphere has progressed to radio sounding in space. This is Luna 3. Quickly after Sputnik, the USSR launched a series of probes at the moon, and in 1959 Luna 3 sent back the first photograph of the dark side of the moon. Now we’re really entering the age of exploration, where robots are sending us visions of things that were not just previously impossible to visit, but previously impossible to even see, which is pretty awesome.
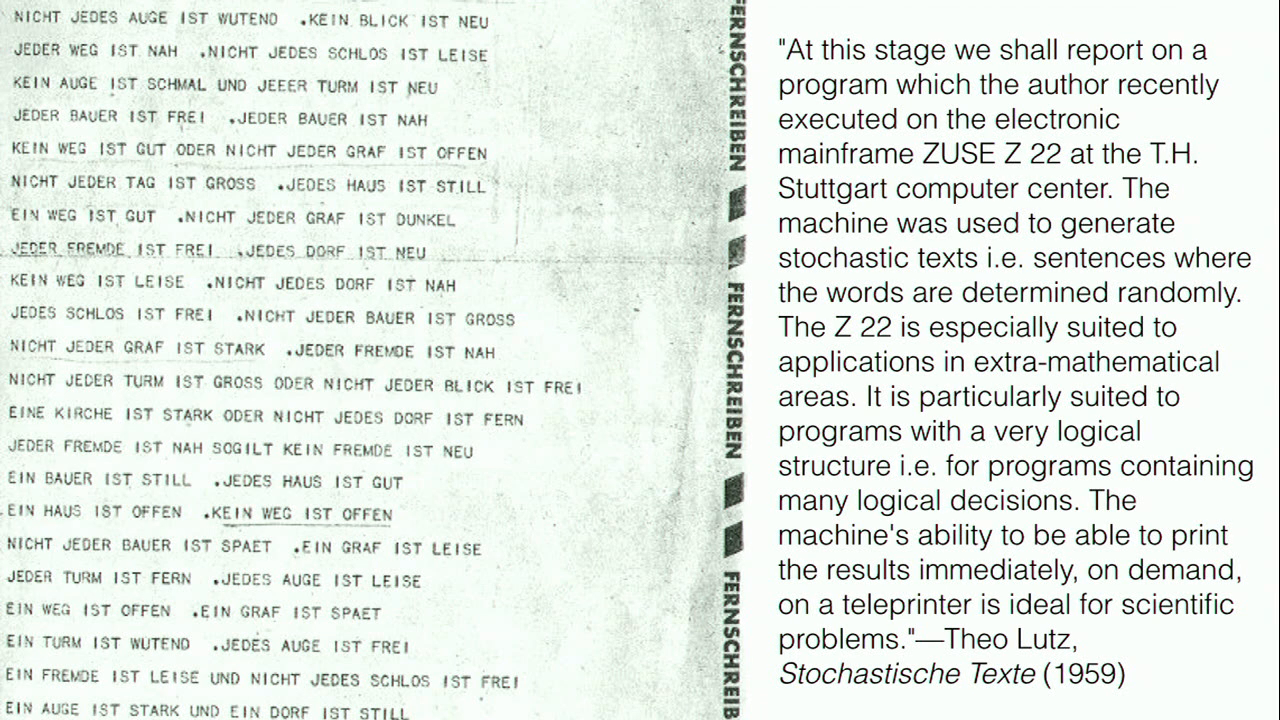
Not uncoincidentally, in 1959, Theo Lutz created the first computerized poetry generator, or what’s widely-recognized as that. His description of the project sort of reads like an advertisement for this particular brand of mainframe. “The Z 22 is especially suited to applications in extra-mathematical areas.” Which basically means, “You can do text with this computer, and here’s how I know.” But it’s computer-generated poetry nonetheless. It’s the first truly automated semantic space exploration agent that can head into the unknown territories of semantic space and send back telemetry of what it finds there, in this case in the form of a printout. The first literal robot exploring semantic space.
So whether balloons and space probes are taking soundings of the universe, or whether it’s procedural poetry taking soundings of semantic space, they’re both doing the same kind of work in my view, just accomplished by different means.
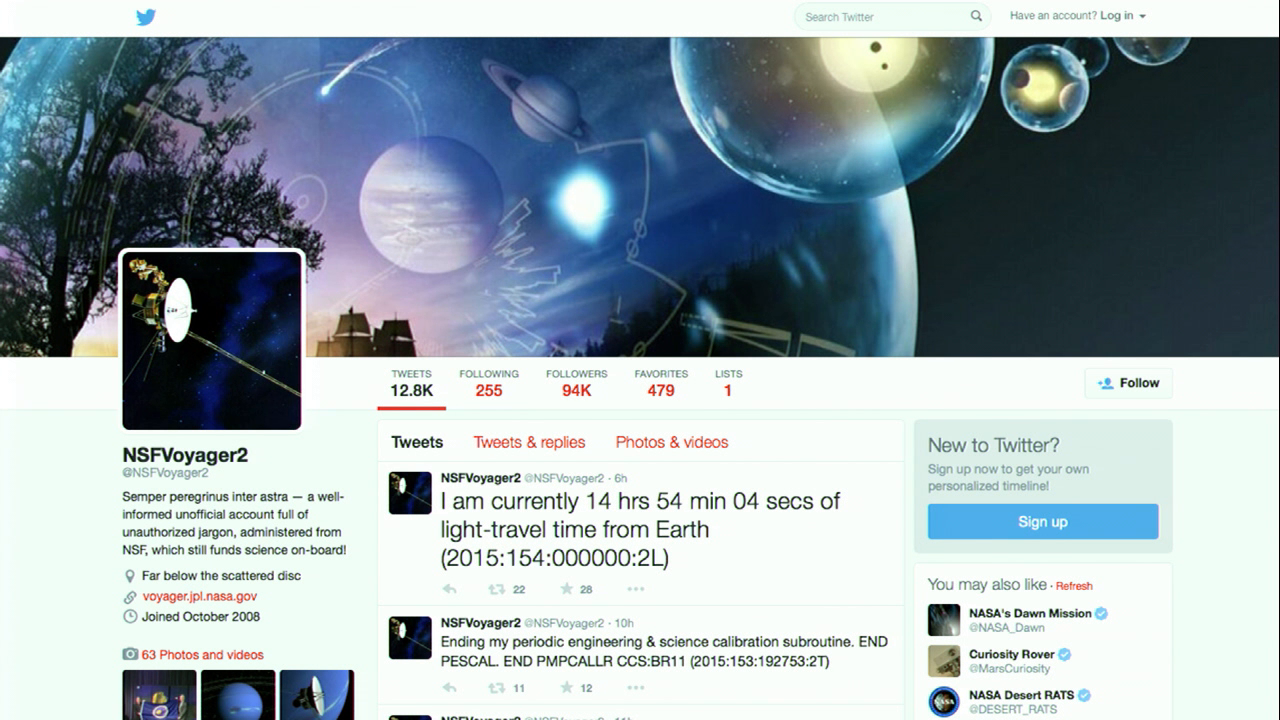
Of course nowadays the work of a space probe can look a lot like the work of a procedural poet. This is the Twitter account of Voyager 2, which is still in operation, which is amazing. I doubt that anything I make will still be working thirty of forty years after it’s deployed. But Voyager 2 is still going strong. The account tweets telemetry from Voyager 2 and it comes right into the Twitter feeds of ninety-four thousand people. Most of the time it’s just saying, “Hey, I’m calibrating something” but that’s kind of exciting, to get information about calibrations from many many light days away.
some of my (literal) robots
Now I want to talk about a few of my own Twitter bots. I like to think of my Twitter as being sort of—well, a lot—like Voyager 2. They’re Twitter accounts that report on the telemetry being sent back from robots that are doing exploration in weird places.
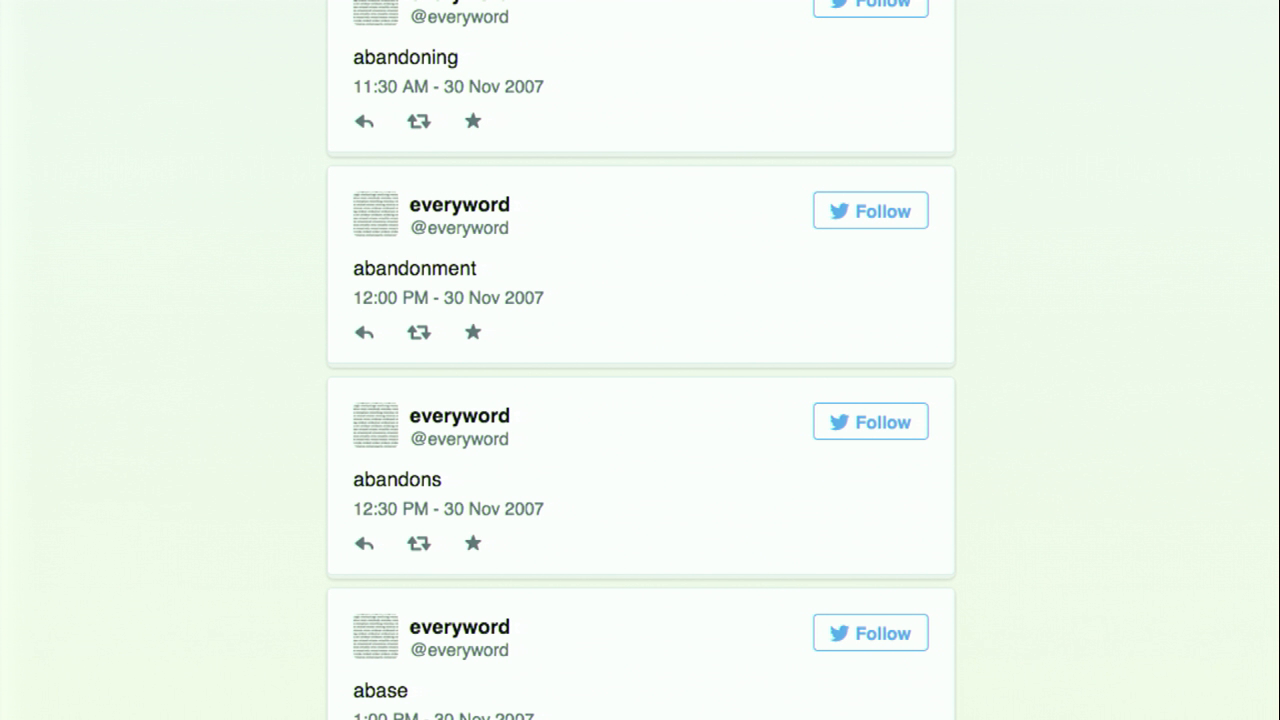
@everyword, as I mentioned earlier, is a bot that I made in 2007 with the intention of tweeting every word in the English language. This is composed of a Python program which connects to the Twitter API. Every half hour it read the next line from the file, and sent it to Twitter. Not very complicated at all. This is what the account looks like if you were just to look at it in the order that the tweets were tweeted. Of course, if you were following it, you would get these one every half hour into your Twitter feed, and they would exist in juxtaposition with the other stuff in your feed. If you visit the account’s page on Twitter, the tweets would be in reverse-chronological order, of course. But the general idea remains the same.
Going back to that visualization of bigram frequency, if you use the @everyword corpus to do this same visualization, you’d end up that looks like this:
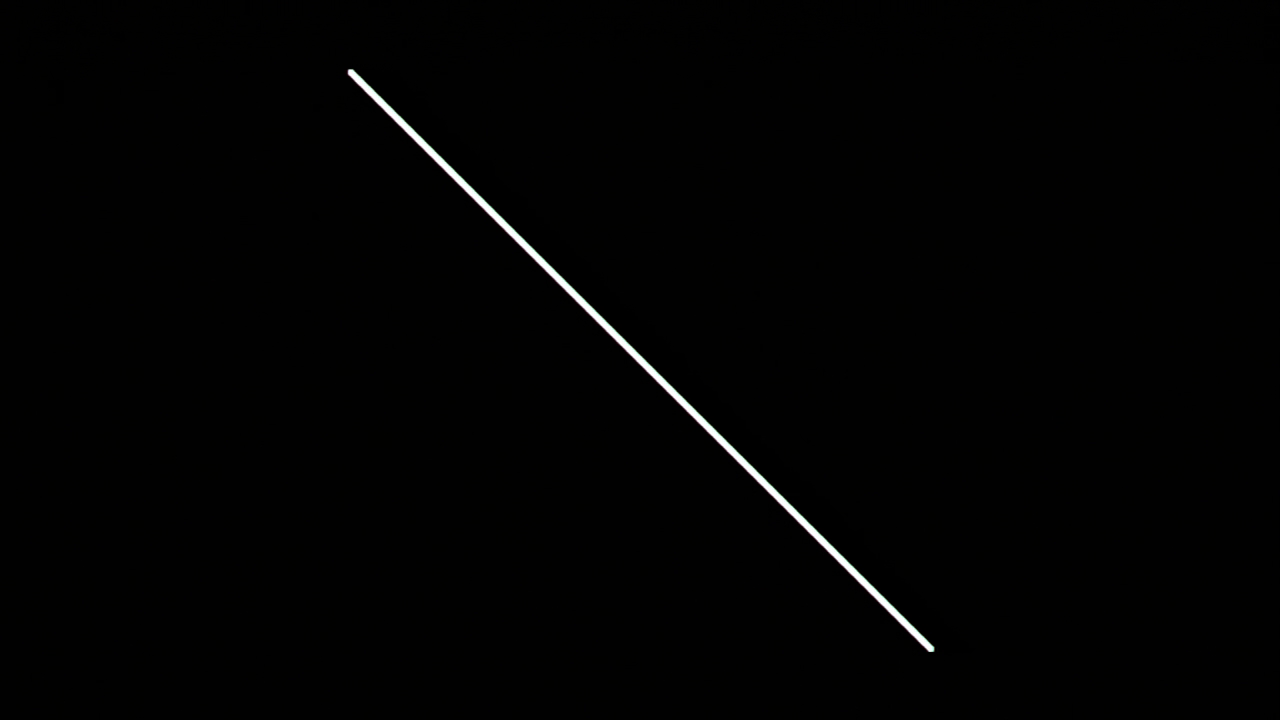
It’s just a straight line through the bigram space, because each word only occurs once, and only occurs in juxtaposition with the word that preceded it alphabetically. This graph kind of reminds me of graphs of the exploratory routes of space probes as they head off into the solar system.
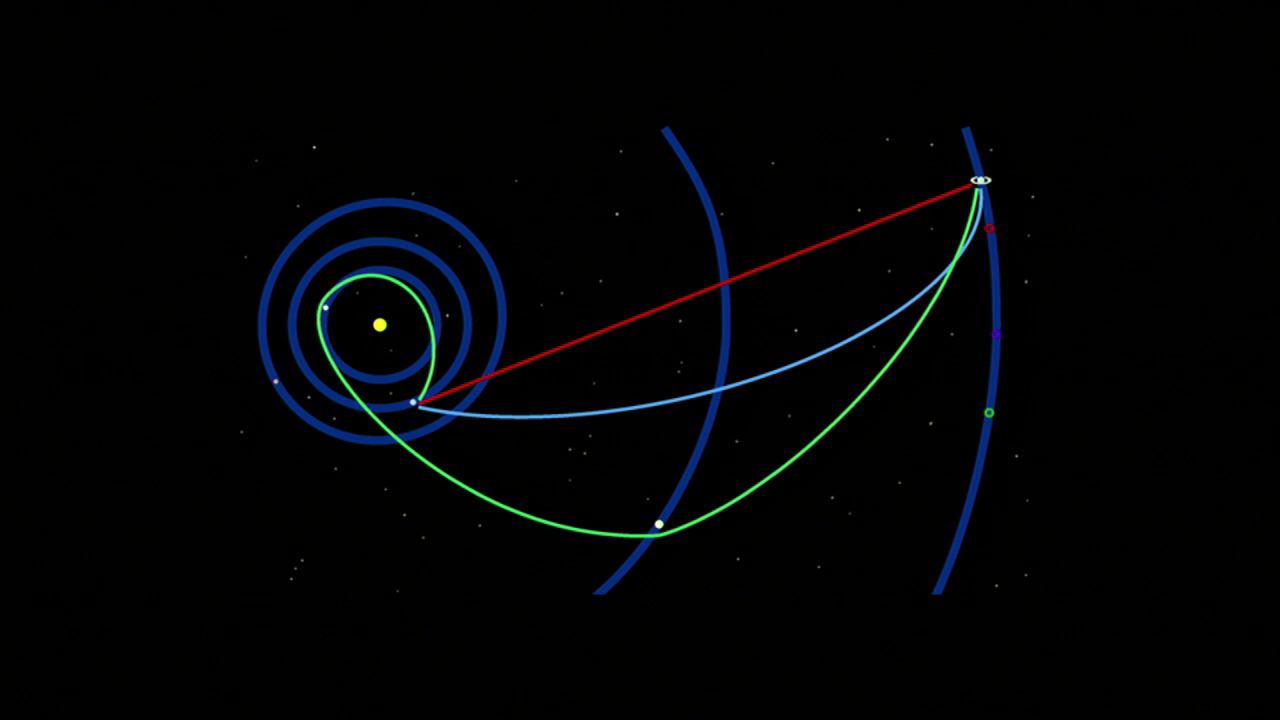
Image: Space probe trajectory example, Wikimedia Commons
Everyword I think is one of the simplest possible exploratory soundings of language. It’s getting a measurement, just going straight through the semantic space and reporting back on what it sees. Everyword I think is also a pretty good example of why a Twitter bot is an idea platform for experimental writing in the same way that it’s difficult to survive in outer space, it can be really difficult to engage with nonsense. You’d probably never buy a book with every word in the English language in alphabetical order. Well, you would; it’s called a dictionary, but you wouldn’t buy it with the intention of reading it from beginning to end, right? But reading it one word at a time every half hour, that’s something that’s a little bit easier to do, and tens of thousands of people decided to engage with this particular work of writing on Twitter by following this bot.
Also, Instar Books is my publisher. We are actually publishing @everyword the book, so check out [their] Twitter account for more details on when that will be released later this summer.
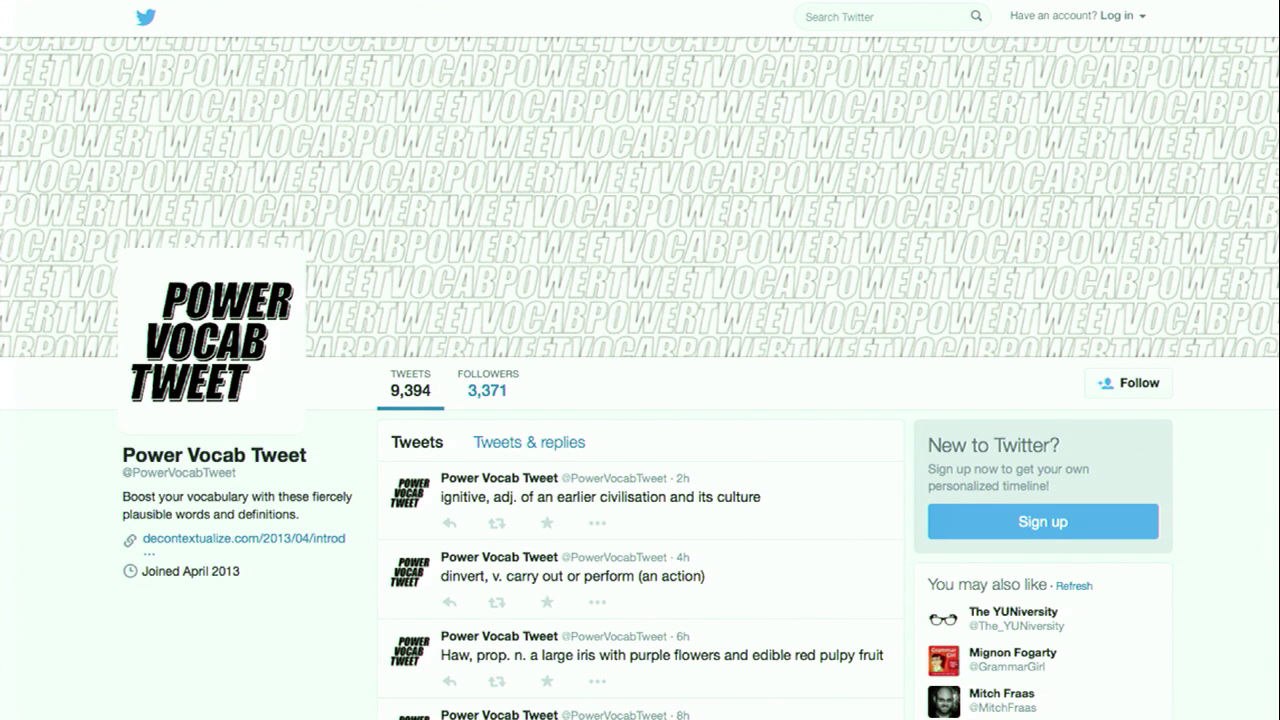
Another Twitter bot of mine is called Power Vocab Tweet. This bot explores semantic space in a very straightforward way by making up new words with new definitions. The words are generated by randomly splicing together two existing words based on character count and syllables. The definitions are generated using a Markov chain text generation algorithm based on word definitions in WordNet. (So thanks again WordNet for giving me the tools that I need to make cool stuff.) The bot ends up generating words that fill in gaps in semantic space that you would never have otherwise thought of. Here are a few of my favorites.
https://mobile.twitter.com/PowerVocabTweet/status/468345328878747648
https://mobile.twitter.com/PowerVocabTweet/status/452188868084002817
This is a delicious new spring fashion:
https://mobile.twitter.com/PowerVocabTweet/status/593852323845255168
Power Vocab Tweet has over three thousand followers, which is pretty good. I’m not sure why. I think it might’ve been included, earnestly, on some “improve your vocabulary by following these Twitter accounts” lists in spite of the bot’s bio, which says “Boost your vocabulary with these fiercely plausible words and definitions.” I feel like that bio gives away the game, but maybe not for some readers.
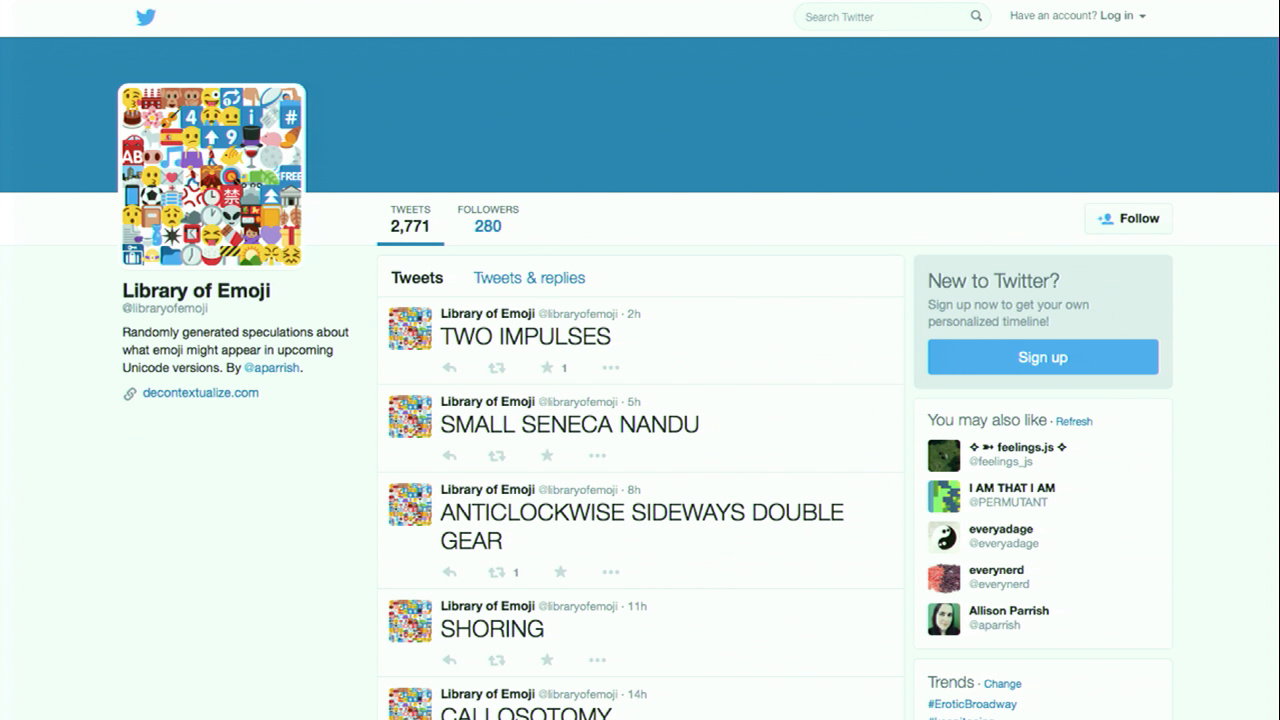
I also made a bot called Library of Emoji. As you may know if you are a Unicode fanatic like I am, the Unicode Consortium releases a new list of emoji every so often that are going to appear in upcoming Unicode versions. So Library of Emoji is a bot I made that sort of speculates on what those might be, using a random process that uses a context-free grammar generator. Like Power Vocab Tweet, it sometimes names concepts that are ideal for emoji and you would never have guessed before that you needed an emoji that had this particular meaning, but once you see it you know that it’s something that you would use all the time.
This has a very specific use:
https://mobile.twitter.com/libraryofemoji/status/490487983712526336
If you’re on the X‑Files, you probably need this emoji.
You might need an emoji for the hindsight fairy:
https://mobile.twitter.com/libraryofemoji/status/526545574297075712
There are Unicode characters for shapes. You might need a Unicode character for a parallelogram with potential:
https://mobile.twitter.com/libraryofemoji/status/540180415878017024
And then the one emoji that we actually need to put in all of our online communications is “poisonous technocracy,” which all of us deal with every day:
https://mobile.twitter.com/libraryofemoji/status/505934765372215296
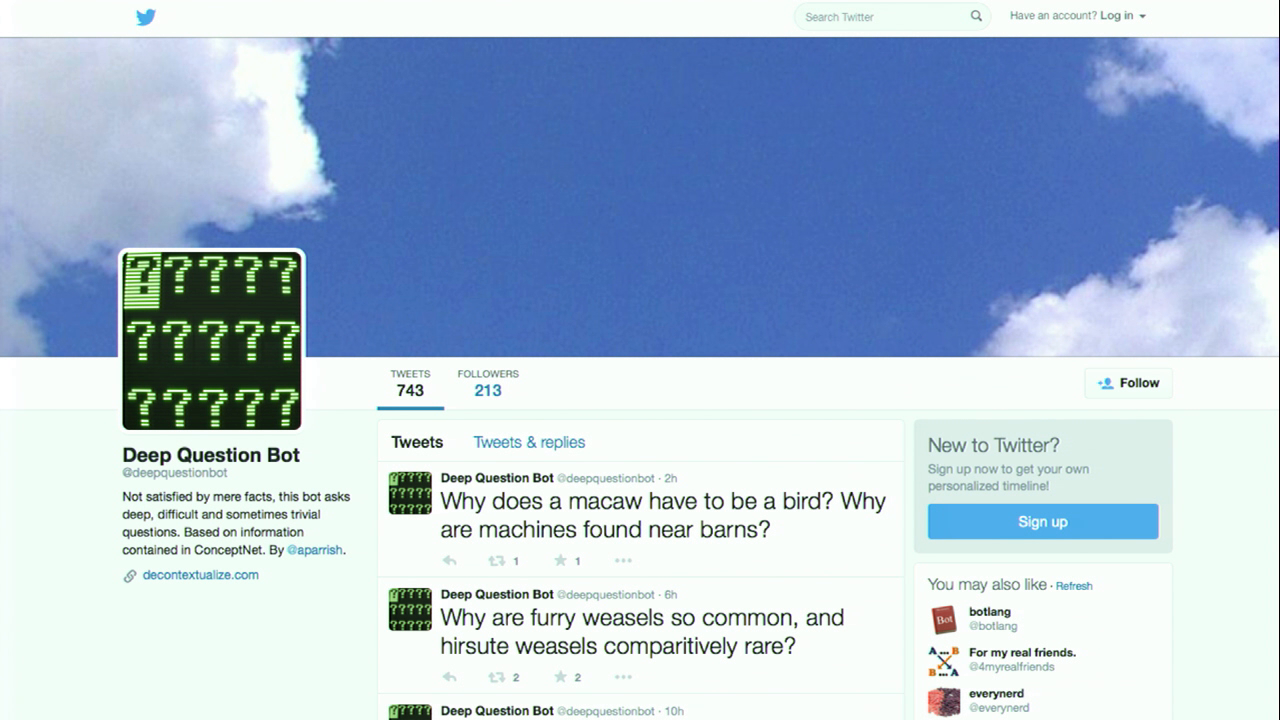
The last little semantic space probe I want to talk about today is Deep Question Bot. This is a fairly recent project. It composes deep questions based on information in ConceptNet. ConceptNet, by the way, to take another cheesy aside, is sort of like WordNet instead of just having “is a” relationships, it tells you all kinds of common sense facts about things. So instead of being able to just tell you that cheese is a food product, ConceptNet will tell you what parts cheese has, or where cheese is commonly located, or the purpose of cheese, or whatever. It’s very weird and a lot of it is volunteer contributed so it’s not super accurate (not that common sense is anything that you could ever actually be accurate about) but it’s very handy and cool for creative writing experiments.
So Deep Question Bot explores semantic space by taking facts from ConceptNet and then just inserting them into templates that call that that common sense into question.
https://mobile.twitter.com/deepquestionbot/status/565700072357519362
So ConceptNet says that mailboxes have mails, but why must mailboxes have mails? Give me a good reason.
Sometimes it invents unlikely situations.
https://mobile.twitter.com/deepquestionbot/status/563706936940843008
What if you found an egg in a dishwasher instead of a refrigerator? I don’t know, what if?
Deep Question Bot almost reached self-awareness this one:
https://mobile.twitter.com/deepquestionbot/status/563284151064100866
And then it had a bit of capitalist critique:
https://mobile.twitter.com/deepquestionbot/status/601561372489555968
Well, have you considered that?
I make new Twitter bots all the time. But they are all sort of concerned with this idea of just taking text and finding news ways of putting it together so we can plumb a little bit deeper into the realms of nonsense.
ethics of semantic exploration
Before I close, I want to talk briefly about another point. I’ve been talking a lot about exploration, and I’ve been working under the assumption in this talk that exploration is something that’s inherently virtuous. But of course, in the history of humanity, exploration is usually just a euphemism for theft and violence. Exploration usually means, “Oh, I’m coming to where you are and I’m going to take your stuff.” Much of the technology that I’ve discussed like radiosondes and space probes were originally developed for military purposes, or with military aims in mind. I think the closest analog in technology right now to the development of the weather balloon is the drone, but I hate drones. Drones are used for surveillance and remote-controlled violence, and I don’t want my poetry robots to do violence. I want them to delight and to elicit wonder and sound the depths of human perception and experience, and I’m wondering is it possible to use exploration as a metaphor for the kind of work that I do and still accomplish those goals given the loaded nature of that metaphor.
As I said before, exploration implies a frontier. And when there’s a frontier, there are people inside of the frontier and there are people beyond it. The word “barbarian” means outsider. But it comes from the ancient Greek. It’s actually onomatopoeia that signifies speech that doesn’t mean anything, “barbar,” or babbling. And it’s telling that we use this word (someone that we don’t understand, someone who speaks nonsense) to refer to people that we consider not to be our own.
I said earlier that nonsense is something that’s never been said before, but obviously something only counts as having been said if we recognize that someone’s vocalizations, that their language, counts as speech, and not everyone is extended that privilege after all. Lots of speech from marginalized groups is dismissed as “nonsense.”
So the border between what’s known and what’s familiar, and what’s sense and what’s nonsense, and what’s discovered and what’s undiscovered, is very much dependent on who gets to speak, who we acknowledge. So for this reason I think that lifting up the voices of the unheard is just as important exploration as sending up wordy weather balloons and semantic space probes.
This is a great quote from Ursula K. LeGuin in her essay “A Non-Euclidean View of California as a Cold Place to Be:”
One of our finest methods of organized forgetting is called discovery. Julius Caesar exemplifies the technique with characteristic elegance in his Gallic Wars. “It was not certain that Britain existed,” he says, “until I went there.”
Ursula K. LeGuin, “A Non-Euclidean View of California as a Cold Place to Be”
The point here for me is that just because there appears to be empty space on one of our semantic maps doesn’t mean that nobody has ever spoken in that space before, or that we necessarily have a right to go there. All exploration is subjective, it happens from a point of view. This is true even for exploration conducted by robots, whether they’re physical robots or semantic robots.
An issue that I think about a lot is using other people’s text. When you’re doing semantic explorations with computer programs, often you’re working with an existing corpus. There’s this great quote from Kathy Acker, who’s talking about the way that language is inadequate for her to talk about herself and her experience of life,
I was unspeakable, so I ran into the language of others.
Kathy Acker, “Seeing Gender”
I like to think that she’s saying not just “I ran into the arms of the language of others” but also “I ran into it and collided with it” thereby scattering it all over the place.
But using the words of others, appropriating the words of others, can be a very powerful methodology, especially when you’re borrowing from people that are in positions of power, and using their words against people in power that are there unjustly. But just because other people’s are available to you doesn’t mean that those words belong to your or that you have a right to use them. Just because you can appropriate someone else’s text doesn’t mean that you should. Kenneth Goldsmith is not here, obviously, but I’m looking at him when I’m saying this.
One of the main dimensions of ethical semantic exploration is this: Be respectful of other people’s rights, their own words, and the words that concern them. Also, if you’ve found a gap in semantic space, the gap might be there for a reason. The concept or n‑gram or turn of phrase that you’ve identified in your exploration might name something that’s harmful or violent. So it’s important to take precautions against this and take responsibility when your bot does something awful. Programmers, like all poets and all engineers, really, are ultimately are responsible for the output of their algorithms. This is a quote from a great essay by Leonard Richardson called “Bots Should Punch Up:”
[W]hat’s “ethical” and what’s “allowed” can be very different things… You can’t say absolutely anything and expect, “That wasn’t me, it was the dummy!” to get you out of trouble.
Leonard Richardson, “Bots Should Punch Up”
He uses a metaphor of a bot being kind of like a ventriloquist’s dummy. You can’t get away with saying anything that you want and then just say, “Oh well, it was the dummy that did it.” It’s not the dummy, it’s you. You did that. That was a state of affairs that you caused to exist in the world.
poetry
To conclude, the mission of poetry I think is to expose readers to the infinite variability and expressiveness of language. The problem is that lots of these possibilities of language are locked up behind barriers that we find it difficult to get through. We can’t see these parts of semantic space that have all kinds of interesting things that will activate our brains in weird ways. We need somebody to hold out hands as we walk into those unknown areas.
So I write computer programs that write poetry not to replace poets with robotic overlords, but to do the exploratory work that humans unaided find difficult to do. Because a computer program isn’t constrained by convention it can sort of speak interesting truths that people find it difficult to say, and it can come up with serendipitous juxtapositions that make language dance and sing and do unexpected things that can be beautiful and insightful. So I’m very excited to be doing this work and excited to be teaching others how to do it.
Thank you.
Further Reference
On August 5, 2015 Allison announced her bot The Ephemerides, imagining what “poems written by space probes would look like.”
Citations
- Phraselette: A Poet’s Procedural Palette
- Computational Poetry is Lost Poetry
- I, rowboat: The Intricacies of AI & Poetry
- Evaluating Mixed-Initiative Creative Interfaces via Expressive Range Coverage Analysis
- Titling,‘Titled, “Untitled”’
- The Artist in the Machine: The World of AI-Powered Creativity
- Tweeting the cosmos: On the bot poetry of The Ephemerides
- Poetic.computer