Karrie Karahalios: So in 1963, demonstrators black and white marched on Washington. I was trying to explain Martin Luther King Day to my seven-year-old the other day, and it was really really hard. And I was secretly— I was publicly glad he could not imagine a space where different people had different water fountains, or had to go to different schools because of the color of their skin.
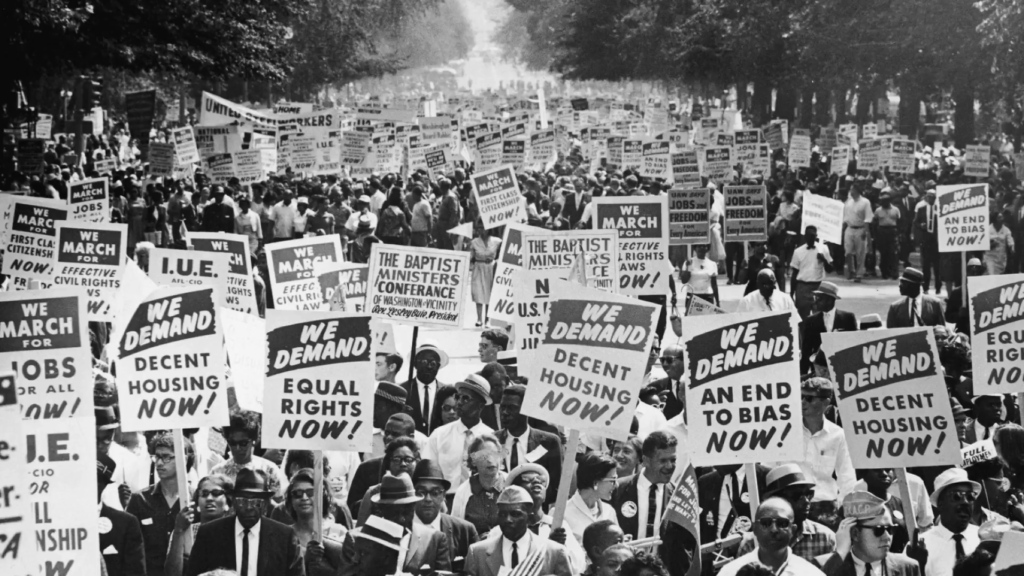
In reading about this historical event it was also interesting to read from historians that JFK don’t even want to do this this early. He was hoping this would be a second presidential term type thing, not a first-time thing. But watching this very public violence unfold in the newspapers, on television, made him want to end this unrest much much sooner. And so he actually went along and supported this protest, to minimize any possible violence that might occur if he did not support it.
By the way, people were actually marching for many many many things. Over 200 thousand people showed up. They were marching to stop segregation, for equality in housing, and for quality in society—jobs in general, as you can see from some of these photos.
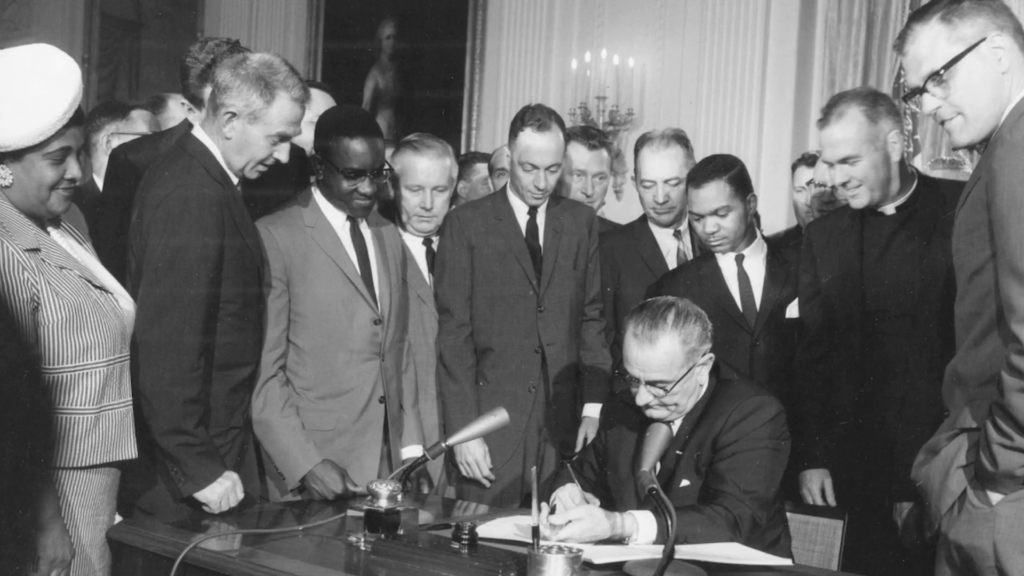
Less than a year later, Martin Luther King was dead, JFK was dead, Lyndon Johnson signed this into law and he was very much behind this. This was the Civil Rights Act of 1964, and there were many other Civil Rights Acts before and after. It prohibited discrimination based on race, color, religion, sex, and national origin by federal and state governments as well as for some public spaces. Also, what was interesting is that this bill actually went beyond what was proposed in the House and the Senate and it actually provided the cutoff of funds for people who violated some of these laws.
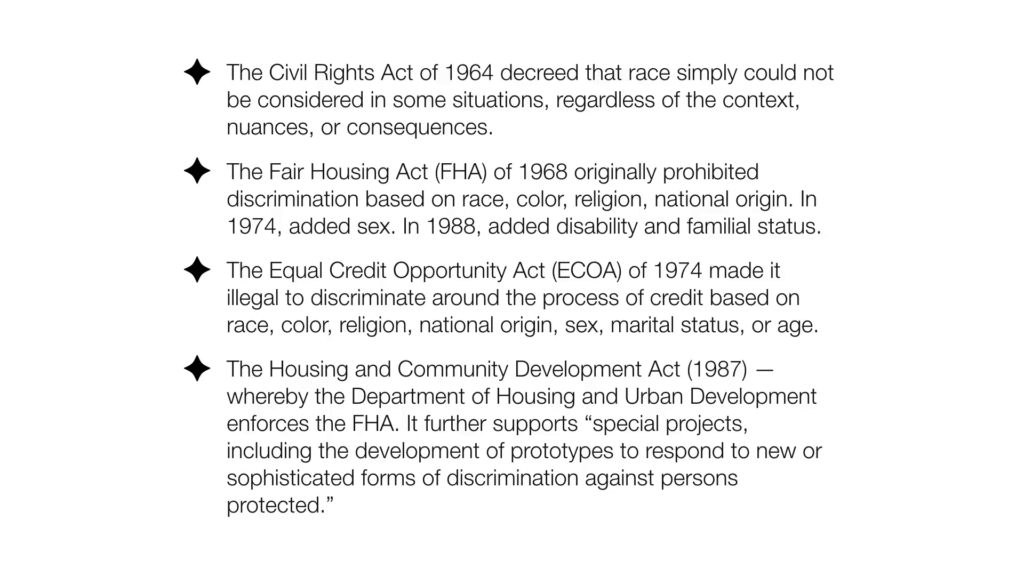
And as I said, with the Civil Rights Act of 1964, after that came the Fair Housing Act of 1968, which originally prohibited discrimination based on race, color, religion, national origin. In ’74 they added sex. In 1988 they added disability and familial status.
And one thing I just want to state, that it’s been harder historically for black women in the world of housing. It turns out that more single black women owned houses than single black men, and yet credit has been harder. The Equal Credit Opportunity Act of 1974 made it illegal to discriminate around the process of credit based on race, color, religion, national origin, sex, marital status, or age.
And then in 1987 we had the Housing and Community Development Act. And that brought on the Department of Housing and Urban Development to enforce the FHA. It further supports (and this is critical to our work) special projects including the development of prototypes to respond to new or sophisticated forms of discrimination against persons protected.
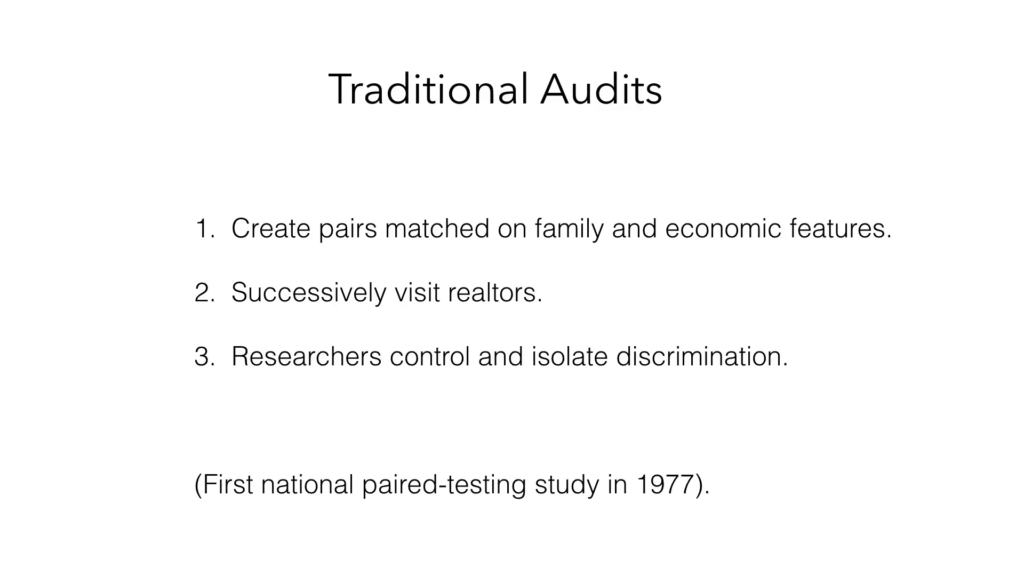
So what did they do, in terms of trying to protect these new forms, to find this discrimination? They started with a type of audit. And what they did is they created pairs of entities looking for housing and they matched them based on economic status, familial status…and they had them visit a realtor successively. And what they found was where one person was discriminated versus when they were not. The first paired study was done in 1977. The most recent one was done in 2012.
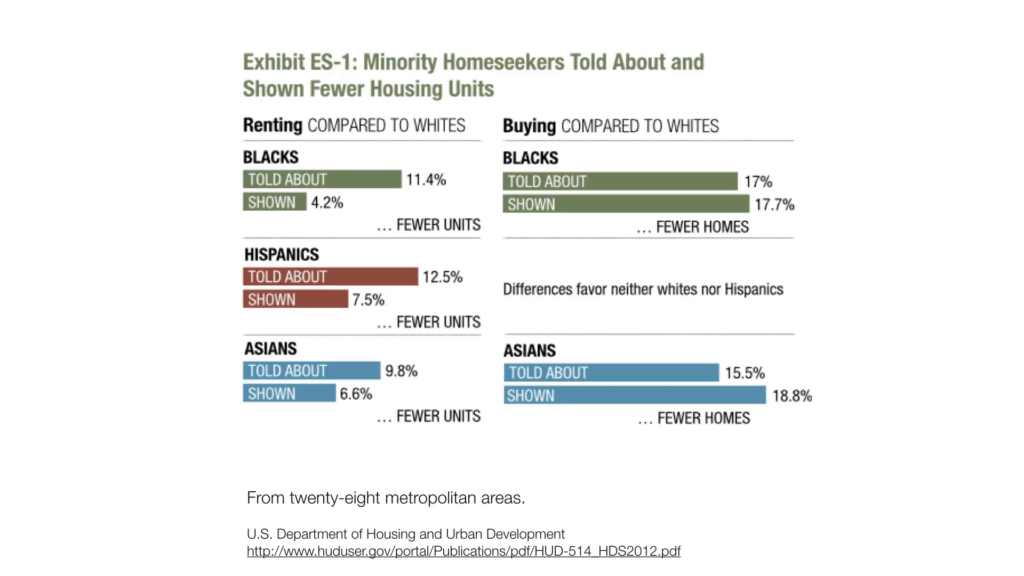
And in the most recent one, following the same traditional audit approach, what they found was that blacks were shown 17.7% fewer homes than whites, and told about 17% fewer homes than whites. Asians were shown 18.8% fewer homes than whites, and were told about 15.5% fewer homes than whites. This isn’t the online world but people physically visiting homes. But imagine going online to a housing site. What could be happening under the hood?, and I’m going to get back to that in a second.
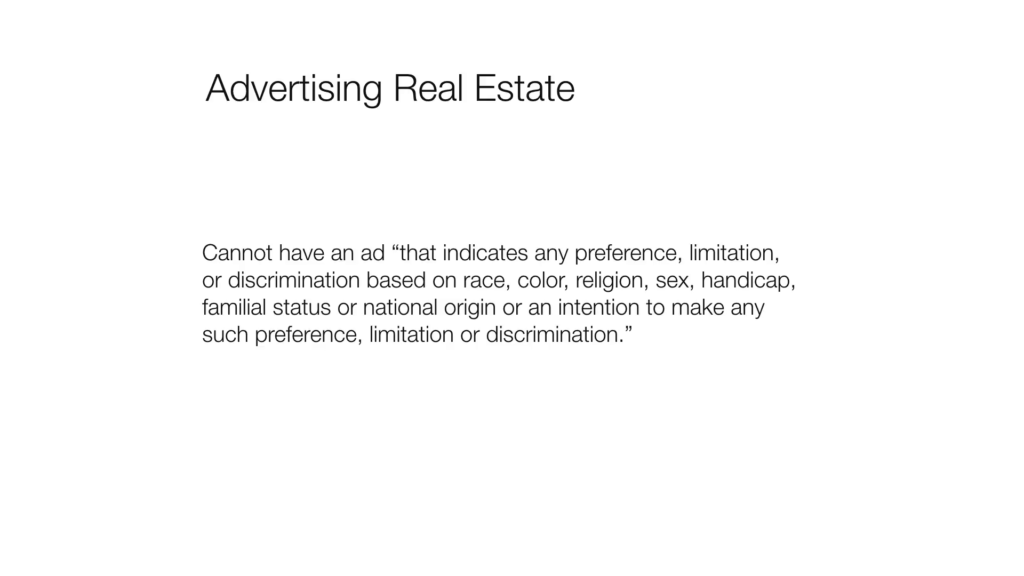
The Fair Housing Act addresses what we see, but it also goes a step further, to address how we advertise to people. So for example you could not have an ad “that indicates any preference, limitation, or discrimination based on race, color, religion, sex, handicap, familial status, national origin, or intention to make any such preference, limitation, or discrimination.” So for example, I cannot put out an ad that says “this is ideal for a white tenant,” “this is ideal for a white female tenant.”
Interestingly enough, we saw that March on Washington, and it’s been over fifty years later and we’re still struggling. In fact there was a case in 1991 against The New York Times where it turns out that they had thousands and thousands of housing ads in their Sunday Times featuring white models. Many of these white models depicted the representative or potential homeowner. The blacks typically represented the janitor, the groundskeeper, and a doorman, an entertainer. And it turns out that in all of these, it was traditionally white families. And so the case was called I believe Reagan versus… Let me find out the exact name of the case before I get it wrong. Reagan versus New York Times 1991. They argued that the repeated and continued of depiction of white human models and the virtual absence of any black human models indicated a preference on the basis of race.
The New York Times fought this for four years before they settled. The Washington Post incidentally changed their policies right away. After that, they basically changed the representations of how they showed models in their newspapers.
So I was really thrilled to see when Rachel Goodman wrote for the ACLU in 2018 that
Thankfully, our existing civil rights laws apply no less forcefully when software, rather than a human decision maker, engages in discrimination. In fact, equipped as they are with the disparate impact framework, the Fair Housing Act (FHA), Title VII of the Civil Rights Act of 1964 (Title VII), and the later-enacted Equal Credit Opportunity Act (ECOA), these laws can make clear that twenty-first century discrimination is no less illegal than its analogue predecessors.
Rachel Goodman, Winter 2018
Now, I was so excited to read this because Rachel is an incredible lawyer. But also because we’ve been trying really hard to fight some of this discrimination online and coming to barriers.
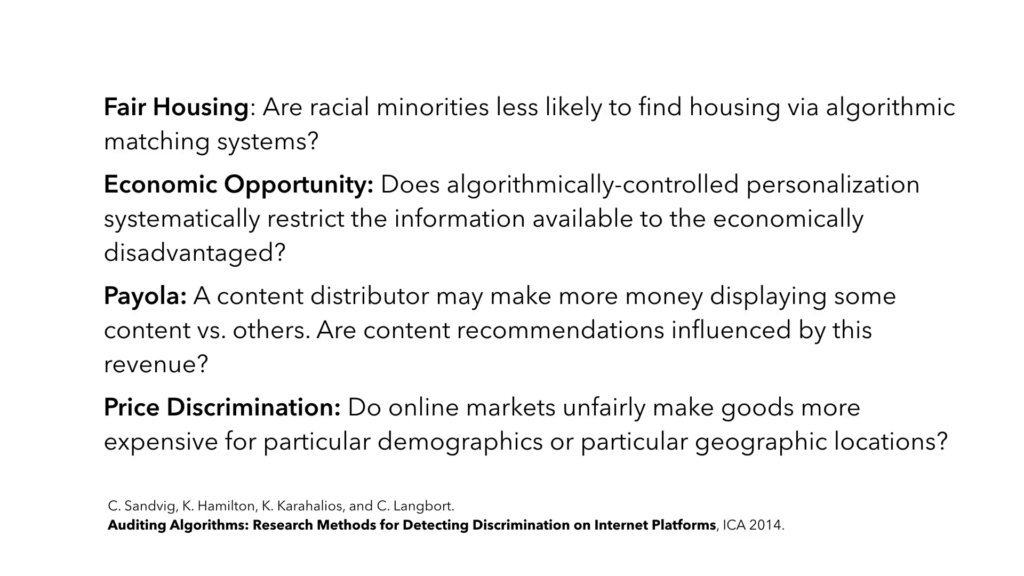
So in 2014, with my wonderful colleagues that I feel privileged to work with, Christian Sandvig, Kevin Hamilton, and Cedric Langbort, we wrote a paper discussing the importance of auditing algorithms and describing the term mentioning why it’s so important coming up with methodologies for doing this and why. One example might be fair housing. Another example might be economic opportunities; can an algorithm actually restrict an opportunity to somebody? Might a type of algorithm that makes more money in one way actually hurt another population? Do some people actually have advantages because of where they live, because of certain algorithmic interfaces?
And we were working on this because more and more of these transactions, whether it be housing, credit, employment, for core social good, are happening online and are happening at scale. And many of these imbalances are not readily visible to the people. We don’t see the separate water fountains. And it’s very very hard to see what’s happening. The creators of these algorithms might not even know what’s happening. And they might not even know that injustice has occurred. You cannot easily compare them.
And so in this paper we describe many different approaches to doing these audits. But two of the big tools were scraping— And many startups today use scraping to get their companies going. And another tool is the sockpuppet. The sock puppet, where you create an identity online. Let’s say I make 200 identities; I make half of them black, half of them white; vary the age ranges; set them out there and see what happens. And Christo Wilson who’s in the audience today has done some amazing work using sockpuppets for price discrimination and for Uber surge pricing. Too many audits to explain in this short talk.
But what’s interesting about these studies is that they mimic the traditional audit studies. And they’re really hard to do. Facebook is not okay with these, you having multiple identities. Google is more okay with it. But you’ll see later that some terms of service make it challenging.
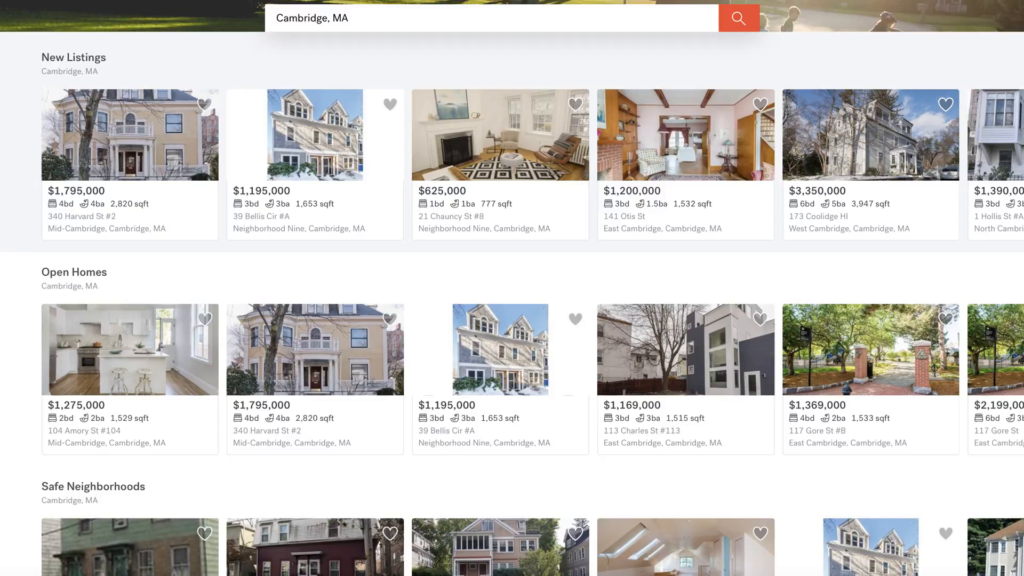
And so by using these tools like the sockpuppets, and scraping, and using bots, and using APIs, we can look at a site like this for housing and maybe try to figure out if some discrimination is happening. Are these homes prioritized differently for different people based on their age, on their sex, and so forth? And it’ll help us actually understand why some of this might be happening.
For example discrimination can occur for many reasons. And the system for example can capture the biases of the humans that use or built it. And even though this study by Ben Edelman— He’s done some amazing studies, by the way. (Sorry to use the term amazing so much, like Trump.) But he studied racial discrimination on Airbnb. And he found that if you had a distinctively African-American name, you’re 16% less likely to be accepted for a home relative to identical guests with distinctively white names.
He also did an interesting study where if you’re a black landlord you make less money than if you’re a white landlord if you control for other factors for the home. And discrimination occurred across all landlords, ones sharing the properties and larger landlords with more properties. And this suggested that Airbnb’s current design choices—and I’m a computer scientist and a designer in human-computer interaction. And the design choices actually can facilitate discrimination and raise the possibility of erasing some of these civil rights gains that people have fought so hard for.
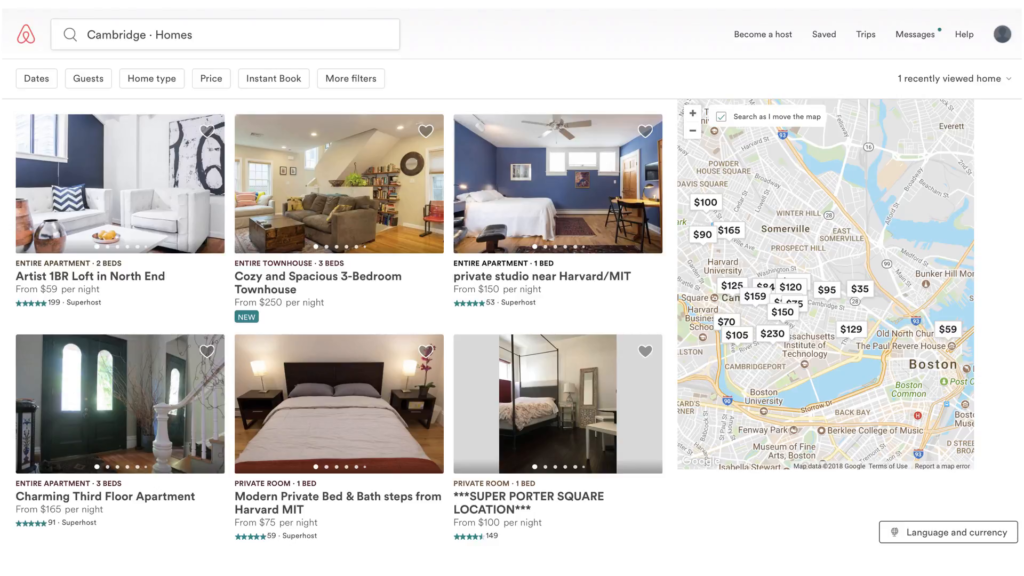
So for example some of things they proposed are not having people’s pictures in here, having statistics up front and then getting into the pictures later. Or suggestions to remove the pictures altogether. But it turns out users hated that so they put pictures back in to satisfy the users. And so here, they were also working on interfaces to change some of these sites. And again, while Edelman’s studies have not looked at the algorithm behind it, they looked at a lot of the inputs.
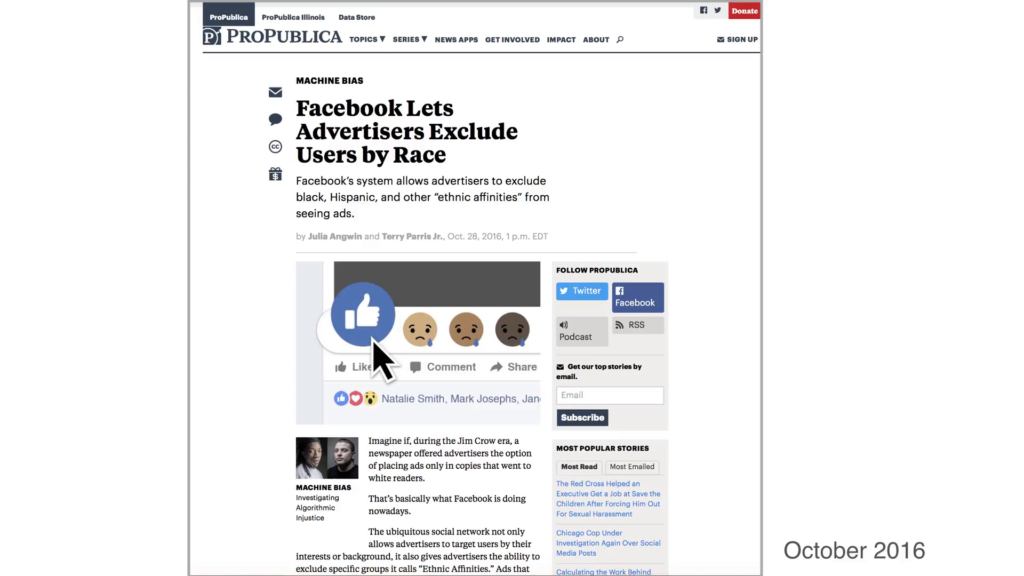
And there’s other places to look at inputs as well. For example here. ProPublica found in October of 2016 that Facebook let advertisers exclude users by race. Now, anyone who could’ve placed an ad on this using many similar sites like Google or Facebook could have known this. But it turns out that people didn’t discuss this idea of what it means to use ethnic affinity as a target variable. And while it’s perfectly legal to target men’s clothing to men, it’s illegal to advertise high-paying jobs exclusively to that same group.
And this is not new in advertising. It turns out that traditionally because of these laws, advertisers have tried to use ZIP code as a proxy, or area code as a proxy, to target specific groups. And you don’t know what might be happening there, especially when you start getting into the machine learning, using machine learning algorithms—specifically deep learning algorithms that use neural nets—where you might have a variable that forms along the way that actually can capture some these characterizations based on other data that you have there. In fact many of the designers don’t even know what’s going on. Some of these layers are now thousands and thousands of layers deep.
And in talking to many developers and engineers of some of these ad targeting systems, I mentioned to them that maybe this shouldn’t be happening, and they looked at me appalled like, “Karrie, we need to use all of the data to get the best possible match.” Many of the developers are not familiar with the law. Many of the developers went on to further counter that perhaps people who are black want to have this type of house and knowing that information could make it better. So, there’s so many levels deep here of having to inform an algorithm literacy, and I’m going to get to that in a bit.
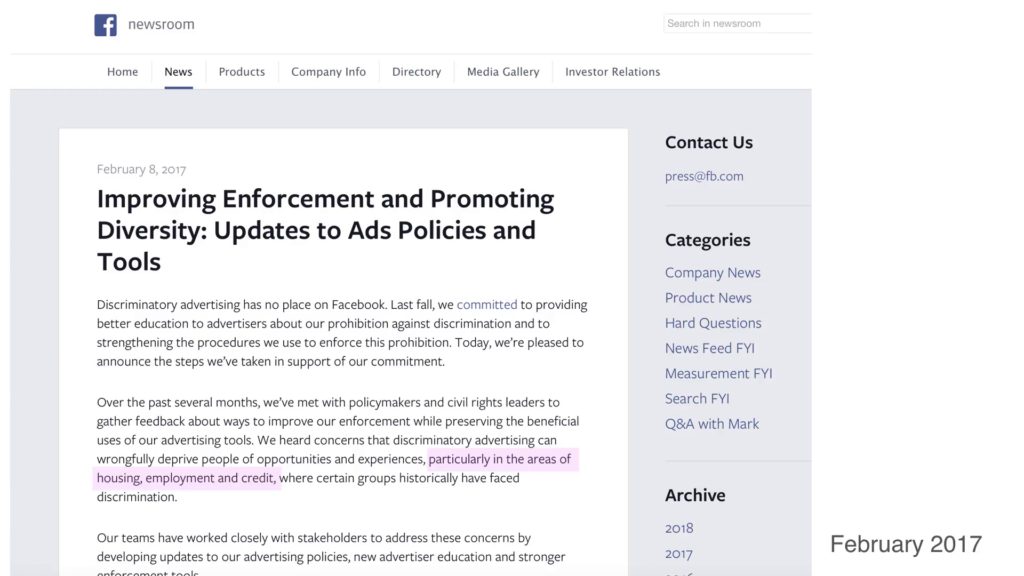
And so because of this Facebook put out a public release that they were appalled. They did not want to help further historical oppression. And they wanted to particularly in the areas of housing, employment and credit, where certain groups were historically facing discrimination, do something about this. And they claimed they went back, they modified their system, so this should not be happening on Facebook anymore. This was February 2017.
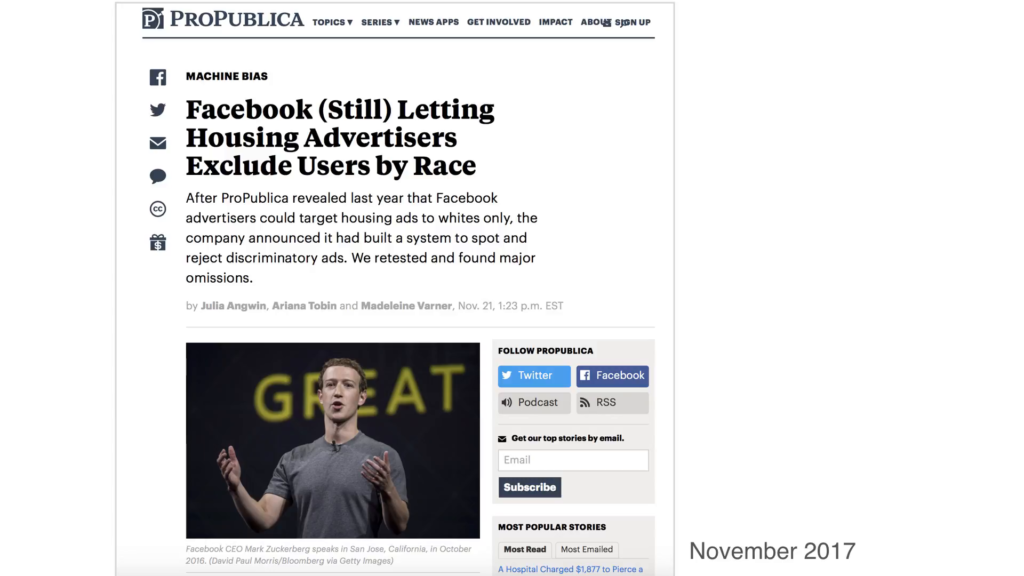
November of 2017, ProPublica went back and said Facebook still is letting housing advertisers exclude users by race. This is calling for more regulation. At some levels this is an interface issue. Also it’s becoming an algorithm issue, because one of the defenses against not being able to capture all of these is it’s hard to know what is housing and what is not. Would an algorithm be used to determine what is a housing ad versus what is an employment ad and so forth. And it’s just going to keep getting much much more complex when these characteristics are inferred from other data, as I mentioned with some of these neural nets and other deep learning systems.
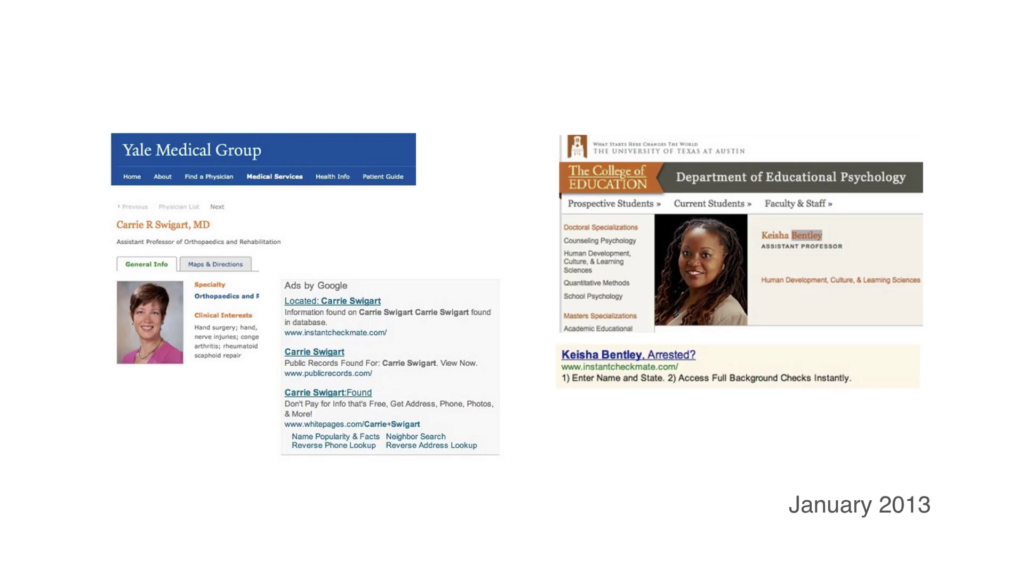
Moving more towards algorithms, Latanya Sweeney’s seminal study looking at algorithm discrimination based on race clearly found that by putting in a white name you got different ads than if you put in a characteristically black name. And this was for many reasons. There’s a bias could be introduced by what people are clicking on this platform. And that’s something to keep thinking about. A system should actually be able to understand that users’ biases might be captured here. And there are no easy solutions to this. Biases are captured in so many different ways. But we need to be aware of them and these audits are so very important.
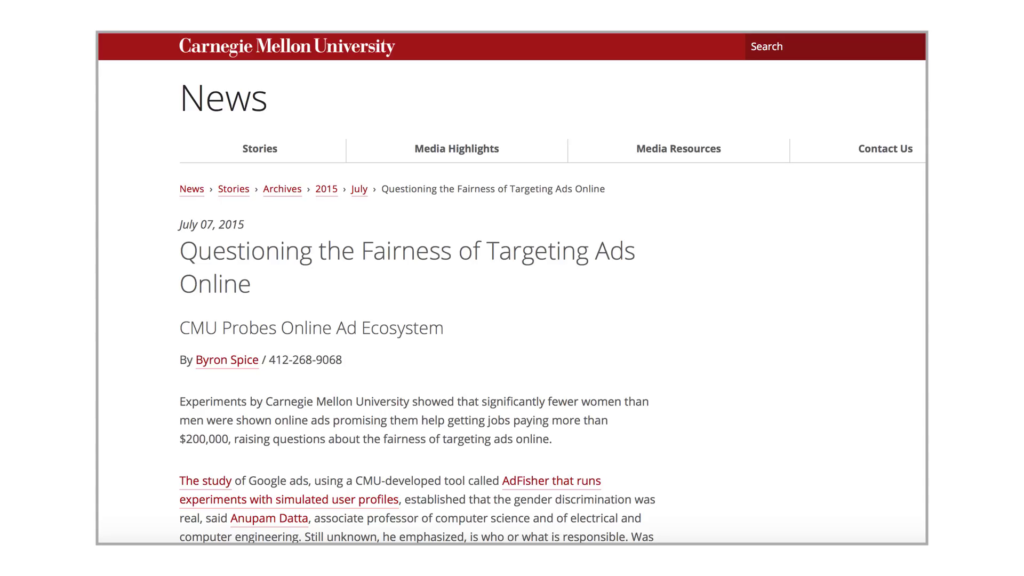
Another example that we touched on earlier and it finally came to pass, it turns out women were being shown ads for lower-paying jobs than men were being shown on Facebook. But again, a lot of this could be based on what people click. A lot of this could be based on what people are targeted towards.
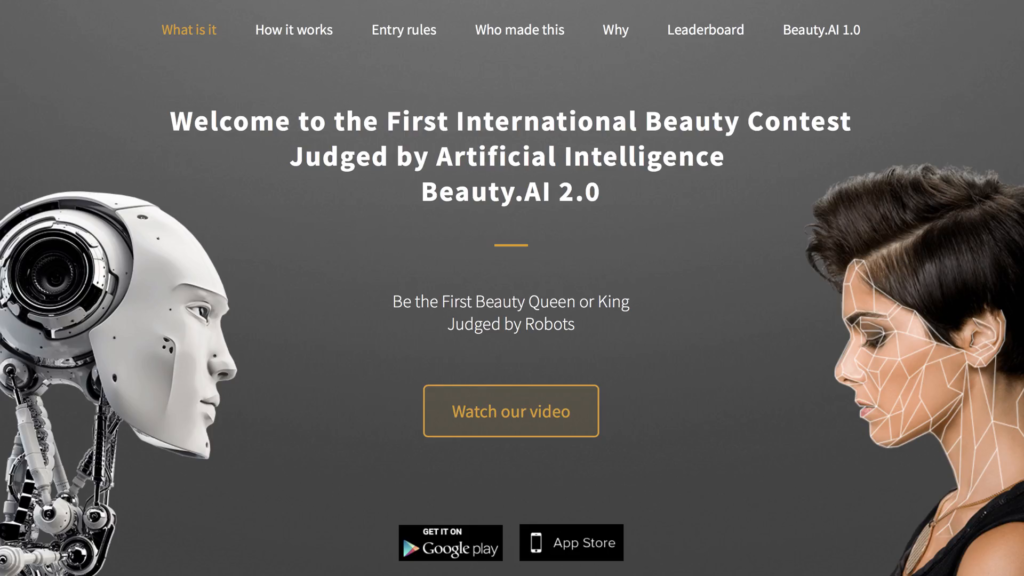
But there’s also another element of bias that comes in that people didn’t think about as recently as 2016. How many of you have seen the Beauty.AI project, out of curiosity? A few of you. This was supposed to be the first-ever unbiased, objective beauty contest, that was just supposed to look at features of your face. It employed deep learning. It employed neural nets with many many different layers. It was sponsored by many respectable communities, got lots of money. All over the place. Over 6,000 people submitted photos to this.
Of the people that submitted photos from all over the world, out of the forty-four winners nearly all were white. There were four Asians, I believe. Only one had dark skin. And many many people were— These photos like I said were sent from all over the world.
There were a number of reasons for why the algorithm favored white people. The main problem, and admitted by the developers, was that the data the project used establish standards of attractiveness, their training set, did not have black faces. So for example, how can you have an objective algorithm if your training set is biased? While the algorithm had no rule in it to actually treat a different skin color differently, the training set it used steered it and taugh it that white faces were more attractive, despite using many of these other parameters as well. The developers of this tool stated this and were like, “We kinda forgot that part in our algorithm.” It’s not just the algorithm, the training set matters as well.
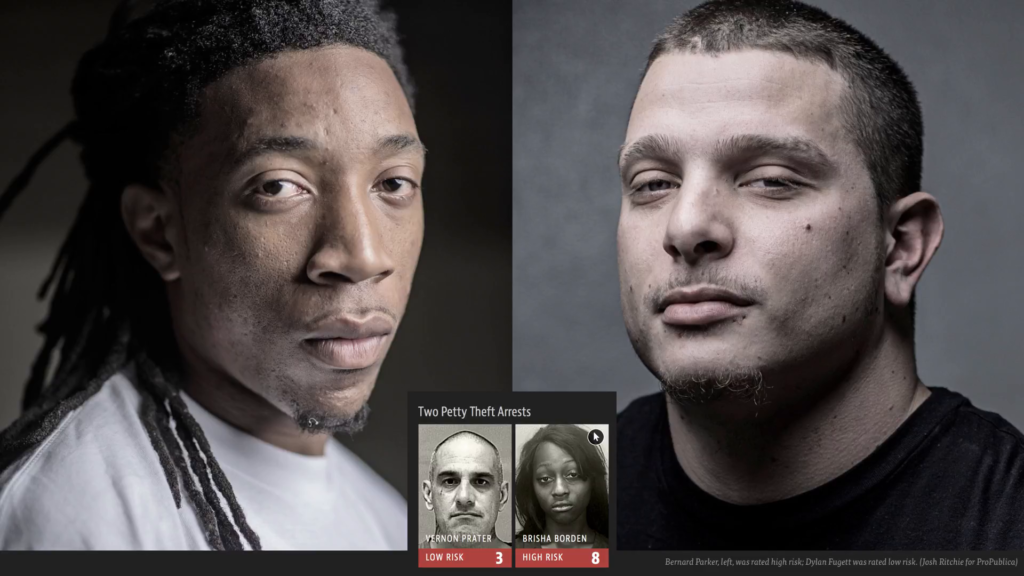
And this is an example I know I don’t need to tell to most people in the room. We can’t capture all forms of bias. But a first important step is to audit these systems. And ProPublica has been a public leader in this, and I’m so excited and I can’t wait to see more of what they do. They published this article in May of 2016 looking at recidivism rates. They did not have— We do not have access to a lot of these algorithms. They’re black boxes to us. We cannot see inside of them. And that’s why it’s so important study them from the outside.
And what they found, that this recidivism score, the likelihood that people were to commit a crime again, was falsely flagged for black defendants moreso than white defendants. In fact white defendants were mislabeled as low risk more than black defendants. Such systems, for those of you don’t know, are in use in many states today. They dictate who can stay home before a trial. In some cases how long your sentence should be. And even though after interviewing the designers of this system, they claimed they did not design to be used in this way. Systems are being used in this way.
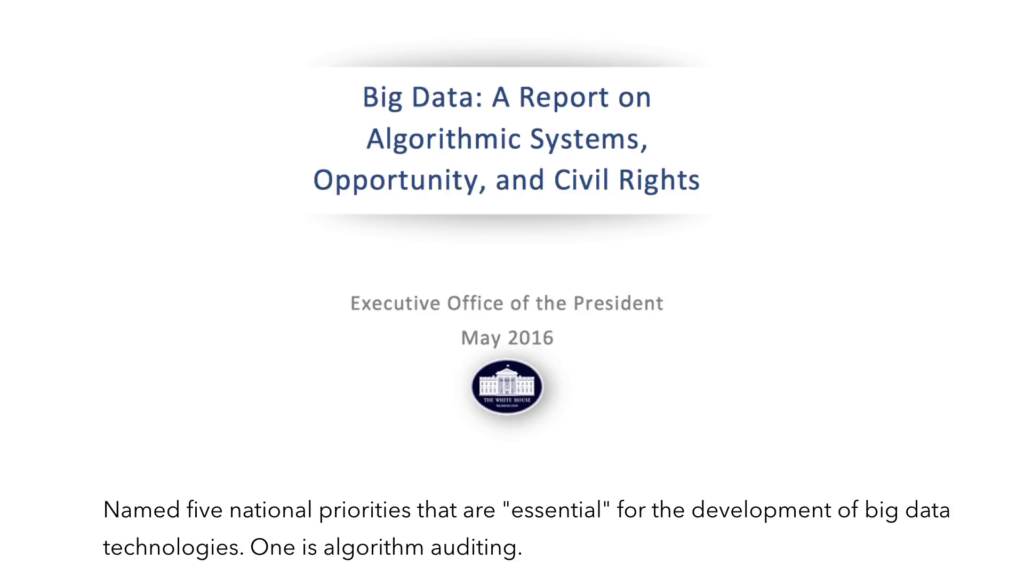
And so, doing years and years of these audits we got really excited in May of 2016, when the White House released this press release on big data. And they named five national priorities that are essential for the development of big data technologies. And one was algorithm auditing, and they cited our paper, and we were doing somersaults. Many other things they looked at were an ethics council, an appeals process, algorithm literacy, and so forth. And in their document they wanted to “promote academic research and industry development of algorithmic auditing and external testing of big data systems to ensure that people are being treated fairly.”
Again, we were so excited by this. And especially to keep moving with some of the algorithms that we had in place. But then we got stuck. Because a lot of what we’re doing, such as web scraping and the creation of the sockpuppets, it turns out that many courts and federal prosecutors have interpreted the law to make it a crime to visit a web site in a manner that violates terms of service or terms of use established by a web site.
We did not want to be criminals. I do not want to ask my students to do something that might be a federal crime. And yet we still think that it’s so important to get some of this work out. This causes a lot of concern, causes a lot of fear, and in many cases it actually closed paths of research and closes avenues available to us to do this work and to do it much more cheaply. Despite the fact that we had no intent to cause material harm or to target web sites’ operations, and have no intent to commit fraud or to access any data information that is not public.
Just to give you a hint, for those of you not too familiar with some terms of service rules, one thing that I want to state is that the scraping and some of these violations of terms of service, since Ethan mentioned norms, are a norm in computer science. Nonetheless, our research group received legal advice from top lawyers recommending that such scraping was almost certainly illegal under CFAA. And we were told that if ever we received a cease and desist letter, that we should stop. If we ever had our IP addresses—and they’re blocked almost all the time—we should stop. And they also conscientiously asked us to do back of the envelope calculations on what load we were putting on the web sites. And if the load was too high, then just to stop. In another project a while back, a lawyer so much as told me not to read any terms of service so that I could have plausible deniability in case I were ever contacted.
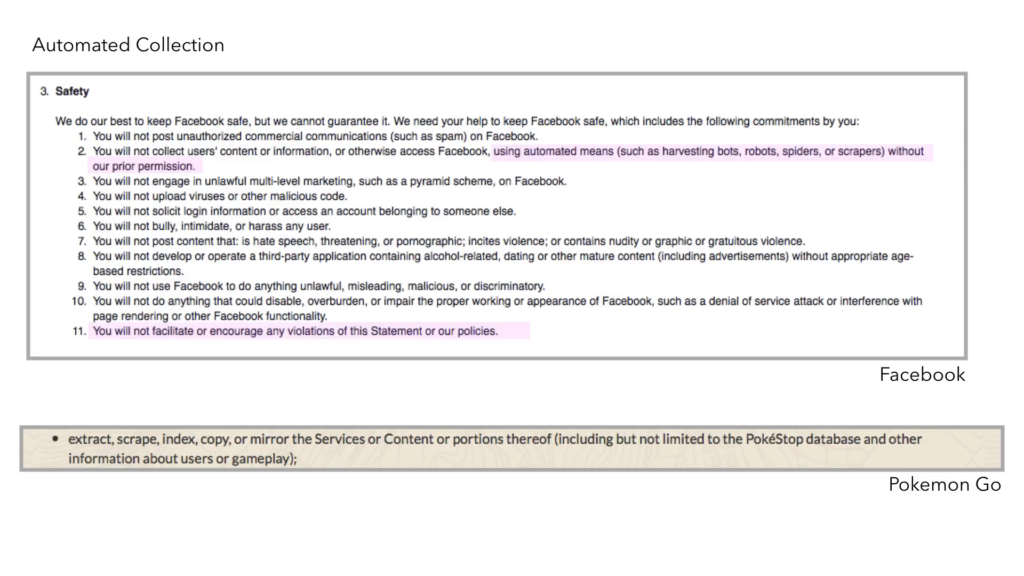
But just some examples of terms of service. Automated collection is forbidden. So this is Facebook on top. I cannot use “automated means (such as harvesting bots, robot, spiders, or scrapers) without prior permission.” Furthermore, I will not “facilitate or encourage any violations of this statement or the policies.”
Pokémon GO: you cannot “extract, scrape, index, copy, or mirror the services or content.” This gets at our scraping tool.
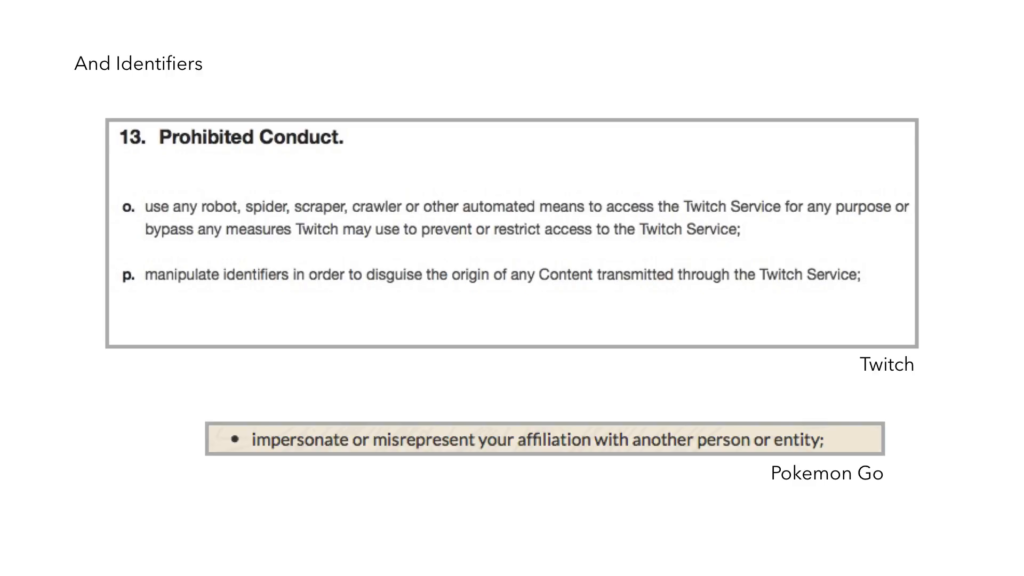
In terms of identifiers or sockpuppets, it turns out I cannot “manipulate identifiers in order to disguise the origin of any content transmitted through the Twitch service,” or “impersonate or misrepresent my affiliation with another person or entity.”
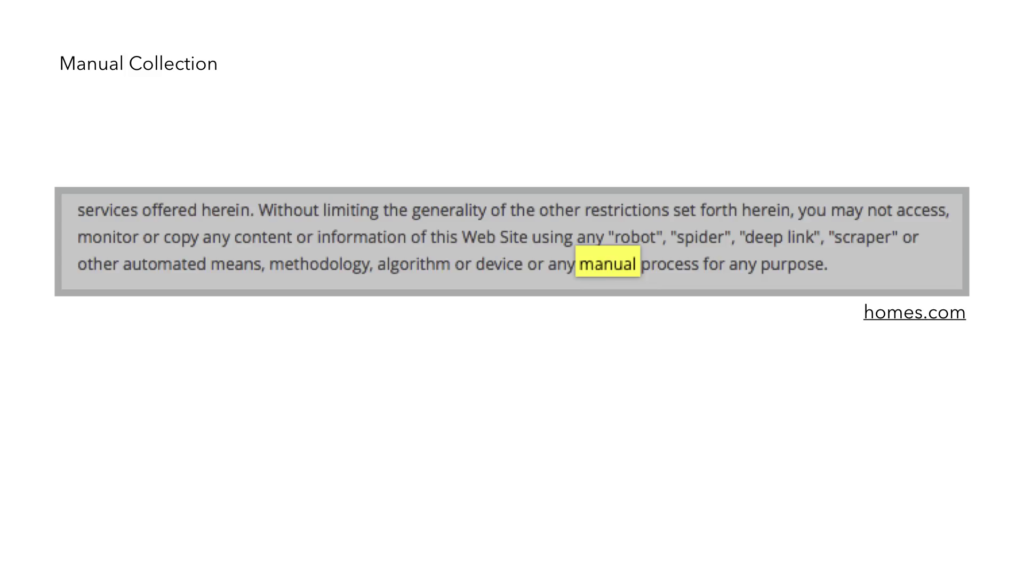
Furthermore, in some cases they go so far as to say that I cannot use a scraper, “automated means, methodology, algorithm or device or any manual process for any purpose.” So does that mean I cannot write something on a piece of paper? And so Ethan, while I’m a big fan of the law, I don’t think it’s very clean. And I would love to discuss that further.
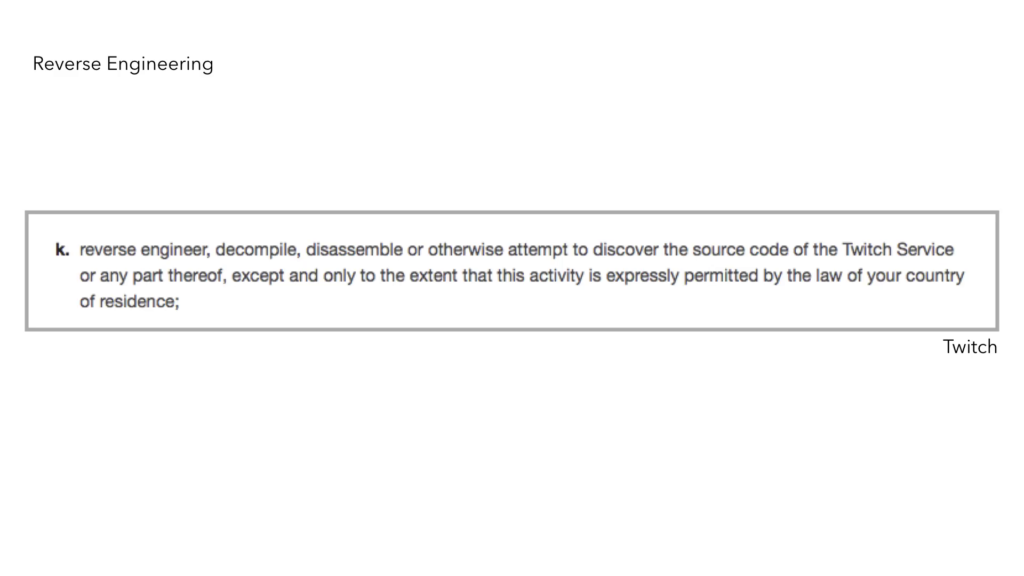
In this case I cannot reverse engineer, something that many computer scientists do all the time with black box systems. In [fact] it’s encouraged in the security community. You cannot do security research unless you do some of this.
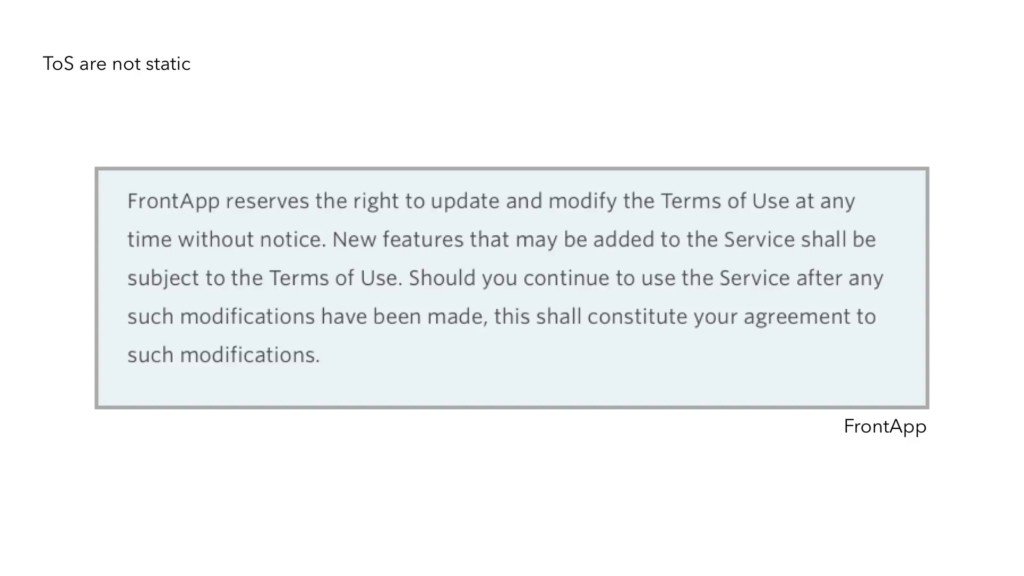
Also, terms of services change. This app reserves the right to update and modify terms of service at any time without notice. I was once working with a company for six months, and when I started the project they were so excited. They’re like, “This is great work. We support you. This is amazing.” A year later the same people told us, “Um, you’re now violating terms of service.” The excitement had turned to less excitement, and expressed caution.
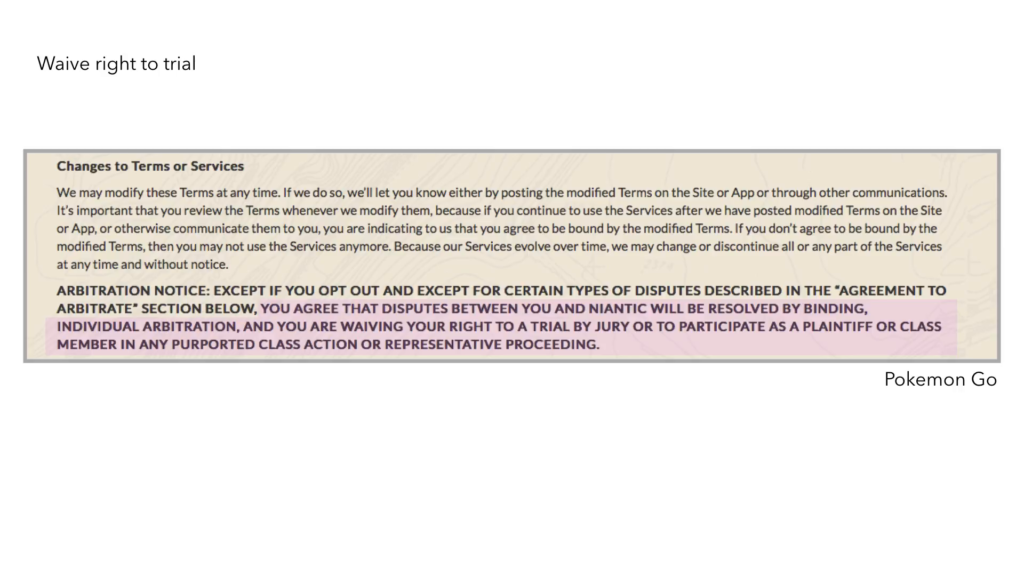
Furthermore, in some cases you actually can waive a right to a trial. Here “you agree that disputes between you and Niantic will be resolved by binding, individual arbitration, and you are waving your right to a trial by jury or to participate as a plaintiff or class member” in any later proceeding.
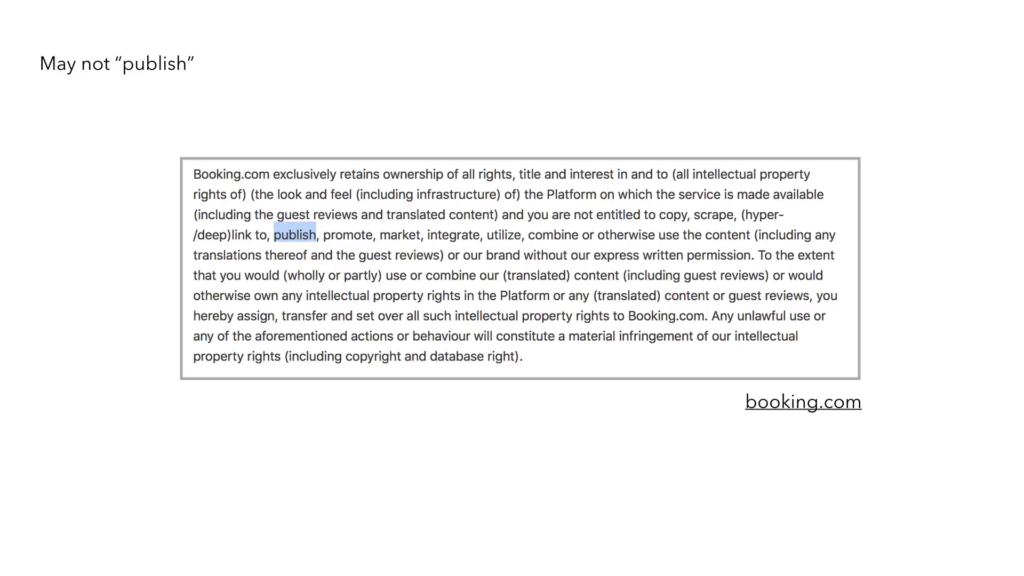
In this case, one lawyer actually told me that I should not publish a paper I was doing because not only can I not copy or scrape, but I cannot publish or promote and “publish” might mean publishing a research paper.
And so you know, there’s other interesting obstacles that keep coming into here. And so this actually… The terms of service are often couched under the Computer Fraud and Abuse Act, which criminalized certain computer acts. And certain uses of the CFAA, or the Computer Fraud and Abuse Act, have been denounced by researchers because of our norms. Because we could all go to jail for security research or discrimination research at any moment and a jury could happily convict us.
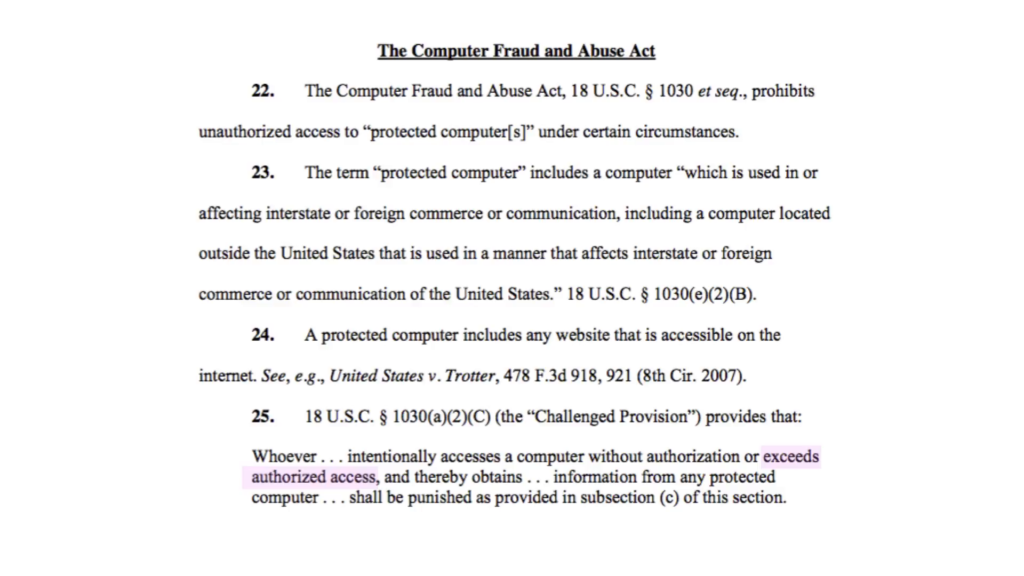
So what is happening in this law? Basically, it prohibits unauthorized access to protected computers under certain circumstances. But the key that’s interesting here for us is that “whoever intentionally accesses a computer without authorization or exceeds authorized access, and thereby obtains something…” It this “exceeds authorized access” phrase which was confusing. And it’s been repeatedly interpreted by courts in federal government to prohibit accessing a publicly-available web site in a manner that violates the web site’s terms of service. If you do, the first violation carries a one-year maximum prison sentence and fine. Second or subsequent violation carries a prison sentence up to ten years and fine. And intent to cause harm is not considered.
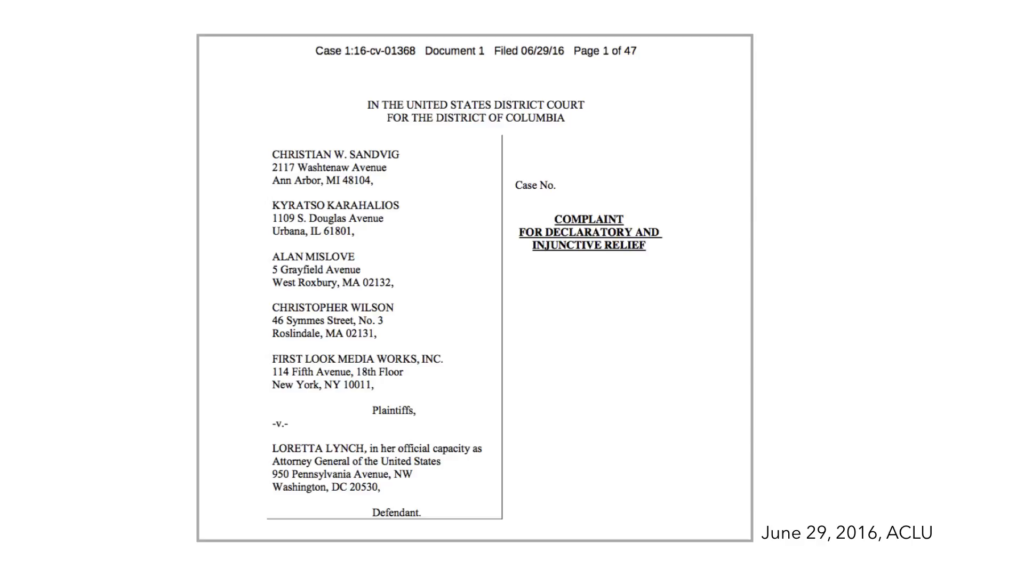
And so, with some colleagues, Christian Sandvig, Alan Mislove, and Christo Wilson, who’s in this room, we filed a lawsuit with the amazing lawyers of the ACLU basically trying to understand the broad and ambiguous nature of this law that prohibits and chills a range of speech and expressive activity that is protected. It protects individuals from conducting robust research on issues of public concern when websites choose to forbid such activity. It could also violate the First Amendment and due process clause of the Fifth Amendment to the US Constitution.
We filed this lawsuit in June 29 of 2016. In September 9 of 2016, the government filed a motion to dismiss. One month after that, they released a previously non-public document that they had made in 2014 suggesting their policy for when the target CFAA crimes. And the example of something they discussed in this document is that one would not get prosecuted for lying about their weight on a dating site, even though that violates terms of service.
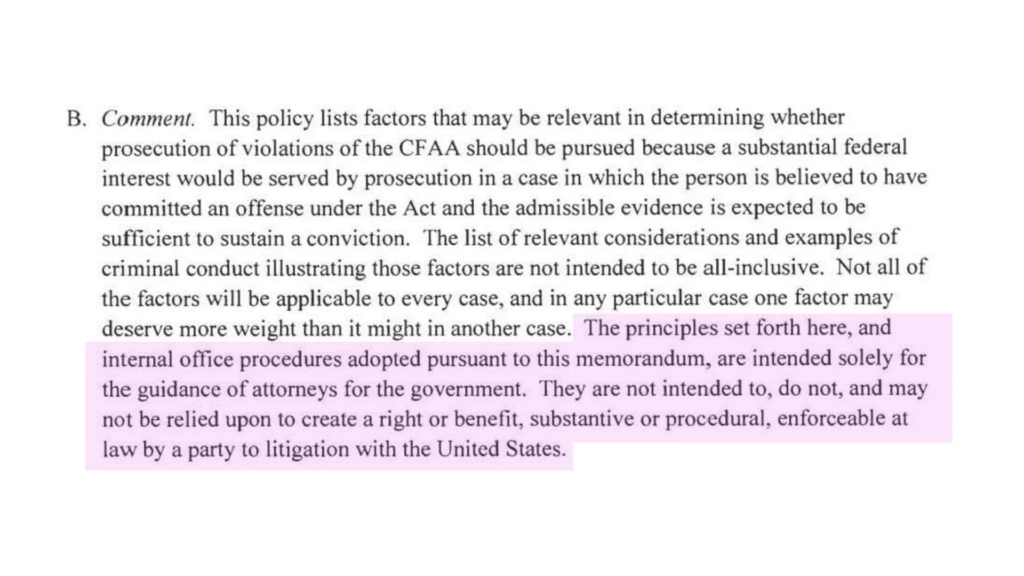
However, in the link for this they also say “the principles set forth here, and internal office procedures adopted pursuant to this memorandum,” are solely guidelines. They do not protect anyone, specifically researchers. And that was our goal here. I’m not a lawyer. After this process I really wish I was a lawyer. If you want to know details of the case, please look at the web site. The lawyers have put up all the public documentation for it.
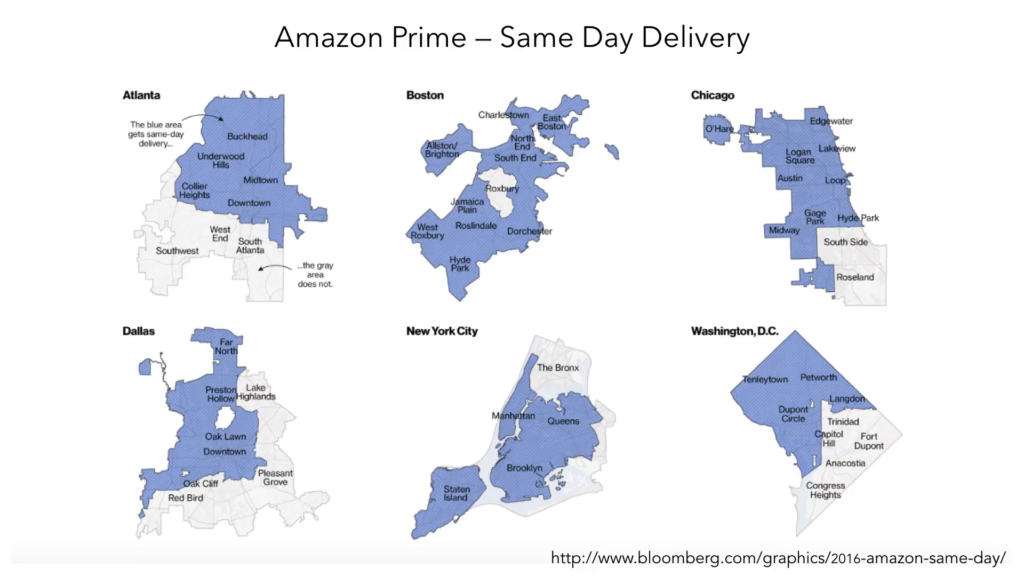
And in closing, I want to say that this has not stopped us from building these tools and I think we should all keep building these tools. Similar to the images in the beginning where a bunch of people came together collectively, one of our big focuses at the moment is building collective audits. This idea where people come together and actually reappropriate a site—for example we explored a hotel booking site and found that they biased low-rating to middle-rating hotels upwards by 25%.
And so what we also found was that by looking at the conversation people were having, we weren’t the first ones to find this. The people, you had found this bias before us. And by looking at the conversations on there, you helped us find it and make it public. And not only that but people were reappropriating this site and saying, “This is what the site says my rating is, but this is what it should be.” And they were actually making it their own platform as opposed to what it was before.
So we also need geographical tools to address these so that what happened with Amazon Prime’s site does not happen today. We need tools that actually let people be able to interrogate their own data, which they cannot do today. And we want people to be able to collect data societally, to help us make these collective audits. And so I’m gonna end there and say that we want people to come together not just to protest but to share and to have a voice. And thank you. And I also want to thank the ACLU for helping us with all of this. And all the students involved. All the faculty involved that I’ve worked with. And the community at large like the MIT Media Lab, like the Berkman Center, that have actually been very very supportive of this work.
Further Reference
Gathering the Custodians of the Internet: Lessons from the First CivilServant Summit at CivilServant