This talk is about technology that goes under various names: emotional robots, affective computing, human-centered computing. But behind all these is actually the technology for automatic understanding of human behavior, and more specifically human facial behavior.
The human face is simply fascinating. It serves as our primary means to identify other members of our species. It also serves to judge other people’s age, gender, beauty, or even personality. But more important is that the face is a constant flow of facial expressions. We react and emote to external stimuli all the time. And it is exactly this flow of expressions that is the observable window to our inner self. Our emotions, our intentions, attitudes, moods.
Why is this important? Because we can use it in a very wide variety of applications. So, everybody wants to know who the person is and what is the meaning of his or her expression, and use it for various applications. When it comes to analysis of our faces in static face images, identification of faces, this problem is actually considered solved. Similarly, we can say for facial expression analysis, in frontal view videos that the problem is more or less solved.
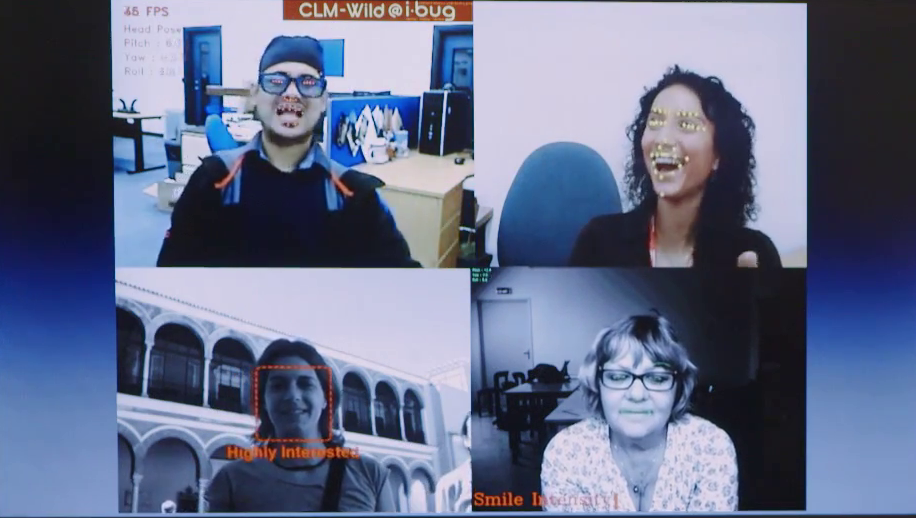
Clip playing on-screen at ~1:37–1:49
As you can see from these videos, we can accurately track faces in frontal views, and even judge expressions such as frowns, or smiles, high-level behaviors like intensity of joy, or intensity of interest, even in outdoor environments. However, when it comes to completely unconstrained environments where we have large changes in head pose, and when he have occurrences of large occlusions, then we are facing a challenge. We call this problem automatic face and facial expression analysis in videos uploaded [to] social media like YouTube and Facebook.
We have to collect a lot of data in the wild, annotate this in terms of where the face is and where the parts of the face are. And then build these multi-view models that will be able to actually handle these large changes in head pose. We also need to take the context in which a facial expression is expressed into account in order to be able to deal with the subtle facial behavior, or with occlusions of facial expressions. So, context-sensitive machine learning models are the future.
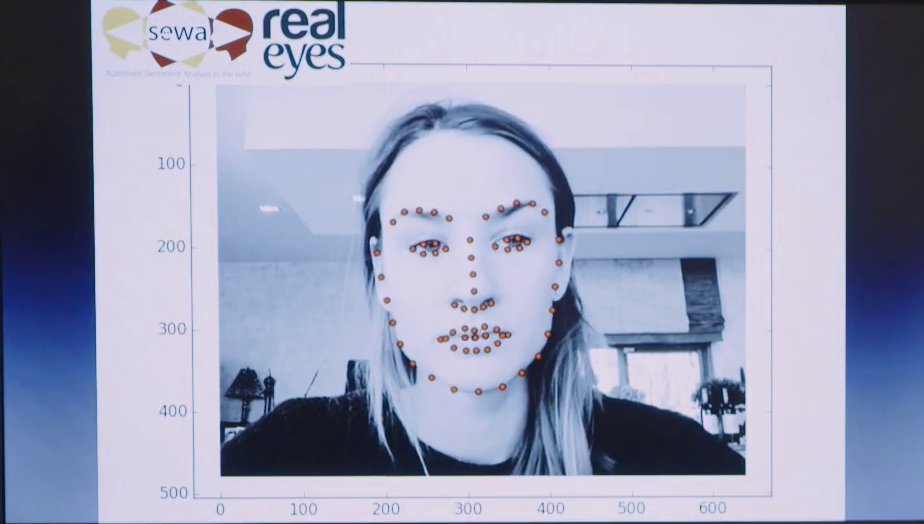
Clip playing on-screen at ~2:48–2:56
Nonetheless, the technology as it is now is still very much applicable to a wide variety of applications. A good example is market analysis, where we could use the reaction of people to products in adverts in order to judge the successfulness of these products in adverts. The software is commercially available [from] Realeyes, and we are working with this company in order to include verbal feedback about products and adverts as well, and to build another tool for skill enhancements such as conflict resolution skills.
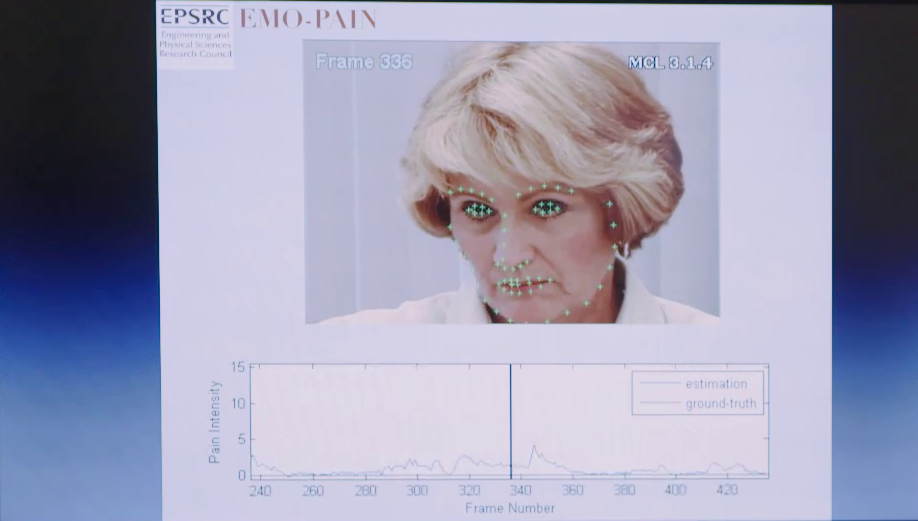
Clip playing on-screen at ~3:28–3:44
Another very important field in which work quite a lot is the medical field, and we currently have the technology available for automatic analysis of pain and intensity of pain from facial expressions. We use this in visitor physiotherapeutical environments, but we could also use that for intensive care.
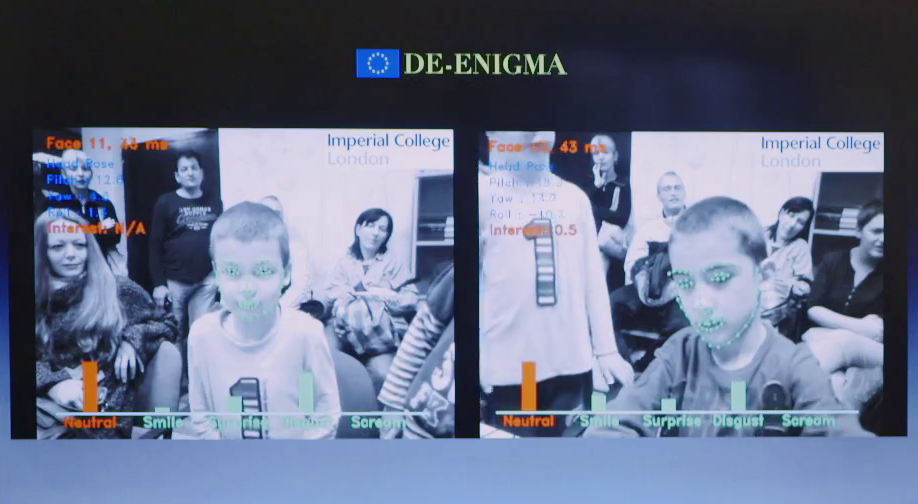
Clip playing on-screen at ~3:50–4:07
Another important project for us is the European Commission project on work with autistic children, where we would like to help the kids to understand the facial expressivity of themselves and others by using social robots with which they interact. These robots will have a camera which will watch them, and the software that will interpret these expressions and give them feedback.
In any case, once this technology really becomes mature and we can truly do face and facial expression analysis in the wild, we would be able to have a lot of applications, such as for example, a system for analysis of a negotiation styles or managing styles. Or simply measuring the stress in job interviews, in car environments, in entertaining environments, and then increase distress if people find this entertaining, or decrease it in order to increase the safety of the driver and the patient. So that’s just to mention a few examples.
Thank you very much for your attention.
Further Reference
Maja Pantic profiles at Imperial College London, and Realeyes.
iBUG, the Intelligent Behavior Understanting Group at Imperial College London