The United States plants more than 170 million acres of corn and soybeans a year, more than any country in the world. And the primary mechanism in the US that we use to subsidize agriculture is actually called the Federal Crop Insurance Program. So, the crop insurance program in the US is also the largest such program globally, with over $100 billion in liabilities annually. So it’s a very big program.
The way that crop insurance works, basically, is it insures farm yields and revenues from loss due to say, bad weather or low prices. It’s a public/private partnership, which works where the government basically sets the premium rates, private companies deliver it, and then farmers pay part of the premium.
So, the primary goals, historically, have been to improve, increase maximization of participation in the program, but less focus has been on the modernization of the rating system. So I’ll just give you an example of how it’s sort of crude in some ways.
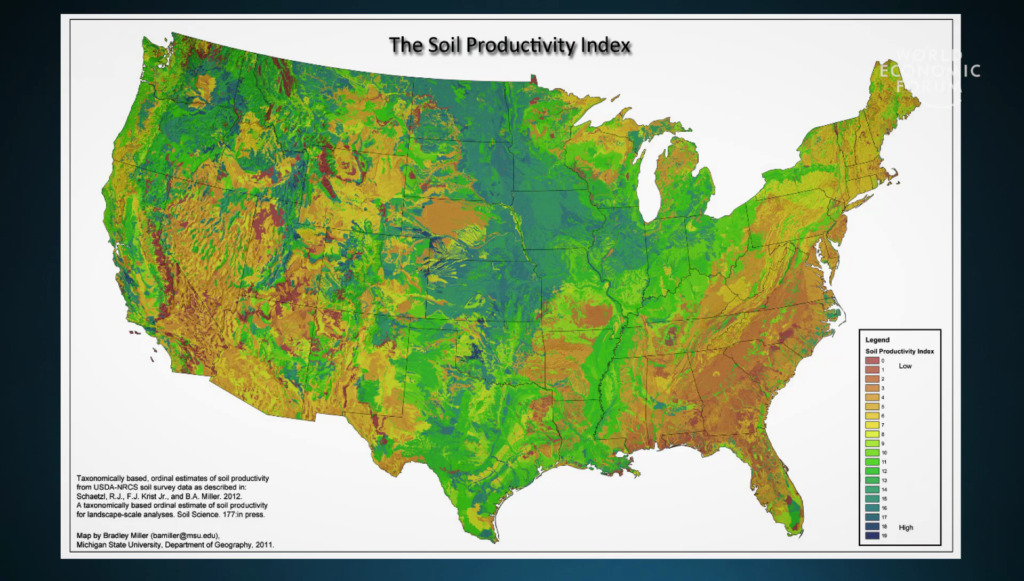
The government sets base premium rates by region but doesn’t necessarily take into account the actual soil on the farm. This could be very important and could involve a lot of work to rework work the rating system, but could have some important implications. The fact that the program has been very successful in maximizing participation, it’s fair to say that far less focus has been on aligning sustainability goals in the program.
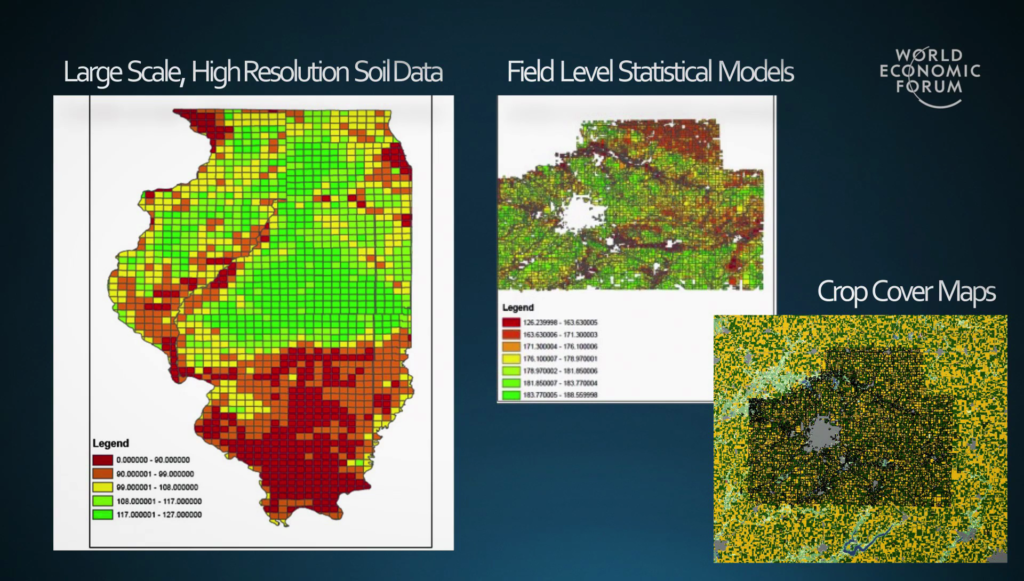
So this raises the question, can we bring big data tools to bear to help align these goals in some way? So, recently we did a study seeing if we could integrate in high-resolution soil data to improve the rating. And so by integrating these big data sets together, we were able to come up with precise field-level estimates of risk. It turns out that the rating accuracy can be dramatically improved by incorporating these data. And so omitting them can result in premium errors on the order of billions of dollars a year.
This is really important because insurance prices can affect incentives in much the same way that prices can affect incentives in any market. So getting the rates right is potentially very important. In doing a lot of work on this, we found that we could probably rebuild the program, even though it might be somewhat difficult. But what it might enable is basically facilitating promotion of soil health and other things through crop insurance incentives, rewarding farmers for improving soil quality while simultaneously building a stronger program.
If we could do this, though, why would this even matter? Some people have said that having these risk management programs basically creates disincentives for farmers to adapt to say, climate change. The reality, though, is that having well-functioning credit risk and management markets is really actually critical to enabling investments in technologies to enable farmers to adapt.
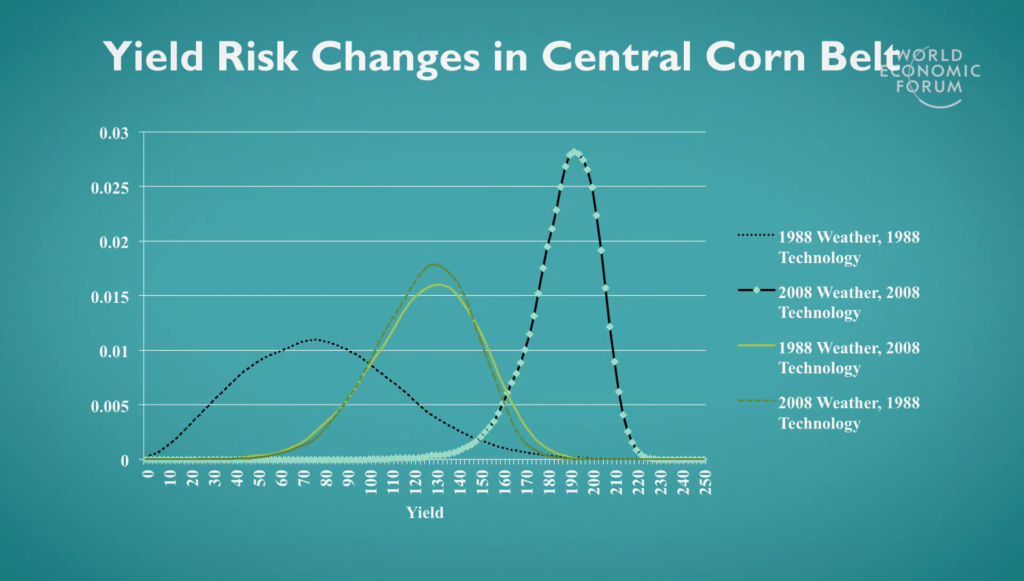
So indeed, in the US what we found in the highest productivity regions is that we’ve seen increases in productivity and decreases in risk, actually, due to the massive gains we’ve seen in biotechnology and management and so forth. A lot of that is exactly because we have these well-functioning markets to facilitate that. So it’s really important.
But yet despite the fact that the underlying structure of the crop insurance program, which is the main way we subsidize agriculture, is still fairly low-tech. Think about crop insurance as a conduit to promoting sustainability. Some gaps must be bridged, and those gaps are integration of big data tools with smart policy designs.
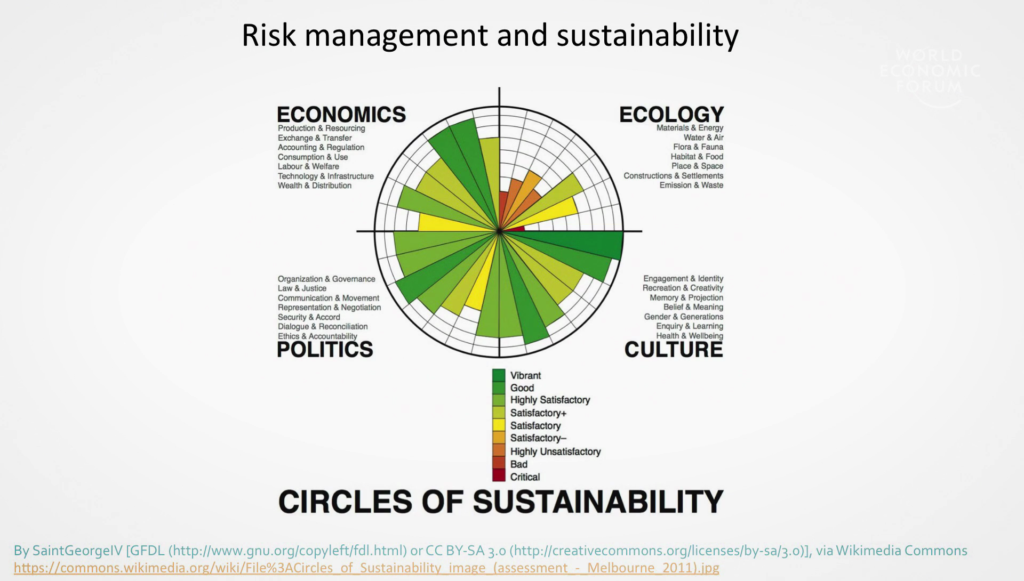
The opportunities here are also not just limited to soil health. There’s a variety of areas right now where advanced computational methods are being brought to bear to improve the functioning of these types of programs. Everything from integrating genetic data, to remotely-sensed data, and others.
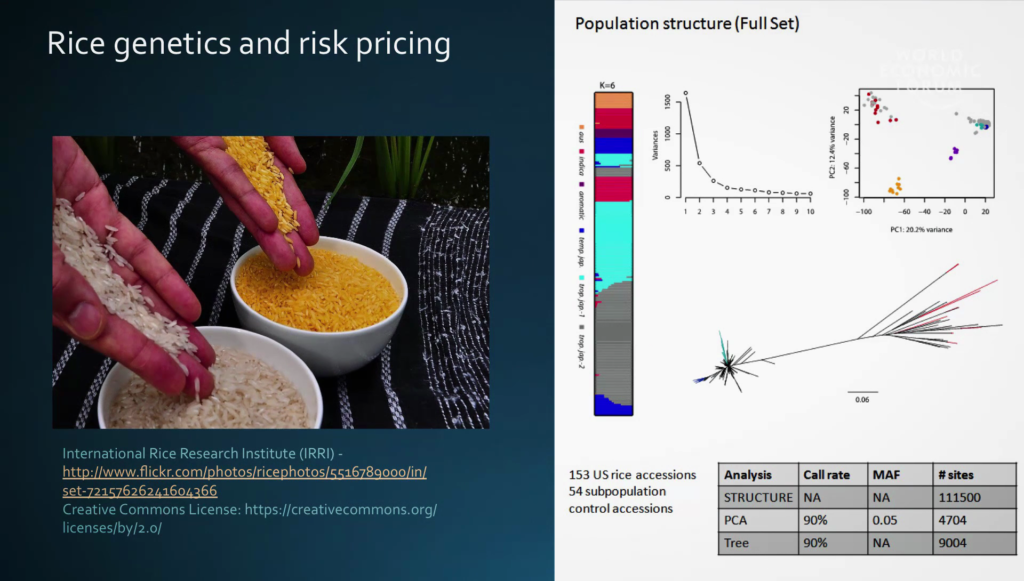
So for example, recently we just did a study where we were able to integrate high-resolution genetic data with high-resolution large-scale yield data for all of the rice in the US grown in the last four decades. And this enabled us to do genetic-specific insurance pricing at the actual snip level, not just on traits. Amazing advancements that ultimately could help breeders and also improve the program.
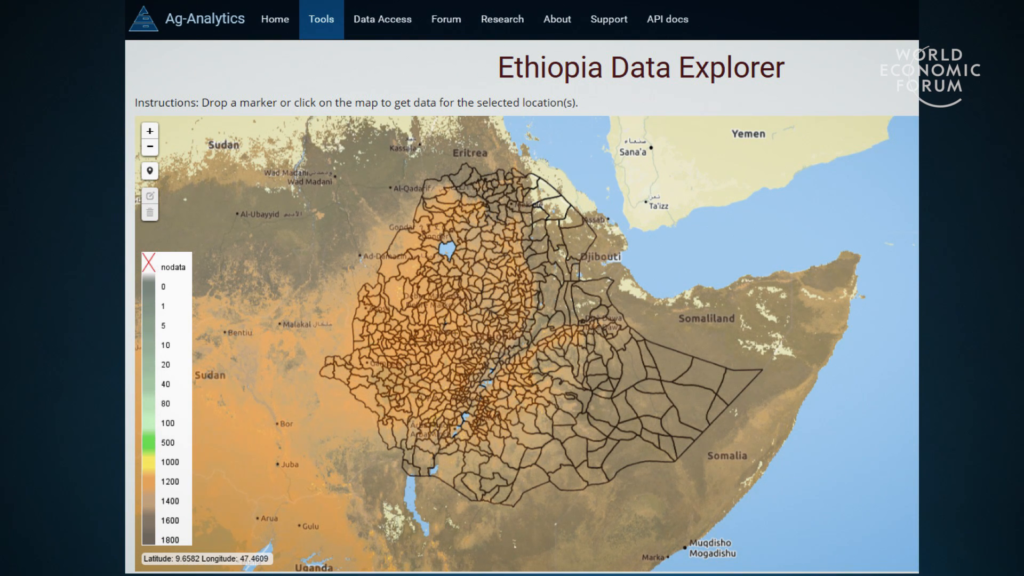
This is also being brought to bear in developing countries, where we’re helping improve and create risk management markets, or we use remotely-sensed satellite data to basically structure insurance contracts for pastoralist livestock producers. We have a lot of tools these days to do that. I mean, we can rate and price insurance from the genetic level, at the soil level, and even literally from space, which is really exciting to have these tools to bring to bear. But more can be done, I think, in terms of aligning the design of these programs to promote some of these goals that we want to reach.
Precision agriculture and government policy and risk management markets, these all have really important roles to play in both intensification and conservation in agriculture. And policy in particular has a very profound role in mediating this intensification/conservation dynamic. And advanced analytics sort of in some ways bring to bear the keys to unlocking these.
So my question to you is what are the opportunities to bring data analytics to bear to improve risk management and policy in your markets? Thank you.
Further Reference
Joshua Woodard profile, Dyson School, Cornell University
2016 Annual Meeting of the New Champions at the World Economic Forum site