I’ve found that identifying ways to be a part of shared collaborative and community projects that produce knowledge or build infrastructure that has been influential and instructive for me has connected me to a lot of really amazing people and ideas. And so, most relevant to this presentation I want to talk about how my more recent work as an open source maintainer has actually helped me learn more about how to be present in communities that are important to me.
Archive (Page 1 of 2)
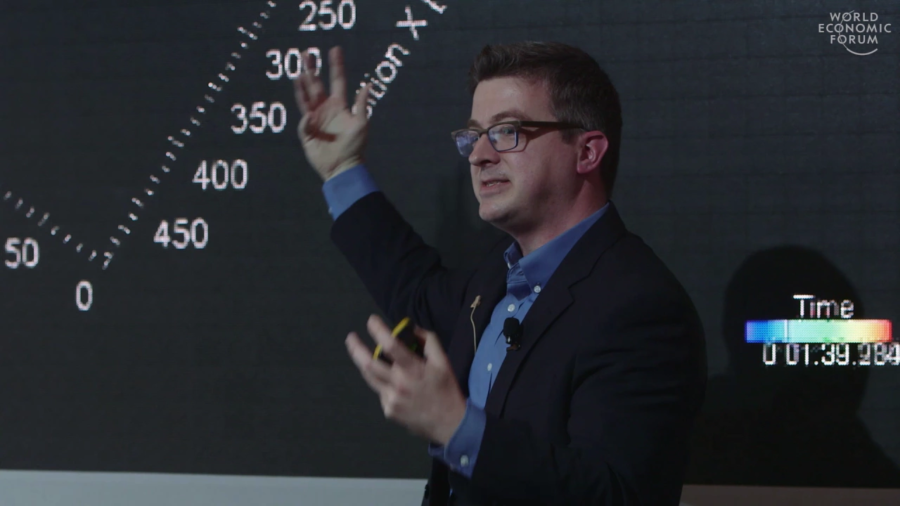
The goal of MICrONS is threefold. One is they asked us to go and measure the activity in a living brain while an animal actually learns to do something, and watch how that activity changes. Two, to take that brain out and map exhaustively the “wiring diagram” of every neuron connecting to every other neuron in that animal’s brain in the particular region. And then third, to use those two pieces of information to build better machine learning. So let it never be said that IARPA is unambitious.
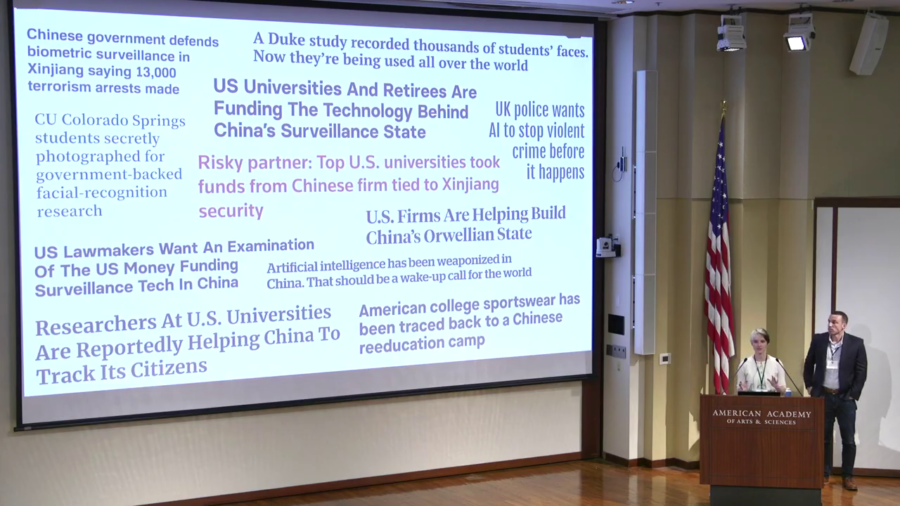
We wanted to look at how surveillance, how these algorithmic decisionmaking systems and surveillance systems feed into this kind of targeting decisionmaking. And in particular what we’re going to talk about today is the role of the AI research community. How that research ends up in the real world being used with real-world consequences.
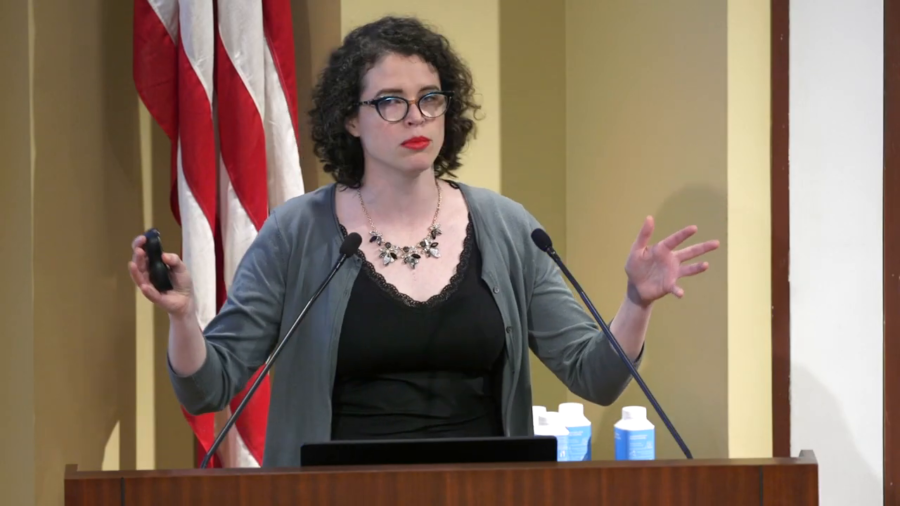
Positionality is the specific position or perspective that an individual takes given their past experiences, their knowledge; their worldview is shaped by positionality. It’s a unique but partial view of the world. And when we’re designing machines we’re embedding positionality into those machines with all of the choices we’re making about what counts and what doesn’t count.
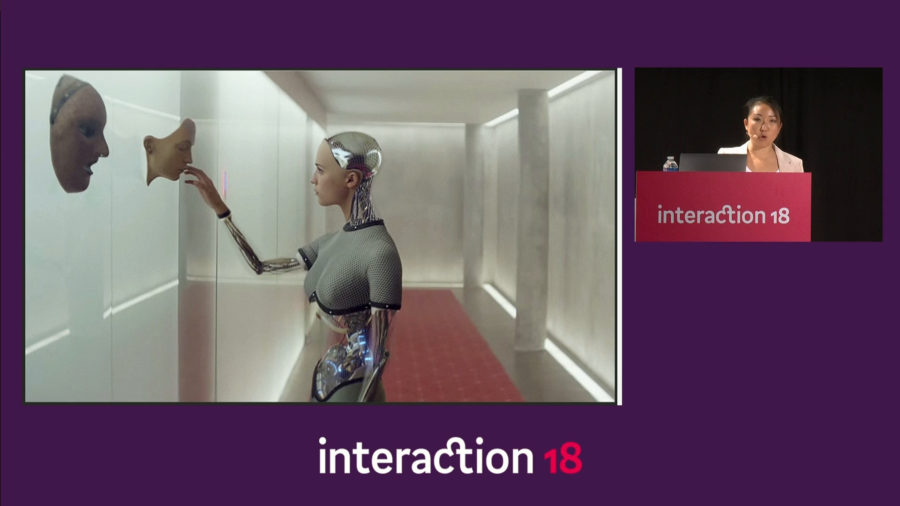
BJ Copeland states that a strong AI machine would be one, built in the form of a man; two, have the same sensory perception as a human; and three, go through the same education and learning processes as a human child. With these three attributes, similar to human development, the mind of the machine would be born as a child and will eventually mature as an adult.
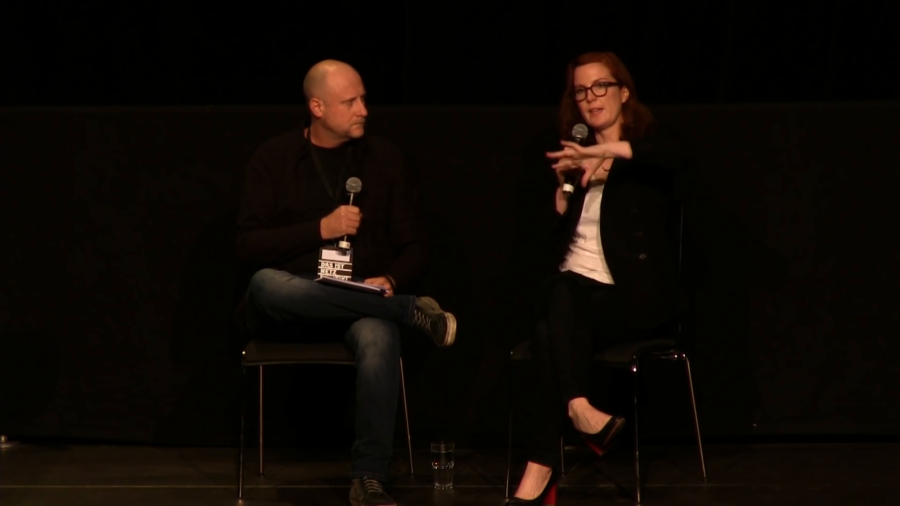
The big concerns that I have about artificial intelligence are really not about the Singularity, which frankly computer scientists say is…if it’s possible at all it’s hundreds of years away. I’m actually much more interested in the effects that we are seeing of AI now.
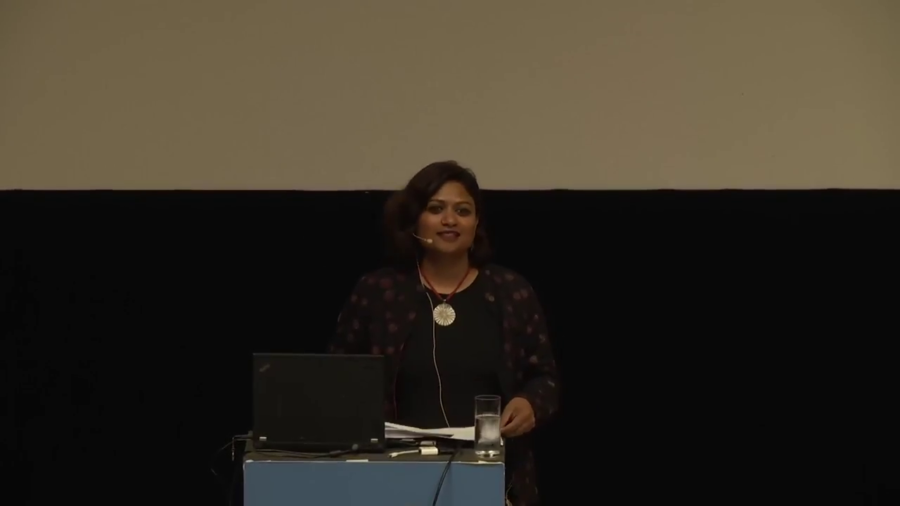
I’m interested in data and discrimination, in the things that have come to make us uniquely who we are, how we look, where we are from, our personal and demographic identities, what languages we speak. These things are effectively incomprehensible to machines. What is generally celebrated as human diversity and experience is transformed by machine reading into something absurd, something that marks us as different.
One of the most important insights that I’ve gotten in working with biologists and ecologists is that today it’s actually not really known on a scientific basis how well different conservation interventions will work. And it’s because we just don’t have a lot of data.