AI Blindspot is a discovery process for spotting unconscious biases and structural inequalities in AI systems.
Archive (Page 1 of 2)
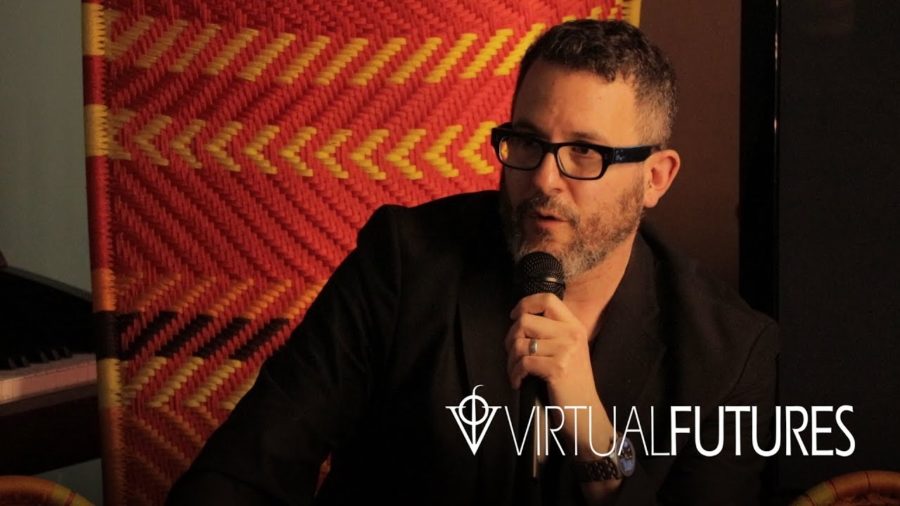
I am profoundly envious of people who get to write about settled domains or sort of settled states of affairs in human events. For me, I was dealing with a set of technologies which are either recently emerged or still in the process of emerging. And so it was a continual Red Queen’s race to keep up with these things as they announce themselves to us and try and wrap my head around them, understand what it was that they were proposing, understand what their effects were when deployed in the world.
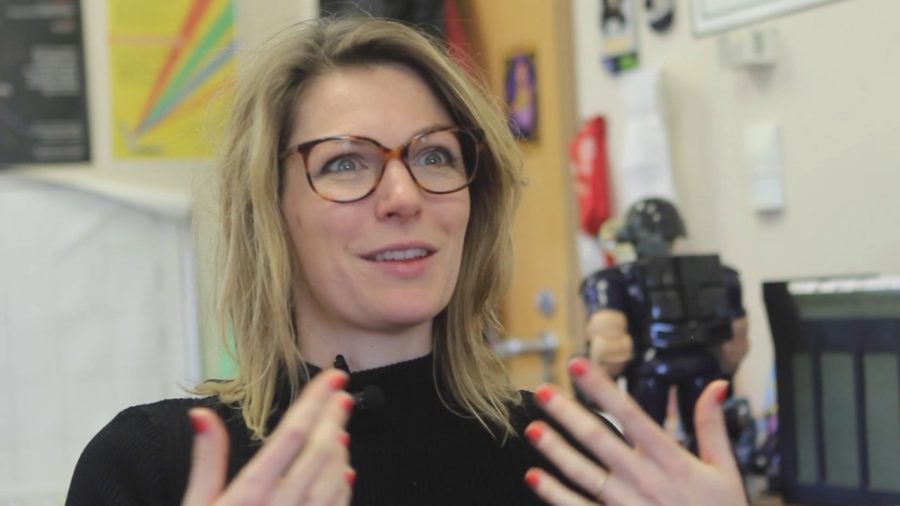
Increasingly we’re using automated technology in ways that kind of support humans in what they’re doing rather than just having algorithms work on their own, because they’re not smart enough to do that yet or deal with unexpected situations.
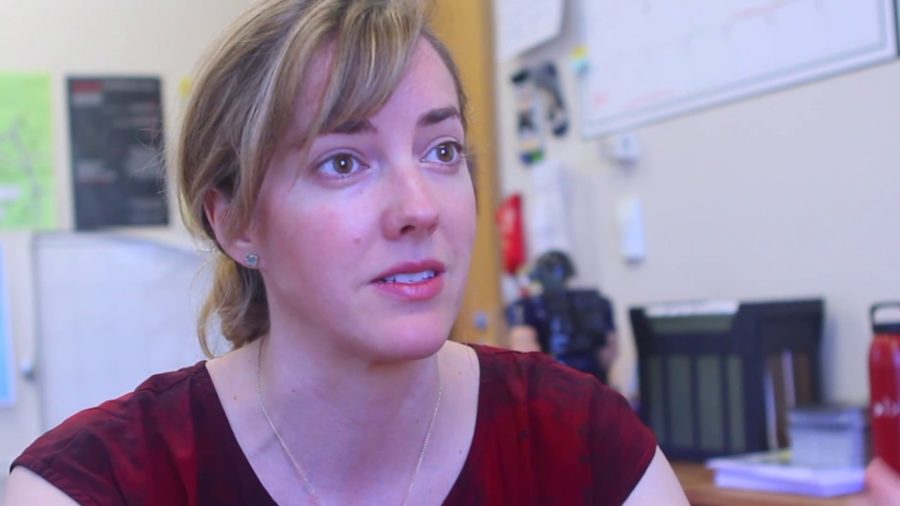
I teach my students that design is ongoing risky decision-making. And what I mean by ongoing is that you never really get to stop questioning the assumptions that you’re making and that are underlying what it is that you’re creating—those fundamental premises.
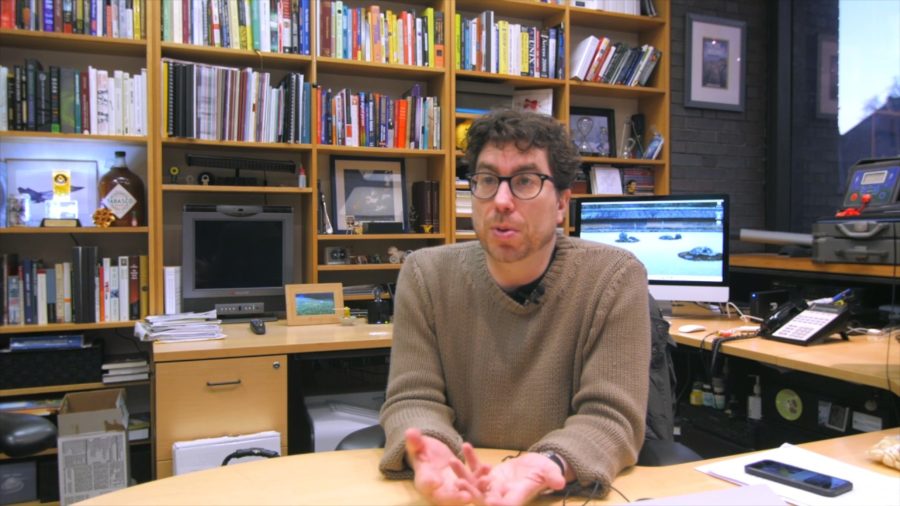
If you have a system that can worry about stuff that you don’t have to worry about anymore, you can turn your attention to other possibly more interesting or important issues.
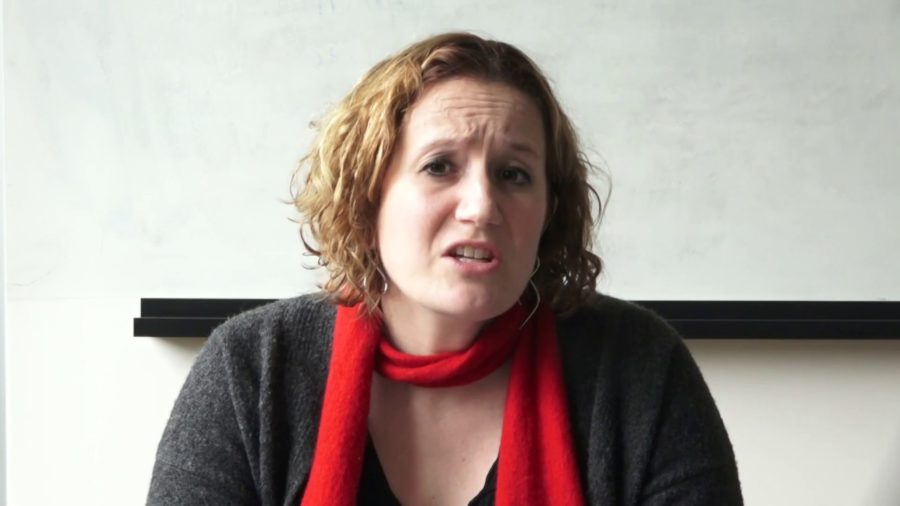
One of the challenges of building new technologies is that we often want them to solve things that have been very socially difficult to solve. Things that we don’t have answers to, problems that we don’t know how we would best go about it in a socially responsible way.
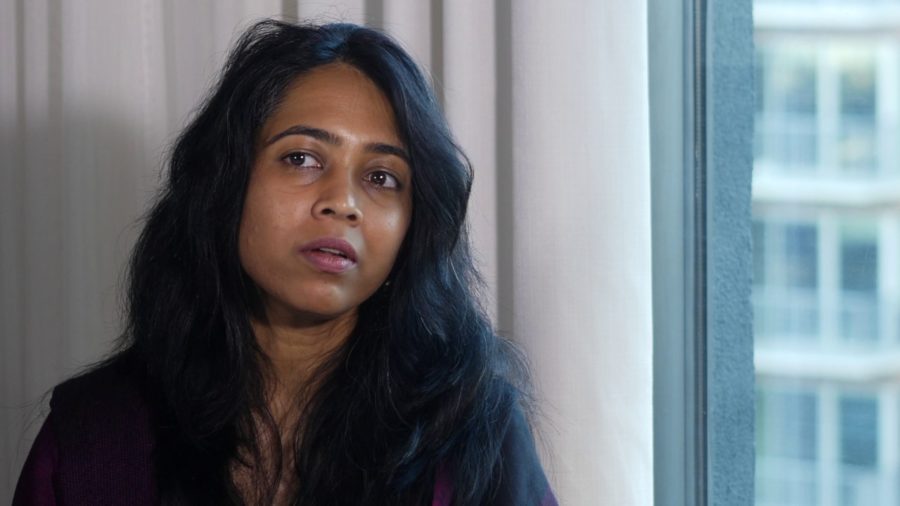
In a world of conflicting values, it’s going to be difficult to develop values for AI that are not the lowest common denominator.
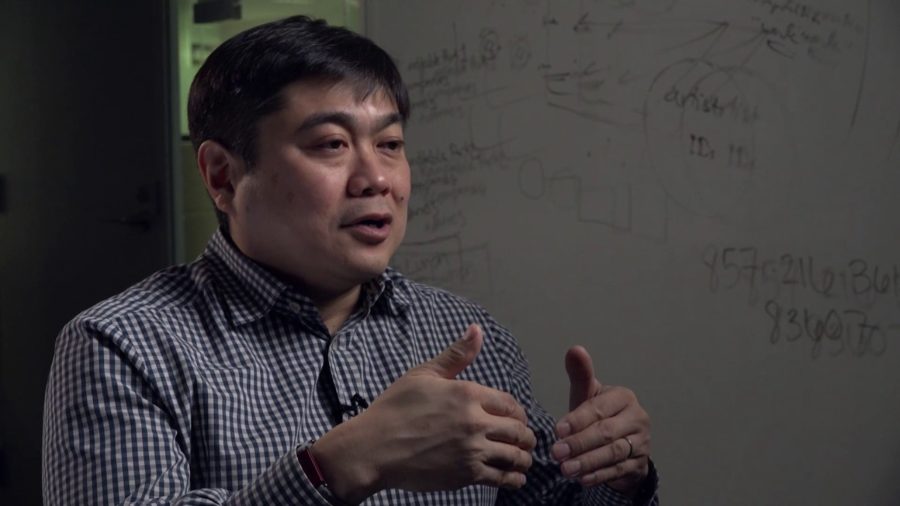
Machine learning systems that we have today have become so powerful and are being introduced into everything from self-driving cars, to predictive policing, to assisting judges, to producing your news feed on Facebook on what you ought to see. And they have a lot of societal impacts. But they’re very difficult to audit.