Maya Indira Ganesh: So, I’m going to just start with in the next twenty minutes telling you about a single-issue publication that I’ve been working on at Tactical Tech. It’s called See(n) Through Machines: Data Discrimination and Design. This publication will come out in a few weeks I hope, and has a number of essays in them. And for the purpose of this talk I’m going to talk about a couple of these essays—some contributions in the publication.
Please do me a favor; I am having some problems with my mother’s bank account, where you usually deposit your rent. From this month on, please transfer money to the following account: …
My landlord
I want to start with something that happened to me recently. I got into a little bit of miscommunication with my landlord. He sent me an email that said this. And it ended up in my spam folder. And as a result he was a little bit annoyed that I was sending the rent to the wrong account for many months.
Now, spam filters are the most common and familiar kinds of machine learning that we come across, but they still get it wrong. This email looked a lot like a phishing attempt, so it got sent to spam. Which is great. I mean, it made me feel very secure, but also annoying. It was also annoying because I missed this email.
Machine learning at its most sort of base level in a very simplified way, is a process of computer software reading a lot of data and identifying patterns and associations in that data, like how different pieces of information in that data set are related to each other. And we can move on to something a little bit more complex that relates to the sort of topic of my talk.
https://youtu.be/NesTWiKfpD0?t=1120
[Ganesh has some trouble getting the above clip to play at ~18:40; comments omitted.] Okay maybe we won’t do the video, which is a shame. But anyway this is a video by… It’s on YouTube, as you can see. So Dr. Michal Kosinski is a computational psychologist at Stanford University who develops deep neural networks, a kind of machine learning process that is said to closely mimic how animal brains are thought to work. They are used to identify patterns in faces based on images loaded online.
So, what Dr. Kosinski does is that he uses deep neural nets to identify extroverts and introverts. And in the clip he was talking about how you can look at people’s faces and identify their gender, how you can look at people’s faces and identify their political views. And he was saying that we get outraged when we think that machines can do these things, but if you think about it for a moment we do these things all the time. We’re constantly making these decisions about people based on when we see their faces, so why can’t we train machines to do the same thing?
So in that clip he was showing extroverted people and introverted people—their faces—and asking us in the audience, or his audience in that talk, to identify which one was the extrovert and which one was the introvert. People got it right. Machines also started getting it right because they could identify patterns in the way that extroverts and introverts were taking photographs of themselves. These were all selfies.
So that’s what the talk was about. And how machine learning works is that software called algorithms are exposed to a training data set, in this case faces of people. And the data can be really anything but it looks at patterns within those sets. So what Kosinski was looking at with the extrovert/introvert was tilt of the head, separation between the eyes, shape of the nose, breadth of the forehead. And then the algorithm was picking up those patterns in the faces and being able to identify them in new faces, so in a new data set that it had never seen.
This process of kind of looking at faces and identifying things about people is nothing new. I mean, we know that this is like over 150 years old. Trying to identify criminals, for example, it was a big thing in Europe about a hundred years ago.
Now, what’s interesting about Kosinski is that a few months ago he was in Berlin and he gave a talk where he described this research. He also talked about new research where he was applying facial recognition technology to gay and straight faces. He was working with a data set, he said, of self-identified gay and straight people who had posted their pictures online, as we all do, and using algorithms to read these faces and to be able to identify patterns in them—whatever patterns they saw. And then he said that with 90% accuracy, his algorithms then, based on what they had learned about the patterns in those faces… And we’re talking about like 35,000 images. That the same algorithm could look at a new data set and identify what was a “gay face” and what was a “straight face.”
And so he talked about this research. It’s not published yet. He was just sort of talking about it so I can’t actually give you a citation. And then, the next slide he showed us was a map of all the places in the world where homosexuality is criminalized and in some places punished by death. And he said, wouldn’t it be really unfortunate if this kind of technology was available to governments in these places. And I think he was concerned, but that did not make him sort of think about the technology he was developing.
Anyway, it’s also useful to note that Dr. Kosinski’s research on psychographics has been in the news for the last few months because allegedly Cambridge Analytica used his research to do microtargeting for election campaigns. So there’s kind of interesting connections there.
So let’s move on to another example. There’s an anecdote about border crossings between Canada and the US. This is from some time ago. The porosity of the border has made it possible for citizens of one country to travel, live in, and work in the other. So it was common for border control police to randomly ask people crossing to say the last four letters of the English language alphabet. Because it isn’t always easy in that part of the world to identify people based on just how they look or how they speak. Canadians, following the British English pronunciation, would say, “double-you, ex, why, zed” whereas Americans would say “double-you, ex, why, zee.” This quaint technique was of course very quickly learned and hacked. And of course after 9⁄11 passport checks became mandatory.
A version of this continues over in Europe. In a recent news story, Deutsche Welle reported that the German government was considering bringing in voice recognition technology to distinguish Syrian Arabic speakers from Arabic speakers from other countries, to ensure that only citizens fleeing Syria were receiving asylum in Germany. Deutsche Welle reports that such software is based on voice authentication technology used by banks and insurance companies.
However, the news report quotes linguistics experts who say that such analyses are fraught. Identifying the region of origin for anyone based on their speech is an extremely complex task, one that requires a linguist rather than automated software.
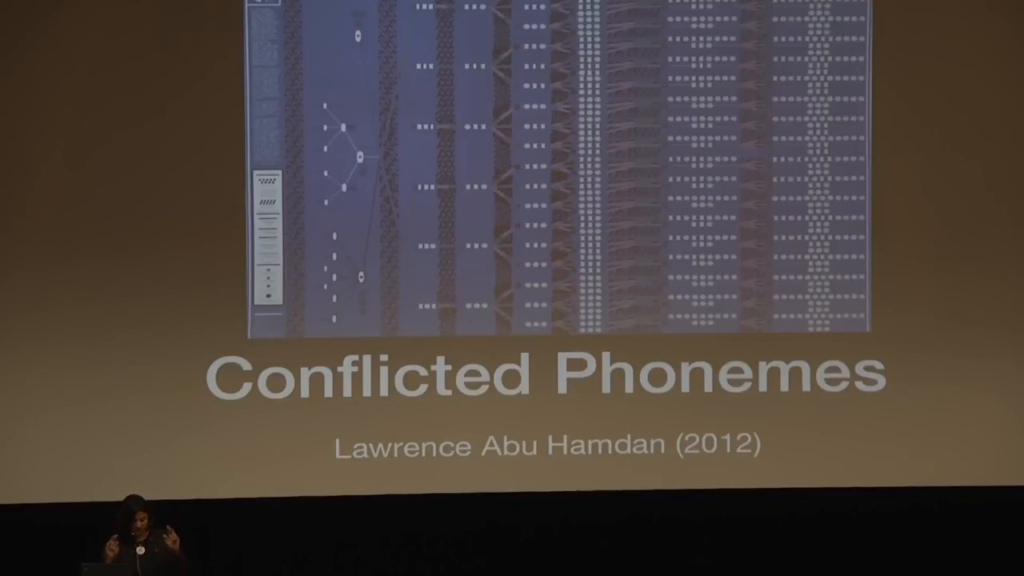
In 2012, the artist Lawrence Abu Hamdan held a meeting in Utrecht in the Netherlands to talk about the use of speech recognition technologies in asylum cases of Somali refugees. Having ascertained that they were coming from relatively safe pockets of Somalia, the Dutch authorities wanted to deny them asylum. Working with a group of cultural practitioners and artists and activists, and Somali asylum-seekers, Abu Hamdan was able to show that accent is not a passport, and was in fact a non-geographic map. Abu Hamdan writes that
The maps explore the hybrid nature of accent, complicating its relation to one’s place of birth by also considering the social conditions and cultural exchange of those living such itinerant lives. It reads the way people speak about the volatile history and geography of Somalia over the last forty years as a product of continual migration and crisis.
Lawrence Abu Hamdan, Conflicted Phonemes artist statement
Voice eventually is an inappropriate way to fix people in space.
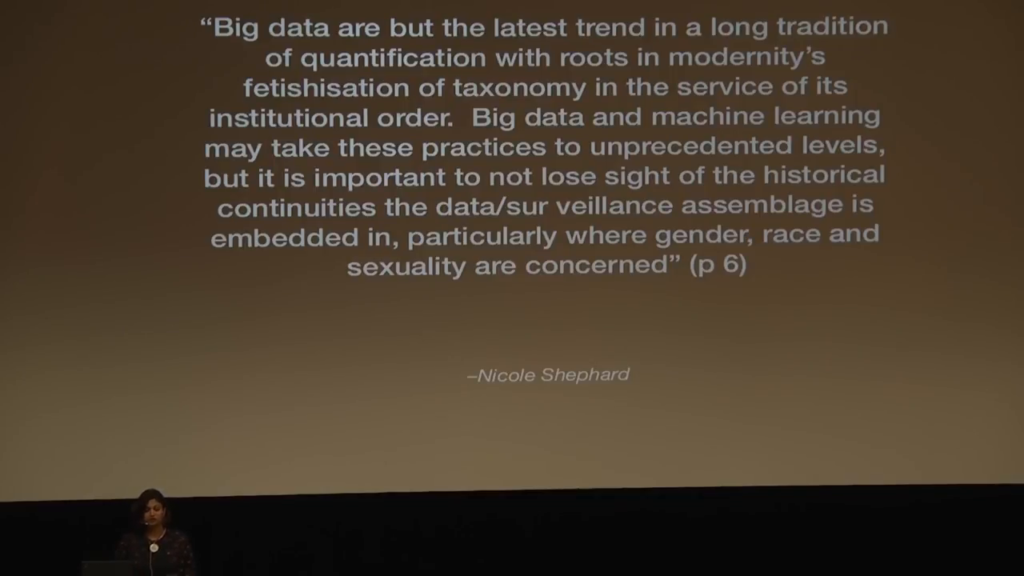
So, as Nicole Shephard writes, we must look at the practices of quantification and what they mean, but as a sort of continuation of history. “Big data are the latest trend in a long tradition of quantification with roots in modernity’s fetishization of taxonomy in the service of institutional order.” And what’s interesting about sort of looking—at least interesting for me in looking at big data technologies, is in a way that sort of challenges the assumption that all of these algorithms and machine learning and big data just kind of plug very seamlessly into human systems, and we can speed up and automate things. And as Shephard says, we need to look at the sort of historical continuities, especially where gender, race, and sexuality are concerned.
So I’m interested in data and discrimination, in the things that have come to make us uniquely who we are, how we look, where we are from, our personal and demographic identities, what languages we speak. These things are effectively incomprehensible to machines. What is generally celebrated as human diversity and experience is transformed by machine reading into something absurd, something that marks us as different.
Big data technologies are not only being used to classify but also misclassify us by what we say and do online. There’s a very interesting recent Pulitzer Prize-winning investigation by ProPublica—maybe many of you have seen this—that showed that racial bias was being perpetuated by the use of algorithms that predicted recidivism rates, or the likelihood of offending again, in that it was predicting that people of color were more likely to commit crimes in the future than white people.
What I found interesting about this story was that it exposed the ways in which state and private institutions, social prejudices, and multiple databases collude in the creation of algorithmic mechanisms with bias. Such networks effects have become the stuff of everyday news now, each story presenting a fresh outrage to established notions of human rights and dignity. For example, image recognition software that runs on machine learning identifies black people as gorillas or Asian people as blinking. Latonya Sweeney finds that people with black-sounding names were more likely to be served ads for services related to arrest and law enforcement. Interestingly I was in Canada recently, just at the the beginning of August for a few weeks for a summer school, and I found that as soon as I went to Canada I was getting these ads for arrest and “take care of your criminal record” and bail. And it was kind of shocking to me and I thought that okay, maybe in Canada people with Indian-sounding names seemed criminal.
So the sort of line of questioning that interests me in looking at data and discrimination is a continuation of Tactical Tech’s work from an exhibition we did last year called Nervous Systems: Quantified Life and the Social Question, which we did at the Haus der Kulturen der Welt between March and May last year. What Nervous Systems did was to try and tell a more layered and nuanced story beyond just how algorithms work, but to look at the complex infrastructures of quantification and software, but also cultural symbols, values, and practices.
One of the things that Nervous Systems did was to sort of unpack the historical precedent of big data, lest we think that these are things that are new. And I like this quote by Shannon Mattern that you know, this quantifying spirit is something that’s kind of old in Europe, but also in many other parts of the world:
Explorers were returning from distant lands with new bytes of information—logs, map, specimens—while back home Europeans turned natural history into a leisure pursuit. Hobbyists combed the fields for flowers to press and butterflies to pin. Scientists and philosophers sought rational modes of description, classification, analysis—in other words, systematicity.
Shannon Mattern, Cloud and Field, Places
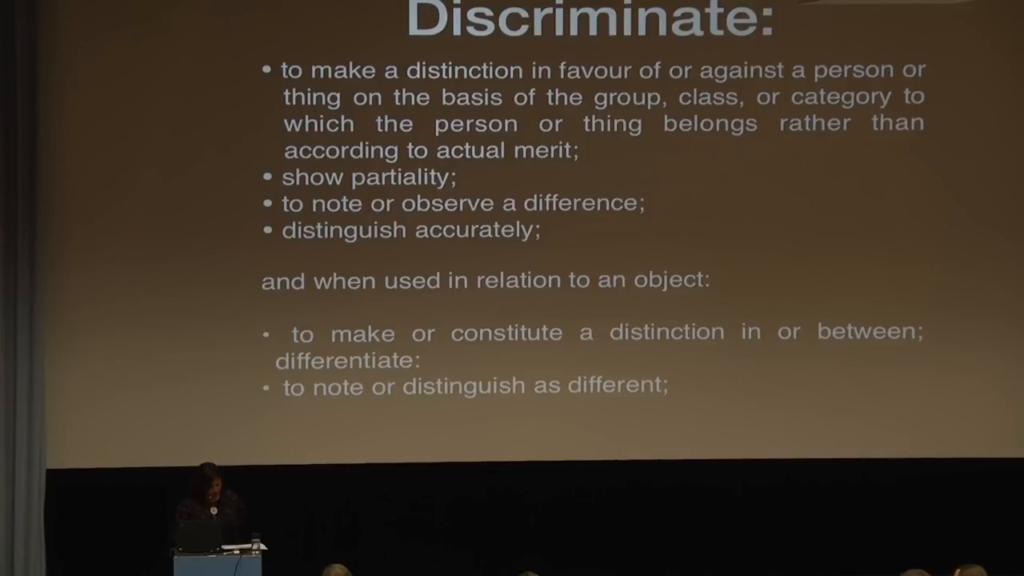
So I want to sort of go from this ordering and systematicity to the idea of discrimination itself. And so I start by sort of unpacking what does the word “discrimination” mean. And actually, you can move away from discrimination in a legal sense to sort of think that… The examples I’ve been talking about show that discrimination is about being very clearly identified, being very clearly distinguished and seen. It’s not necessarily just about disadvantage per se, but about visibility. And what does visibility through a machine mean for different kinds of people?
In work that I did last year at Tactical Tech with Jeff Deutch and Jennifer Schulte, we wrote about what we call the “tension between anonymity invisibility.” We examine the technology practices and environments of LGBT activists in Kenya, and of housing and land rights activists in South Africa. We found that they wanted the kinds of visibility that technology brought, but in order to do their activism and make their claims, that visibility brought risks because of their marginal position in society. Being gay and from a working-class background or a very Christian environment, or from a small town, or some combination of these, was much more risky, perhaps, for someone who is maybe English-speaking, upper class, urban. Both ran the risk of exposure through technology and had to maintain their presence online very carefully. But the latter’s visibility was less of a liability.
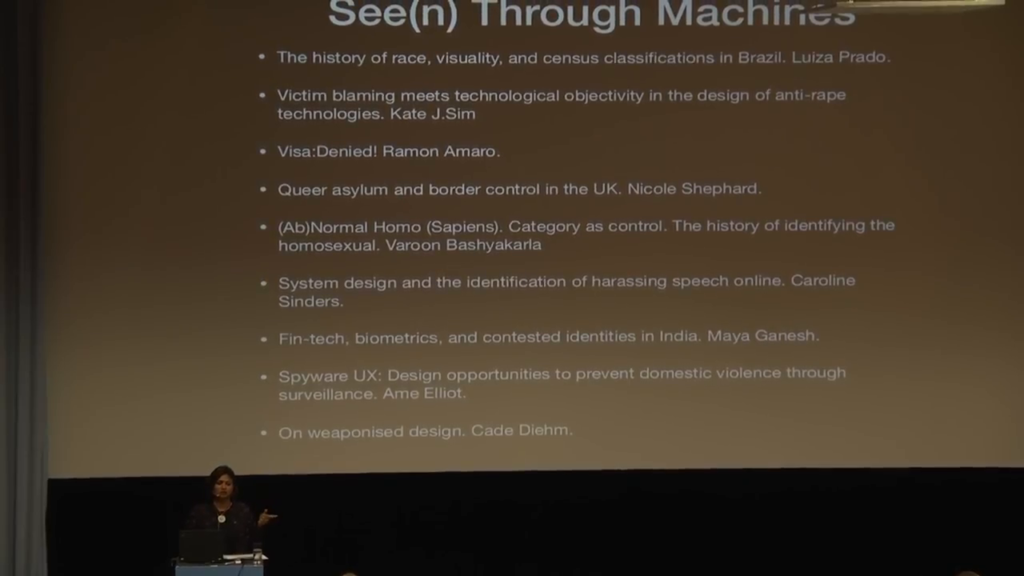
So for the past four months I’ve been working on this zine, or magazine, about data and discrimination which I call “See(n) Through Machines” to sort of examine what it means to be seen through machines, but also can we look through the machine in the process of doing so. These are some of the contributions that are there, and I’m going to take you quickly through them.
The first one is actually really interesting because Luiza Prado is a designer who’s looking at this artifact called the Humboldt Cup. It’s actually on display at the me Collectors Room in Auguststrasse in Mitte. The Humboldt Cup is a 17th century Dutch artifact. And what she does is she traces this cup, which has engravings of Brazilian natives, and through that explores how racial classification and the census in Brazil developed.
And since I’m running out of time I’m going to leave that and talk about the last D, actually, which is design. So that there’s data, discrimination, and design. I’ve been really interested in sort of struggling with the idea of design and what we expect of design in this context. If we design something better, we think maybe the problem will go away. Or that if we had more visibility and transparency in the design process, systems will be improved and held to account.
One contribution in the zine that talks directly to this is Kate Sim’s contribution on anti-rape technologies. She says that anti-rape technologies are about good user experience—UX—as well as about gathering more data about the context in which rapes happen, in order to design better systems. She finds that the design process is dislocated, and anti-rape technology is actually developed in many different cities, and because this process is dislocated makes accountability difficult.
Ame Elliott and Cade Diehm directly take on UX design and the weaponization of design and malware, saying that just making something easier to use, even if it’s malware, isn’t necessarily a good thing. There’s a lot of emphasis on marketing good design to make systems more usable.
Caroline Sinders writes to designers and technical experts to consider that perhaps machines and machine learning are as messy as the people who make them. In showing how complex it is for machines to learn, how language works, she discusses developments in automating online harassment and abuse identification in Wikipedia.
At some level, talking about machines and bodies and data and discrimination is really also a study in failure and error, in machine systems but also in our own human imagination and empathy for and about each other. So I will end on that note and ask that you look out for our new publication. It should be out by the end of September. Stay in touch, and I’m happy to take more questions as we go forward. Thanks.